In recent years, the venture capital (VC) industry has witnessed a seismic shift as artificial intelligence (AI) and machine learning (ML) technologies transform traditional investment practices. As we enter 2024, the adoption of these cutting-edge tools is no longer a mere trend but a necessity for VCs looking to maintain a competitive edge in an increasingly data-driven landscape.
The Current State of AI Adoption in Venture Capital
The integration of AI and ML in venture capital has been gaining momentum, with industry leaders recognizing the potential of these technologies to revolutionize every aspect of the investment process. According to a recent Gartner report, while only 5% of VCs were leveraging AI for decision-making in 2021, this figure is projected to skyrocket to 75% by 2025.
Early adopters of AI in venture capital, such as SignalFire, EQT Ventures, and InReach Ventures, have paved the way for a new era of data-driven investing. These pioneering firms have developed proprietary AI-powered platforms that are reshaping how deals are sourced, evaluated, and managed.
However, the road to widespread AI adoption in VC is not without its challenges. Many firms face hurdles such as:
Data quality and availability issues
Integration with existing processes
Balancing AI insights with human judgment
Ethical considerations and potential biases
Despite these obstacles, the potential benefits of AI in venture capital are too significant to ignore. Let's explore the key applications that are driving this technological revolution in the VC industry.
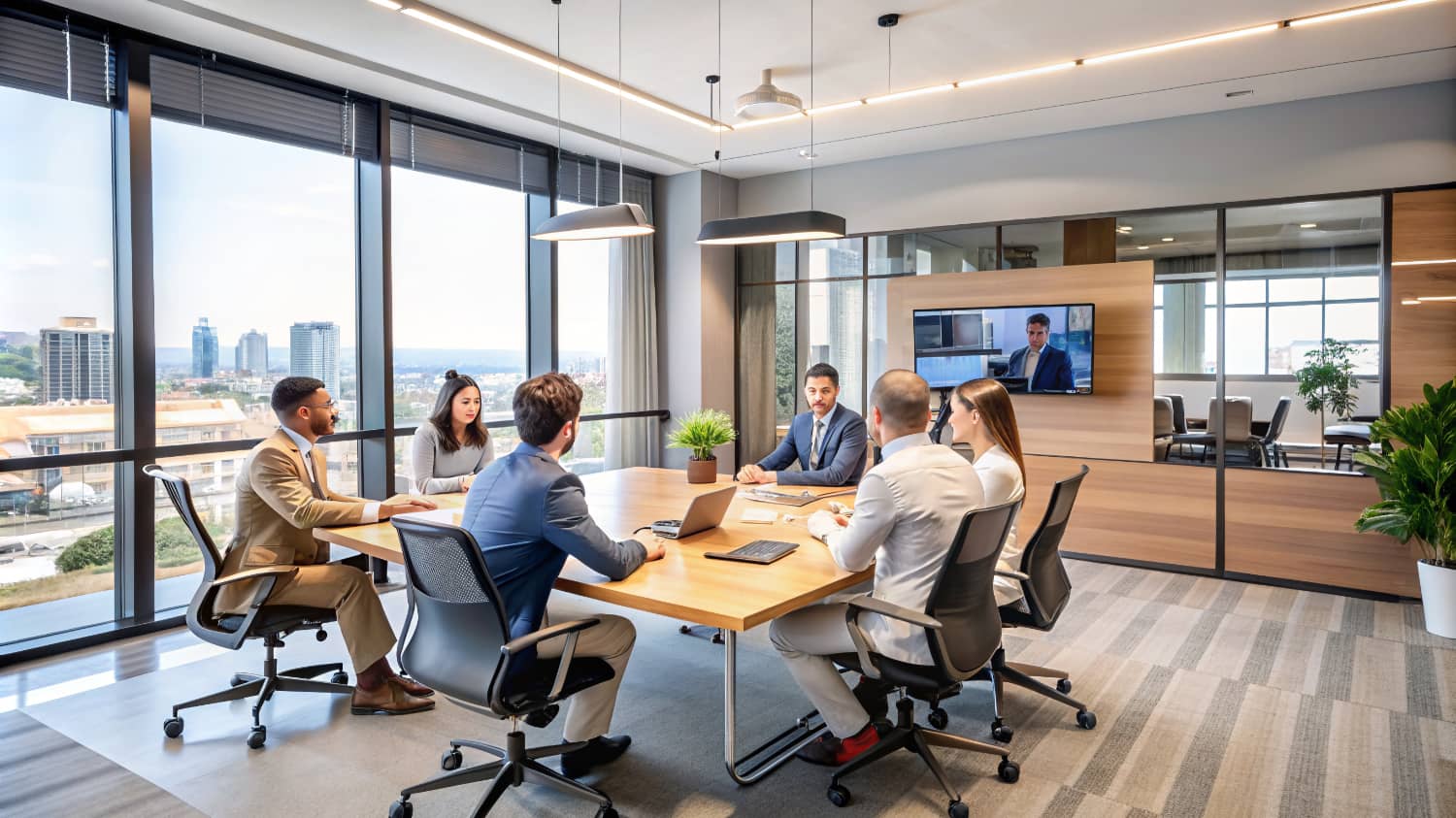
Key Applications of AI and Machine Learning in Venture Capital
1. Deal Sourcing and Screening
One of the most impactful applications of AI in venture capital is in the realm of deal sourcing and screening. AI-powered platforms are enabling VCs to cast a wider net and identify promising startups with unprecedented efficiency.
For example, EQT Ventures' Motherbrain platform uses machine learning algorithms to analyze vast amounts of data from multiple sources, helping the firm discover high-potential companies before they hit the radar of traditional VC networks. Similarly, InReach Ventures' proprietary AI system sifts through millions of data points to uncover promising early-stage European startups.
These AI-driven deal sourcing tools offer several advantages:
Automated deal flow management: AI systems can process and categorize thousands of potential deals, freeing up valuable time for VC professionals.
Pattern recognition: Machine learning algorithms can identify subtle patterns and trends that human analysts might overlook, potentially uncovering hidden gems.
Reduced bias: By relying on data-driven insights, AI can help mitigate some of the unconscious biases that may influence human decision-making.
2. Investment Decision-Making
AI is also making significant inroads in the investment decision-making process. Machine learning models are being employed to evaluate startups, assess risk, and predict potential returns with greater accuracy than ever before.
AI Application | Description | Benefits |
Startup Evaluation | ML models analyze various data points to assess a startup's potential | More objective and comprehensive evaluation |
Risk Assessment | AI algorithms calculate risk factors based on historical data and market trends | Improved risk management and portfolio diversification |
Return Prediction | Predictive analytics forecast potential returns on investments | Data-driven investment decisions |
These AI-powered tools are not meant to replace human judgment but rather to augment it. By providing data-driven insights, they enable VC professionals to make more informed decisions and allocate their time and resources more effectively.
3. Due Diligence and Market Analysis
The due diligence process, traditionally a time-consuming and labor-intensive task, is being streamlined through the application of AI and machine learning. Natural Language Processing (NLP) algorithms can rapidly analyze vast amounts of unstructured data, including news articles, social media posts, and company documents, to gather insights about a potential investment.
AI-powered market analysis tools are also helping VCs gain a deeper understanding of competitive landscapes and industry trends. These systems can:
Map out competitive landscapes with greater detail and accuracy
Identify emerging market trends before they become mainstream
Provide real-time updates on industry developments
By leveraging these AI capabilities, VCs can conduct more thorough due diligence in less time, potentially uncovering critical information that might have been missed through traditional methods.
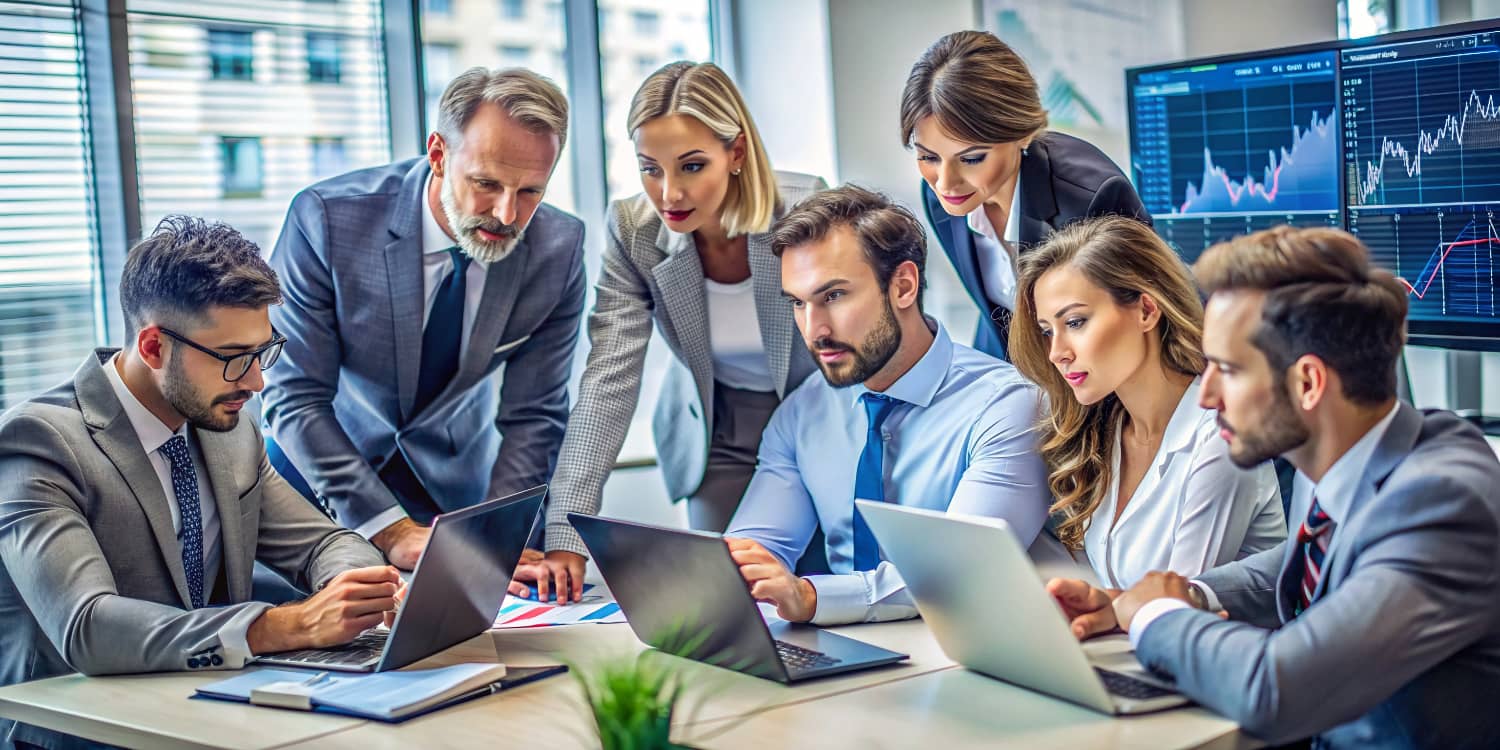
Portfolio Management and Monitoring
As AI continues to transform the venture capital landscape, its impact extends well beyond the initial investment decision. Portfolio management and monitoring have become increasingly data-driven, allowing VCs to track and optimize their investments with unprecedented precision.
AI-Driven Performance Tracking
Modern AI systems can continuously monitor a wide array of metrics for portfolio companies, providing real-time insights into their performance. These platforms aggregate data from multiple sources, including:
Financial reports
Web traffic analytics
Social media sentiment
Employee growth rates
Product adoption metrics
By analyzing this data, AI algorithms can detect early signs of success or potential issues, allowing VCs to respond proactively. For instance, Hatcher+'s VAAST platform uses machine learning to score companies based on various performance indicators, enabling investors to quickly identify which startups in their portfolio require attention or support.
Predictive Analytics for Startup Growth
One of the most exciting applications of AI in portfolio management is the use of predictive analytics to forecast startup growth trajectories. These models consider historical data, market trends, and company-specific factors to project future performance.
For example, an AI system might predict:
Revenue growth over the next 12 months
Likelihood of securing the next funding round
Potential for market expansion or product diversification
Armed with these insights, VCs can make more informed decisions about follow-on investments, resource allocation, and exit strategies.
Automated Reporting and Insights Generation
AI is also streamlining the reporting process for VC firms. Natural Language Generation (NLG) technologies can automatically create detailed reports on portfolio performance, market trends, and investment opportunities. These AI-generated reports can save significant time and resources while providing consistent, data-driven insights to both internal teams and limited partners.
AI Technologies Powering VC Applications
The AI revolution in venture capital is driven by several key technologies, each playing a crucial role in different aspects of the investment process:
Natural Language Processing (NLP): NLP allows AI systems to understand and analyze human language, enabling the processing of vast amounts of unstructured data from sources like news articles, social media, and company documents.
Machine Learning Algorithms: These form the backbone of many AI applications in VC, from deal sourcing to risk assessment. Popular techniques include:
Random forests for classification tasks
Support Vector Machines for pattern recognition
Gradient Boosting for predictive modeling
Deep Learning and Neural Networks: These advanced AI techniques are particularly useful for complex tasks like image recognition (e.g., analyzing startup pitch decks) and time series forecasting (e.g., predicting startup growth trajectories).
Computer Vision: This technology enables AI systems to interpret visual information, which can be valuable for analyzing charts, graphs, and other visual data in startup presentations and reports.
Case Studies: AI Implementation in Leading VC Firms
To better understand how AI is being applied in practice, let's examine a few notable examples:
SignalFire's Beacon Talent
SignalFire, a data-driven VC firm, has developed Beacon Talent, an AI-powered recruiting platform that tracks millions of potential startup employees. This tool not only helps portfolio companies hire top talent but also serves as a valuable source of data for investment decisions.
EQT Ventures' Motherbrain
Motherbrain, EQT Ventures' proprietary AI platform, analyzes data from multiple sources to identify promising investment opportunities. The system has been credited with sourcing several successful investments, demonstrating the power of AI in deal discovery.
InReach Ventures' AI Investment Platform
InReach Ventures has built a comprehensive AI system that covers the entire investment process, from deal sourcing to due diligence. Their platform processes data from over 200 sources, enabling the firm to efficiently discover and evaluate early-stage European startups.
Hatcher+'s VAAST Platform
Hatcher+ has developed the Venture as a Service Technology (VAAST) platform, which uses AI to analyze and score potential investments. The system considers over 500 data points for each company, providing a data-driven approach to deal evaluation and portfolio management.
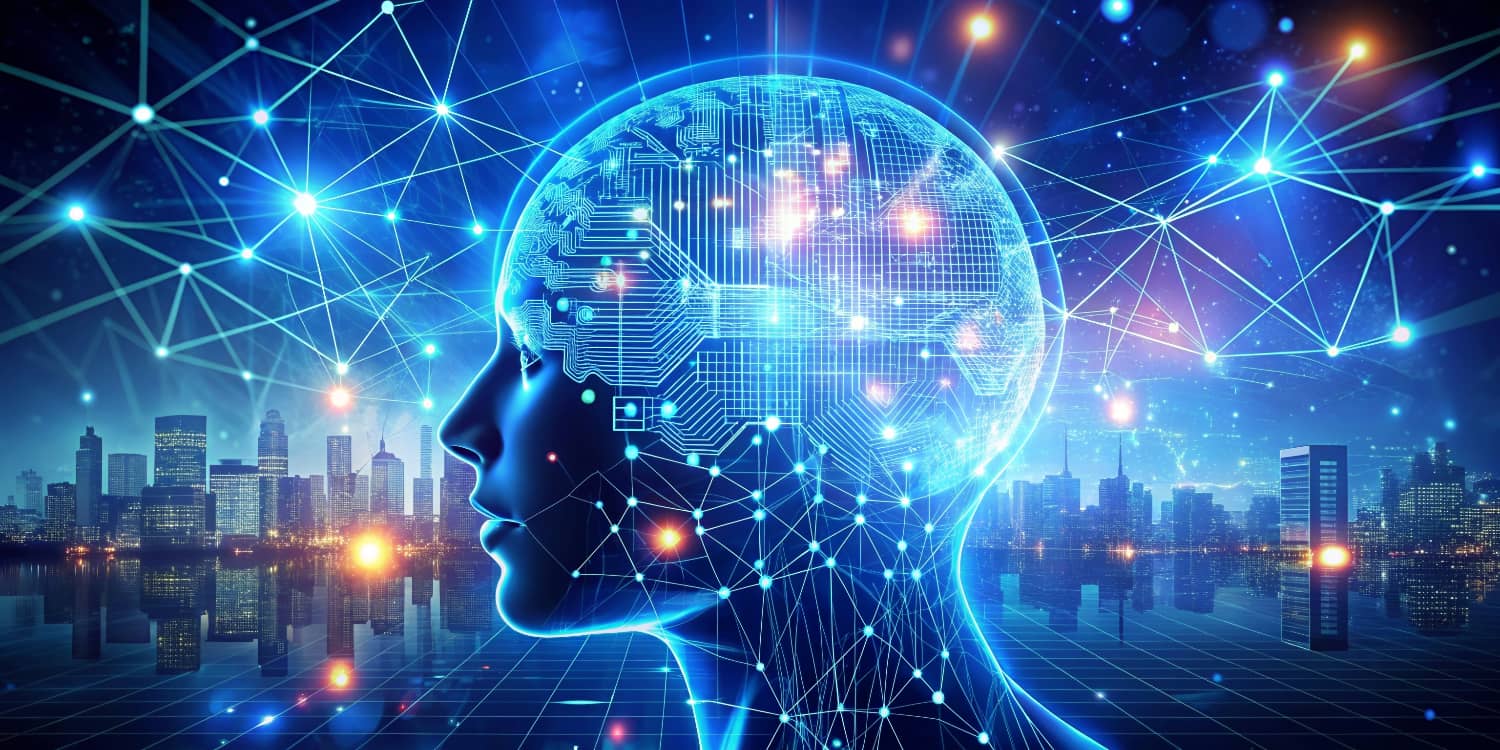
Benefits of AI and Machine Learning in Venture Capital
The integration of AI and machine learning into venture capital operations offers a myriad of advantages that are reshaping the industry. Let's delve into the key benefits:
Improved Operational Efficiency
One of the most immediate and tangible benefits of AI in VC is the dramatic increase in operational efficiency. AI-powered systems can:
Process vast amounts of data in seconds
Automate repetitive tasks
Streamline workflows
For instance, Pilot Growth Equity's NavPod platform automates up to 90% of deal sourcing activities, allowing the firm to focus more on high-value tasks like relationship building and strategic decision-making.
Enhanced Deal Discovery and Evaluation
AI significantly expands a VC's ability to discover and evaluate potential investments. Traditional methods often rely heavily on personal networks and manual research, which can be limiting. In contrast, AI-driven platforms can:
Scan millions of companies globally
Identify promising startups before they hit mainstream radar
Provide data-driven insights for more objective evaluation
Redstone's AI system, for example, has helped the firm discover three companies in the last 12 months that they ultimately invested in, which might have been overlooked using traditional methods.
Data-Driven Decision Making
By leveraging machine learning algorithms and predictive analytics, VCs can make more informed investment decisions based on comprehensive data analysis. This approach:
Reduces reliance on gut feelings and personal biases
Provides quantitative backing for investment theses
Enables more accurate risk assessment and return predictions
InReach Ventures reports that their AI system helps remove human inefficiency in reviewing hundreds of companies daily, allowing investors to focus on pre-qualified opportunities.
Reduced Bias in Investment Processes
While not eliminating bias entirely, AI can help mitigate certain types of unconscious biases that may influence human decision-making. By focusing on data-driven metrics and patterns, AI systems can:
Highlight opportunities that might be overlooked due to personal biases
Provide a more diverse range of investment prospects
Potentially increase diversity in startup funding
Challenges and Limitations of AI in Venture Capital
Despite the numerous benefits, the adoption of AI in venture capital is not without its challenges:
Data Quality and Availability Issues
The effectiveness of AI systems is heavily dependent on the quality and quantity of data available. In the early-stage startup ecosystem, this can be particularly challenging due to:
Limited historical data for young companies
Inconsistent reporting standards across startups
Difficulty in obtaining reliable private company data
As Hatcher+ notes, it took them about a year and a half to collect and clean up data on approximately 600,000 VC transactions over 22 years to train their AI algorithms effectively.
Balancing AI Insights with Human Judgment
While AI can provide valuable insights, it's crucial to strike the right balance between algorithmic recommendations and human judgment. The venture capital industry still relies heavily on factors that are difficult to quantify, such as:
Founder charisma and resilience
Team dynamics
Market timing intuition
As one interviewee stated, "You need to eyeball the founder, you need to ask those valuable questions, you need to feel the connection."
Ethical Considerations and Potential Biases
AI systems can inadvertently perpetuate or even amplify existing biases if not carefully designed and monitored. VCs must be vigilant about:
Ensuring diverse training data
Regularly auditing AI systems for bias
Maintaining transparency in AI-driven decision processes
Integration Challenges with Existing VC Processes
Implementing AI systems into established VC workflows can be complex and may face resistance from team members accustomed to traditional methods. Successful integration requires:
Comprehensive training programs
Clear communication of AI's role and limitations
Gradual implementation to allow for adjustment and refinement
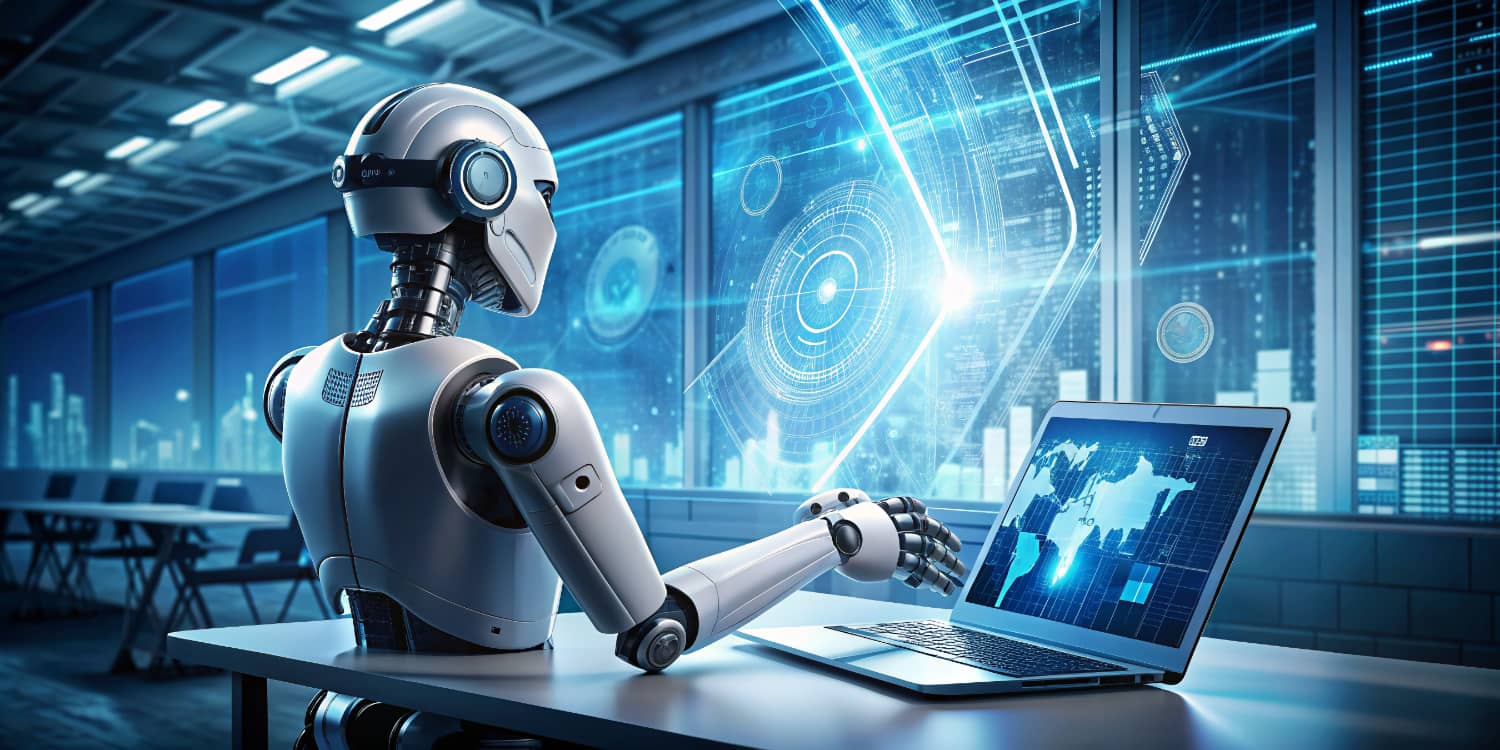
The Future of AI in Venture Capital
As we look ahead, the role of AI in venture capital is set to expand further. Emerging trends and potential new applications include:
Advanced Natural Language Processing: Improved ability to analyze startup pitches, customer feedback, and market sentiment.
AI-Driven Founder Matching: Platforms that connect startups with the most suitable investors based on comprehensive data analysis.
Predictive Exit Strategies: AI models that can forecast optimal exit timings and methods for portfolio companies.
Cross-border Investment Analysis: AI systems that can navigate complex international markets and regulations to identify global opportunities.
AI-Assisted Term Sheet Negotiation: Algorithms that can suggest optimal deal terms based on historical data and current market conditions.
As these technologies continue to evolve, the role of human VCs will likely shift towards higher-level strategy, relationship building, and providing value-add services to portfolio companies. The most successful VCs of the future will be those who can effectively leverage AI tools while maintaining the human touch that is crucial in the startup ecosystem.
Best Practices for Implementing AI in VC Firms
As the adoption of AI in venture capital accelerates, it's crucial for firms to approach implementation strategically. Here are some best practices for VCs looking to leverage AI effectively:
1. Develop a Comprehensive AI Strategy
Before diving into AI implementation, VCs should:
Define clear objectives: Identify specific areas where AI can add the most value to your firm's operations.
Assess readiness: Evaluate your firm's current data infrastructure and technical capabilities.
Create a roadmap: Outline a phased approach for AI adoption, starting with high-impact, low-complexity applications.
2. Build or Acquire AI Capabilities
VCs have two main options for acquiring AI capabilities:
In-house development:
Pros: Customized solutions, proprietary advantage
Cons: Requires significant investment in talent and resources
Third-party solutions:
Pros: Faster implementation, lower initial costs
Cons: Less customization, potential dependency on external providers
Many successful AI-driven VCs, like Redstone and InReach Ventures, have opted for in-house development to maintain a competitive edge. However, smaller firms might find third-party solutions more accessible as a starting point.
3. Ensure Data Quality and Ethical Use of AI
The foundation of effective AI is high-quality data. VCs should:
Implement robust data collection and cleansing processes
Regularly audit data sources for accuracy and relevance
Establish clear guidelines for ethical AI use, including bias mitigation and privacy protection
4. Train and Upskill VC Professionals
To maximize the benefits of AI, it's essential to:
Provide comprehensive training on AI tools and their applications
Foster a data-driven culture within the firm
Encourage continuous learning and adaptation to new AI technologies
5. Maintain a Balance Between AI and Human Insight
While AI can significantly enhance decision-making, it's crucial to:
Use AI as a tool to augment human judgment, not replace it
Encourage critical thinking and questioning of AI-generated insights
Recognize the importance of qualitative factors that AI may not capture
Conclusion
The integration of AI and machine learning in venture capital is no longer a futuristic conceptâit's a present reality that's reshaping the industry. From deal sourcing and due diligence to portfolio management and exit strategies, AI is enhancing every aspect of the VC lifecycle.
Key takeaways include:
AI-driven deal sourcing and screening are dramatically expanding the pool of potential investments.
Machine learning algorithms are providing data-backed insights for more informed investment decisions.
AI-powered portfolio management tools are enabling more proactive and effective support for startups.
The most successful VCs will be those who can effectively blend AI capabilities with human expertise and relationship-building skills.
As we move forward, the question for VCs is no longer whether to adopt AI, but how to implement it most effectively. Those who embrace this technology thoughtfully and strategically will be well-positioned to thrive in the increasingly competitive and data-driven world of venture capital.
The future of VC is undoubtedly AI-augmented, but it will always require the unique human elements of vision, experience, and intuition that have long been the hallmarks of successful venture investing.