Poor customer service can have devastating impacts on businesses. According to research, 89% of customers will leave brands after just a few bad experiences. With the meteoric rise of review sites and social media, negative customer feedback can spread like wildfire and severely damage company reputation. In this ultracompetitive landscape, delivering outstanding service is imperative for attracting and retaining customers.
This is where customer service analytics (CSA) comes in. CSA utilizes sophisticated data analysis techniques to transform raw customer interactions into actionable insights. Unlike traditional metrics like CSAT and NPS which provide rearview snapshots, CSA adopts a forward-looking approach. Powerful analytics tools equipped with artificial intelligence (AI) and natural language processing (NLP) can process high volumes of multi-channel customer data to uncover hidden opportunities. The insights derived using CSA enable businesses to understand changing customer expectations, optimize processes, predict churn and ultimately craft exceptional customer experiences.
With CSA adoption expected to grow over 20% annually, leading companies are investing heavily in analytics to stay ahead of the curve. This article will walk you through everything you need to know to unlock CSA's immense potential for driving customer satisfaction and fueling business growth.
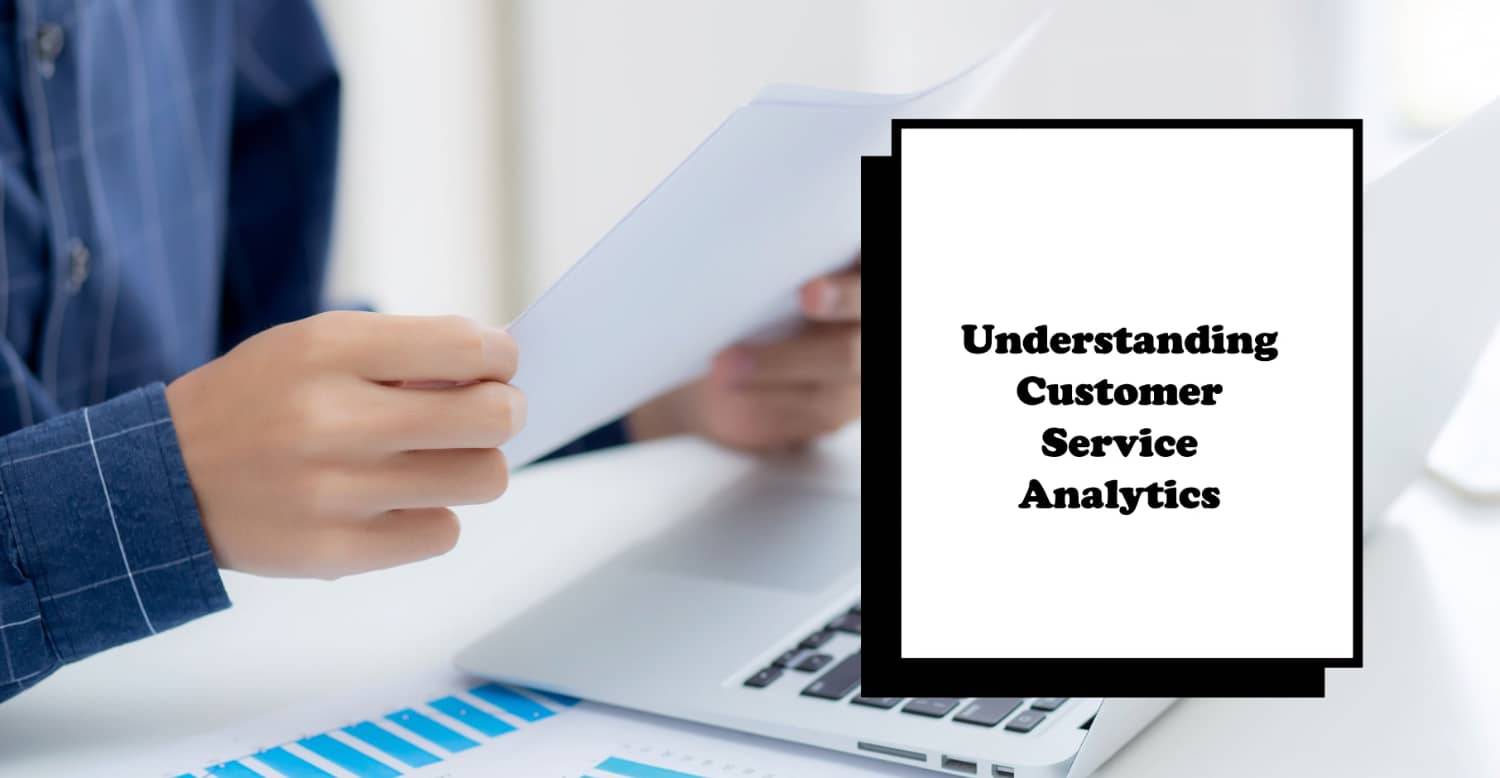
What is customer service analytics?
Customer service analytics (CSA) refers to the practice of collecting and analyzing data from customer interactions to derive meaningful insights. This includes both quantitative metrics as well as qualitative feedback across communication channels like phone, email, chat, social media and surveys.
While traditional key performance indicators like CSAT and NPS provide a snapshot, CSA adopts a more advanced approach. It leverages:
Descriptive analytics: To summarize what happened during customer interactions
Diagnostic analytics: To understand why specific events occurred
Predictive analytics: To anticipate future outcomes using AI algorithms
Prescriptive analytics: To receive AI-powered recommendations for driving optimal outcomes
Armed with these techniques, businesses can dive deeper into the voice of the customer. For instance, speech recognition and natural language processing extract key themes from unstructured conversations. Sentiment analysis detects emotions like frustration or delight. Linking this granular data with customer profiles further enriches visibility.
As per Gartner, the top five use cases for investing in CSA are:
Improving customer experience
Increasing customer lifetime value
Differentiating from the competition
Retaining customers
Attracting new customers
This underscores how CSA has evolved from a narrow operational tool to a key pillar of customer experience strategies. The insights unlocked using CSA empower various business functions to make data-backed decisions, align processes with evolving customer expectations and design hyper-personalized engagement.
Leveraging the Power of Customer Service Analytics
CSA derives insights by analyzing multi-channel customer service data from sources like:
Calls: Call logs, audio recordings, transcripts
Chat/Messaging: Conversation transcripts
Email: Ticket details and email content
Surveys: Responses and verbatims
Social media: Posts, comments and messages
This data is then processed to extract key metrics across three broad categories:
Customer Satisfaction Metrics
These indicate overall happiness of customers with the service experience.
CSAT: Customer satisfaction score
NPS: Net promoter score
CES: Customer effort score
Operational Efficiency Metrics
These metrics showcase the effectiveness of support operations.
FCR: First contact resolution rate
ART: Average response time
AHT: Average handling time
Customer Outcome Metrics
These highlight the business impact of customer service.
CLV: Customer lifetime value
Retention: Customer retention rate
Churn: Customer churn rate
Sentiment analysis is crucial for making sense of human conversations. Using NLP and ML, sentiment analysis detects emotions and categorizes them as positive, negative or neutral. These insights help understand satisfaction drivers.
By consolidating analytics across channels, CSA paints a comprehensive picture of the customer journey. Business can leverage these AI-powered insights to delight customers and accelerate growth.
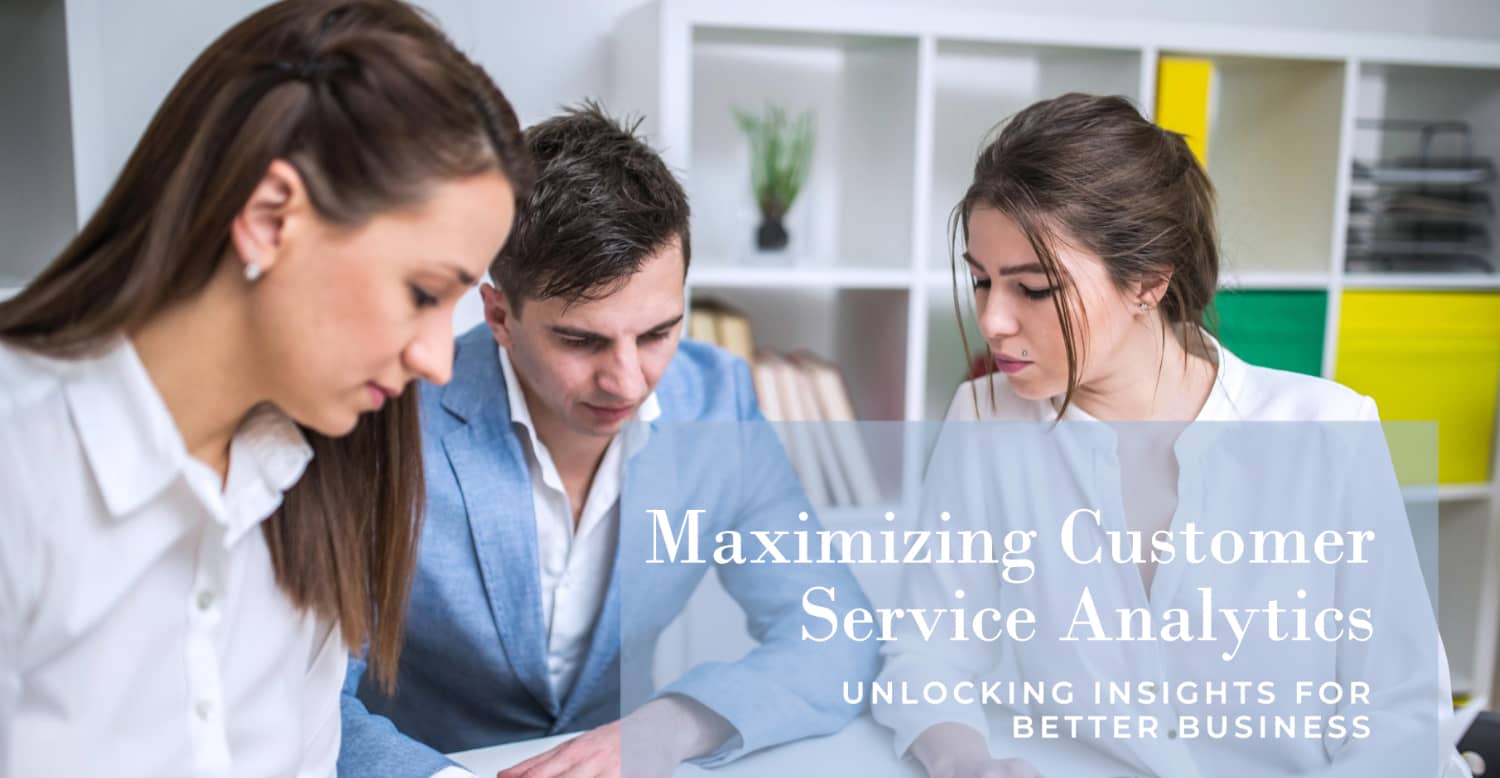
How can data analysis improve customer service?
CSA sheds light on areas of improvement that traditional metrics fail to highlight.
Enhancing Processes
Granular analysis of customer conversations reveals pain points in existing workflows. For example, diagnostic analytics can pinpoint the root causes behind repetitive issues. Addressing these through process changes or additional training drives efficiency.
Predicting and Preventing Churn
AI-based predictive models can identify customers likely to churn based on interaction patterns and sentiment signals. Proactively engaging these accounts using tailored incentives and offers retains them.
Optimizing Resource Allocation
Linking customer data with support traffic patterns facilitates better capacity planning. Descriptive analytics allows calibrating staff strength to manage fluctuations driven by external events. This balancing act boosts productivity and keeps costs in check.
In essence, CSA powers a outside-in approach where customer needs take center stage instead of internal KPIs. The AI-generated insights open your eyes to hidden weak spots while revealing new opportunities to deliver wow moments. This culture of continuous improvement is key for long-term success.
Implementing CSA for Business Success
Implementing a robust analytics practice entails a systematic approach across three phases:
1. Data Collection
This prerequisite step involves consolidating customer data from all touchpoints into a central repository. CRM systems like Zendesk and Salesforce provide out-of-the-box integration with diverse channels. A data lake stores structured and unstructured data.
2. Data Preparation
Raw data needs processing before analysis. Tasks include:
Cleansing: Fixing inaccuracies
Normalization: Formatting consistency
Enrichment: Augmenting data with external sources
Anonymization: Removing personal identifiers
3. Analysis and Visualization
Sophisticated analytics software leverages AI/ML algorithms to process prepared data and uncover insights. Visual data storytelling using dashboards and reports simplifies interpretation and drives actionability.
Ongoing monitoring through prescriptive analytics keeps KPIs on track. But the key is getting enterprise-wide buy-in and developing an analytics-focused culture. Appointing CX leadership and evangelists across functions stimulates adoption. With the right vision and plan, analytics success can become an organizational reality.
Tools and Technologies for Customer Service Analytics
A wide array of solutions exist in the marketplace to meet diverse customer service analytics needs. Here are some popular options:
SentiSum
SentiSum is an AI-powered analytics engine purpose-built for customer support conversations. Key capabilities include:
Multi-channel analysis: Email, chat, messaging, calls
Granular tagging
Sentiment analysis
Real-time insights
Intuitive dashboards
It seamlessly integrates with service management tools like Zendesk, Salesforce and Freshdesk.
Zendesk Explore
This analytics module is natively embedded within Zendesk Support for unified reporting. It offers:
Descriptive analytics
Custom reports/dashboards
Data exports
User activity audit
The tight integration simplifies analysis for Zendesk clients.
Service Cloud Einstein
The analytics offering from Salesforce applies AI models on Service Cloud data. Notable features:
Predictive lead scoring
Automated case classification
Next best action recommendations
It unlocks detailed insights to boost agent productivity.
The choice ultimately depends on analysis needs, willingness to adopt new tools and budget considerations. But the common thread is leveraging data to better serve customers.
Case Studies in Customer Service Analytics
The transformative impact of CSA is best highlighted through real-world examples.
Zoom
The video communications platform leverages AI and analytics across operations including customer support. By analyzing millions of support tickets with sentiment analysis and predictive modeling, Zoom has:
Lowered ticket resolution time by 23%
Cut customer complaints by 12% since 2019
Maintained high customer satisfaction
This exemplifies how CSA powers data-driven decisions to calibrate processes with customer expectations.
AirAsia
The low-cost airline employs diagnostic analytics to determine the root causes behind flight delays. By tracing interdependencies between functions, AirAsia identified that over 45% of delays were linked to aircraft turnaround processes. Addressing these through smarter scheduling and automation has:
Reduced average delays by 30%
Cut lost baggage by 21% on problematic routes
Improved Net Promoter Score
This showcases the systemic impact of CSA-driven insights on enhancing performance.
The transformations achieved by industry leaders highlight CSA's immense potential. Purposeful analytics strategies can catalyze similar success stories across the business landscape.
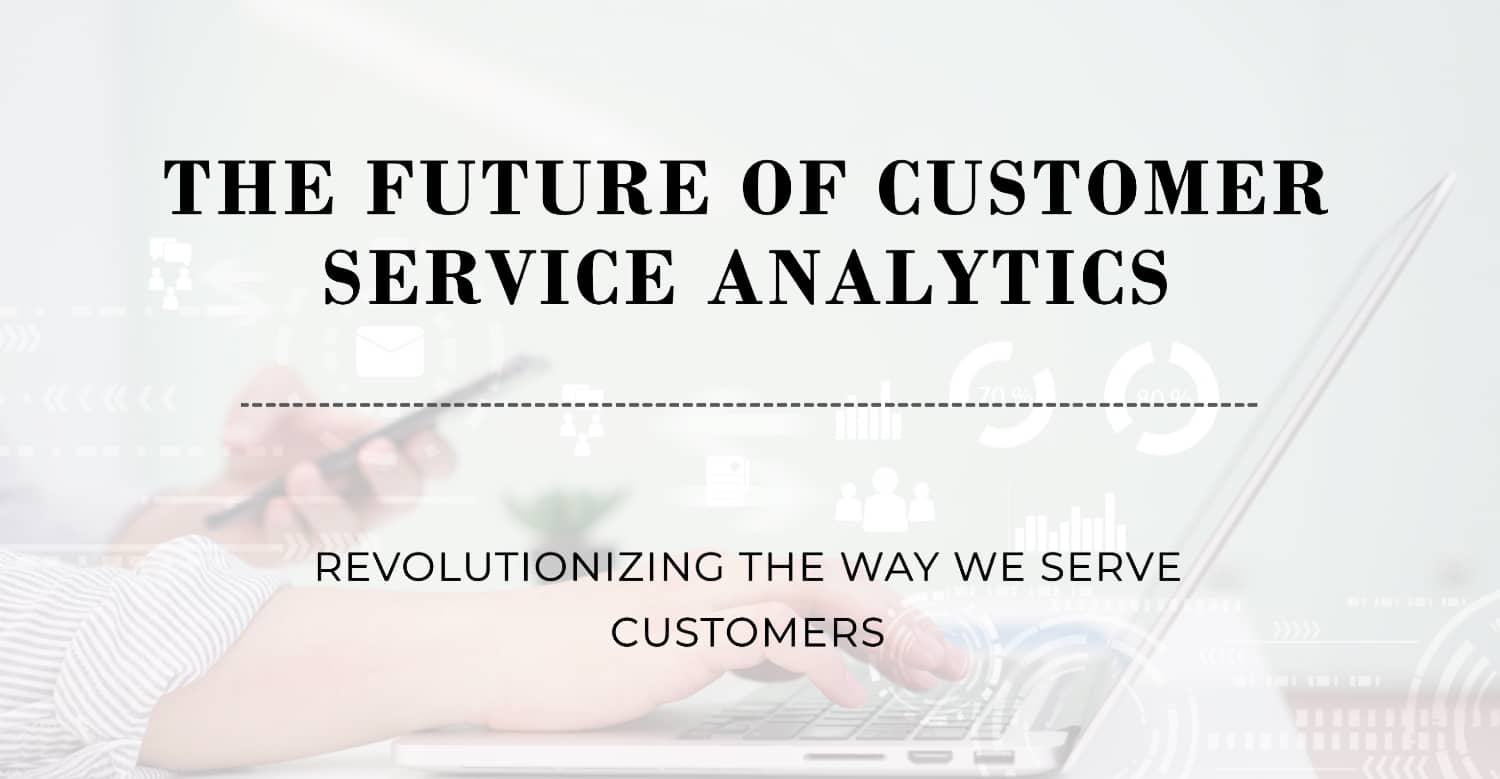
The Future of Customer Service Analytics
CSA is poised for massive innovation driven by emerging technologies.
AI and Machine Learning
Sophisticated AI and ML algorithms will catalyze a revolution in analytics. Capabilities like:
Automated speech recognition
Video/image analysis
Multi-lingual support
Hyper-personalization
Will become ubiquitous. Sentiment analysis will gain more granularity by detecting subtle emotions.
Real-Time Engagement
As models ingest data streams in real-time, the scope for prescriptive analytics will expand dramatically. Agents will be empowered to take context-aware actions dynamically during customer interactions.
Democratization
Self-service analytics tools will equip non-technical users across functions to conduct autonomous analysis without relying on data specialists. Augmented analytics systems will guide users, educate them and suggest insights.
Embedded Analytics
In future, analytics will become seamlessly woven into operational platforms instead of being adjacent solutions. For instance, CRMs will assimilate analytics capabilities for tighter insights-to-action orchestration.
CSA will be the vehicle for next-generation customer service that preempts needs, delights unconditionally and delivers incredible value.
Customer Service Analytics: The North Star for Exceptional Experiences
Customer service analytics illuminates the path to stellar customer experiences. By methodically capturing and examining high-fidelity customer data, businesses gain an information edge to excel on metrics that define success - satisfaction, loyalty and growth.
CSA transcends superficial sentiment metrics to unearth crucial insights around processes, predictions and prescription. Descriptive analytics investigates the what, diagnostic analytics explores the why while predictive analytics reveals the what's next. This expanding purview arms businesses with unmatched customer intelligence to sculpt positive journeys.
The future shines even brighter, with AI and automation enhancing analysis sophistication. As organizations embed analytics within systems and cultivate data-driven cultures, they manifest the capacity to understand, anticipate and serve customers exceptionally. Ultimately, CSA provides the beacon of truth that guides every function to make decisions the customer would love. When analytics becomes a way of corporate life, achieving customer centricity is no longer an aspiration but an outcome.