The age of artificial intelligence (AI) is upon us. Once a fledgling technology confined to research labs and science fiction, AI has now firmly entered the mainstream. An estimated 35% of businesses already leverage AI to enhance products, improve efficiency, and gain competitive advantage. However, for companies yet to embark on their AI journey, implementation can seem intimidating. How can you navigate the complex AI landscape and harness its power to drive business success?
This comprehensive guide aims to empower organizations and show them how to successfully implement AI into their business. We will demystify artificial intelligence, assess your readiness to adopt it, develop a robust AI strategy, choose the right implementation approach, integrate AI across operations, and ultimately, embrace continuous AI innovation. With the right framework in place, AI can help automate mundane tasks, uncover actionable insights, and take your organization into the future.
Leading technology consulting services and digital transformation partners highlight AI's incredible value. AI consultants can provide expertise during evaluation, recommendation, and deployment of enterprise-wide AI adoption. However, determining where to start and who to trust to steer your AI initiatives can be an obstacle. This guide offers best practices for AI implementation planning, illuminating key steps to integrate AI seamlessly. We will explore critical factors in selecting AI solutions and providers to mitigate risk and accelerate returns on your AI investments.
Equipped with an understanding of AI's potential, a clear roadmap to adoption, and insights from those pioneering this technology, your organization will gain confidence in unlocking AI's possibilities. By journey's end, you will have the knowledge to make AI a core competitive advantage.
Understanding the AI Landscape
Before diving into the details of AI implementation, it's important to level-set on what exactly artificial intelligence is and the landscape of AI applications.
What is Artificial Intelligence?
Artificial intelligence, or AI, refers to software and machines designed to perform tasks that normally require human intelligence. This includes skills like visual perception, speech recognition, decision-making, and language translation.
While AI may seem futuristic, in reality it is already integrated into many familiar products and services:
AI underpins popular personal assistants like Siri, Alexa and Google Assistant
AI powers the recommendations served by Netflix and Amazon
AI enables self-driving technology in cars
AI facilitates machine translations in Google Translate
Machine Learning Basics
A core component behind most AI is machine learning (ML). Machine learning involves "training" software algorithms with large sets of data, allowing the programs to learn from examples rather than needing explicit programming for every scenario.
There are three main types of machine learning:
Supervised learning: The algorithm is trained on well-labeled, structured data to map the relationship between inputs and desired outputs. It can then apply this learning to new unlabeled data to make predictions.
Unsupervised learning: The algorithm derives insights from datasets without pre-existing labels. Clustering patients into risk groups based on health factors is an example.
Reinforcement learning: The algorithm develops a model for how to maximize a reward through trial-and-error interactions with an environment. Game-playing AIs like AlphaGo use this approach extensively.
Beyond machine learning, there are also fields like natural language processing (NLP) focused on understanding human language, and computer vision centered on analysis of visual inputs like images and video.
Real-World AI Applications
Understanding these foundational areas allows companies to determine where their needs and AI capabilities align. Some of the common business use cases for AI implementation and machine learning include:
Chatbots & Virtual Assistants: AI-powered bots can provide 24/7 automated customer service, support internal teams, or act as an intelligent assistant for executives.
Predictive Analytics: Machine learning models can forecast future business trends and scenarios to aid planning around high-value initiatives.
Anomaly Detection: By analyzing large data sets, AI can pinpoint deviations, outliers, and anomalies that may signal security threats or fraud risks.
Computer Vision: AI can extract insights from visual content at massive scale, powering crucial applications in medical imaging or manufacturing quality control.
Entertainment and Gaming: AI is increasingly used to enhance user experiences in interactive platforms. For example, in gaming, advanced AI models power dynamic environments and personalized gameplay in popular titles like Sun Of Egypt 3, enriching the experience for players by adapting scenarios in real time.
As organizations explore how to implement AI capabilities, understanding these real-world applications provides a roadmap for aligning technology to business needs. Both the pace of advancement and variety of applications continue to expand rapidly, ensuring that companies can stay innovative and competitive.
Now that we've covered AI concepts at a high-level, we can dive deeper into assessing your organization's readiness and requirements.
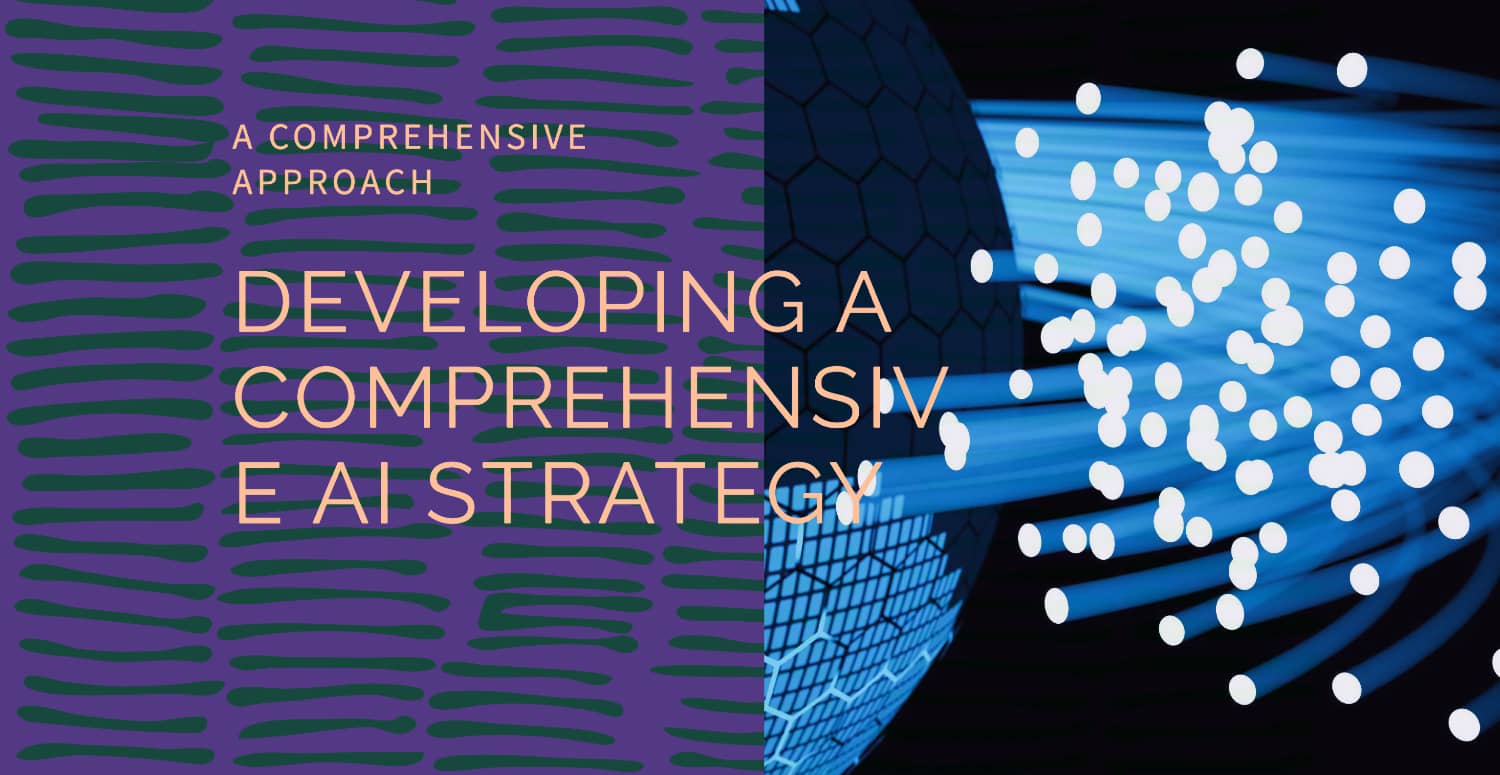
Developing a Comprehensive AI Strategy
With a data-driven understanding of the current state through AI readiness assessments, organizations can define a robust strategic plan to guide implementation.
Constructing an effective AI implementation strategy requires aligning on vision, governance, resourcing, and sequencing to ensure efforts stay targeted on business priorities rather than just chasing technology trends.
Establishing an AI Vision and Objectives
Just like any major business initiative, AI adoption must connect clearly to financial and operational objectives. Leadership teams should specify:
What pressing business challenges will AI be used to address?
What KPIs will define success over the next 1-3 years?
How will we benchmark progress of AI initiatives to demonstrate impact?
Informing stakeholders and aligning executive leaders around specific transformative use-cases is vital to driving urgency, investment, and AI implementation in your company.
AI Ethics and Governance
Since AI systems perform tasks previously requiring human discretion, establishing guidelines for ethical usage, transparency, and accountability is equally essential even early on. Aspects to address include:
What decisions or processes are not appropriate or too risky for full automation? Where is human oversight still essential?
How will bias be mitigated within training data or algorithms themselves?
How will AI usage rights, personal data privacy, security protocols and other policies be instituted across development and usage?
Who will be accountable for AI monitoring, retraining and model updates post-deployment?
Codifying AI principles and policies into governance frameworks embeds critical checks and balances as adoption expands.
Resourcing an AI Program
Depending on assessment findings around internal skills, organizations must secure the multidisciplinary talent needed for AI platform development and implementation, data engineering, machine learning model building, and more specialized roles. Budget must align to needs on:
Cloud infrastructure and data warehouses
Tools - notebooks, model repositories, MLOps pipelines
External staff augmentation
Relevant research libraries and pretrained foundations
A Phased AI Adoption Roadmap
Rather than attempting a broad rollout all at once, companies should pursue phased adoption to maximize learnings:
Phase | Focus | Timeline |
Proof of Concept | Demonstrate feasibility of AI for a focused business process using minimum viable data and models | 3-6 months |
Pilots | Deploy AI to start enhancing defined processes and users at small scale | 6-12 months |
Scaling Engineering | Industrialize pipelines, MLOps infrastructure for efficient model development | 12-18 months |
Domain Expansion | Grow AI coverage across additional business units | 18-24 months |
This sequencing balances short-term returns with long-term investments to systematically scale AI's footprint.
With the strategy and roadmap defined, deciding the right AI implementation process and methodology is the next key step.
Choosing the Right AI Implementation Method
Once the vision and strategic roadmap for implementing artificial intelligence have been aligned, the next decision point is what implementation approach makes the most sense for your organization. Essentially there are three routes to launching AI capabilities:
Build In-House
Leverage AI SaaS Solutions
Partner with AI Experts
The path taken depends heavily on several factors - level of internal skills, customization needed, and budgets.
Building AI In-House
Developing algorithms fully internally allows for maximum customization aligned to existing infrastructure. However it requires both specialized data science talent as well as modern machine learning platforms and stacks to be viable. Considerations include:
Data engineering and ML talent has to be budgeted for and developed internally long-term
Timelines for internal capability build-up can be lengthy before producing material outcomes
Requires research and commitment to MLOps and ML infrastructure modernization
Unless there are deep pre-existing capabilities, most organizations find it optimal to at least complement internal teams through external partnerships.
Leveraging AI SaaS Solutions
If speed and convenience are higher priorities than customization, turnkey SaaS products can provide pre-built models for common use cases like process automation, image classification and natural language processing. With off-the-shelf solutions:
Faster to trial and implement due to productized models and interfaces
Limited tuning to internal data
Can enable quicker proof-of-concepts but less differentiation
Ongoing vendor dependencies
Evaluating fit-for-purpose along both technical and business dimensions is key before committing long-term.
Partnering with AI Experts
Skilled consulting partners with vertical domain expertise offer a third path - customized yet accelerated implementing AI in business. The benefits of working with external AI specialists include:
Augmenting gaps in internal skills with flexibility
Applying institutional knowledge to avoid pitfalls
Staying current with latest breakthrough techniques
Unblocking progress without need to train internal staff
Blending the strengths of productized solutions with expert guidance tailored to your use cases provides an advantageous balance of control, agility and capability development.
In the end success requires realistic self-assessment of where existing skills and solutions fall short both now and for the future. AI talent strategy and sourcing lie along a spectrum rather than binary make vs buy decisions. Prioritizing speed to impact and flexibility is what enables staying ahead.
Now we dive deeper into the vital steps of actually integrating AI operationally.
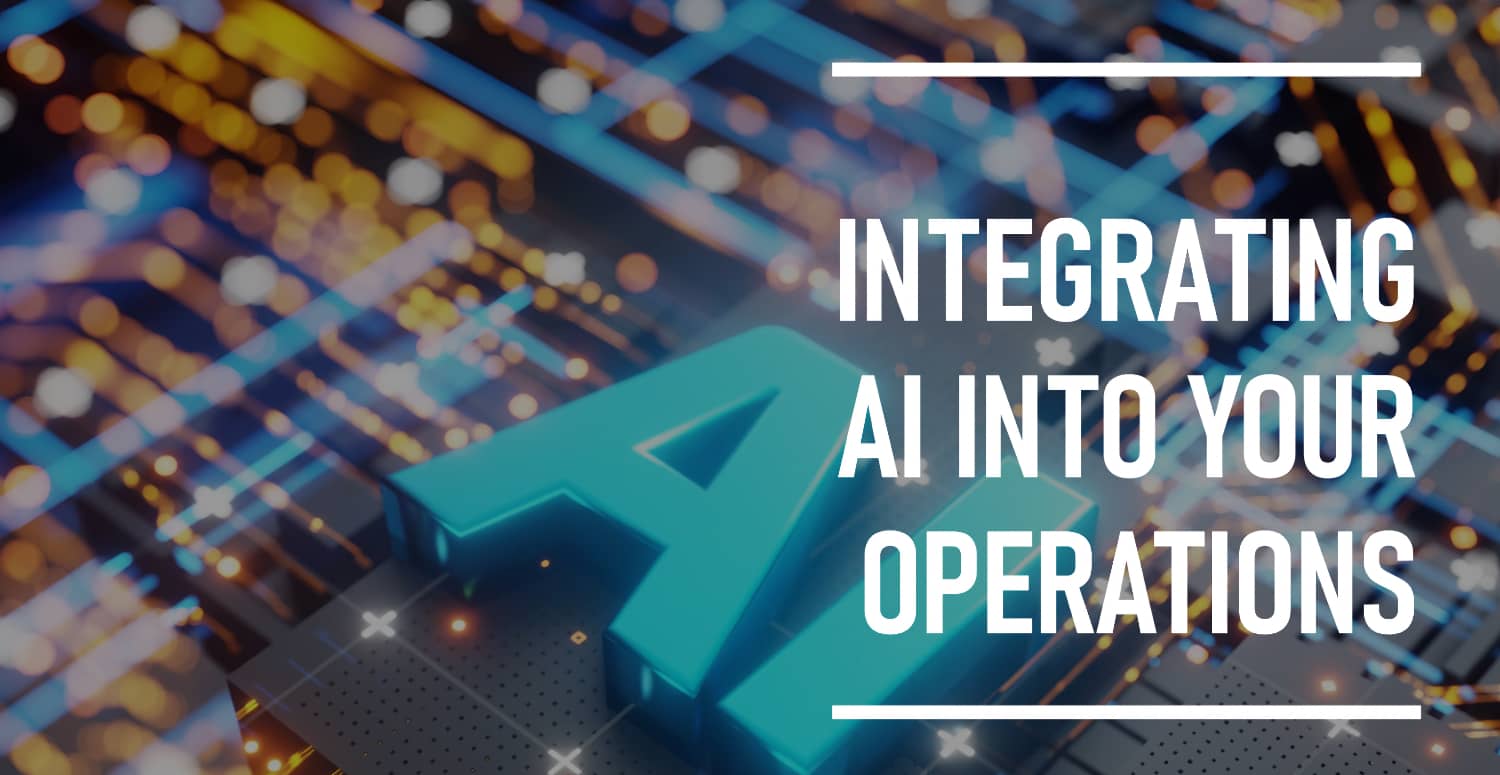
Integrating AI into Your Operations
With foundational data, infrastructure, talent and an overarching adoption roadmap established, the hands-on work of embedding machine learning into business processes can begin through well-orchestrated integration.
Much like traditional software development lifecycles, introducing AI-based capabilities requires upfront planning and phased testing before being ready for full production deployment.
Develop an Integration Plan
Define how AI will interoperate with surrounding processes and systems:
What inputs are needed for the model? How will data pipelines or services feed predictions to downstream consumers?
Does the AI component plug into or replace part of existing software workflows?
Are there new user interfaces or reports required for staff to leverage outputs?
Have fallback methods been defined if models degrade in performance over time?
Scripting integration touch points up front is vital for smooth AI implementation in your company.
Address Security and Privacy
Since sensitive data will flow through these AI systems, proper controls must govern:
What data masking, encryption or access policies are needed for handling regulated data?
How will credentials, secrets and model artefacts be secured across their lifecycle?
What compliance auditing capabilities need integration?
Drive Adoption Through Training
Make sure staff understand the context of how AI decisions augment their roles:
Train on basics of how the algorithms work to build trust
Enable easy ways to provide input on model relevancy over time
Highlight use cases where human discretion still overrides outputs
Proactive and continuous training is key to unlocking potential and benefit from implementing AI.
Monitor for Model Decay
Plan ongoing model monitoring once in production:
Data drift, concept drift, and outlier detection should trigger automatic retraining
Implement performance dashboards and reports operational leaders will consume
Leverage MLOps techniques like canary deployments for controlled model updates
AI lifecycle governance ensures reliability at scale.
The process does not end at deployment - truly embedding responsibilities for retraining, enhancement and accountability solidifies ROI.
Next we will cover how to perpetuate a cycle of continuous enhancement powered by AI.
Embracing AI for Continuous Improvement
The most transformative organizations view AI not as a one-time project but rather as an engine to drive an intelligent, data-driven culture focused on perpetual improvement.
They recognize success metrics evolve quickly, so models require constant tuning. They incentivize data sharing, ideation and governance from the edge rather than just the center. And they never stop incrementally expanding the footprint of experimentation with intelligent systems.
Encouraging a Culture of Data-Driven Decisions
Enable teams closest to your customers to specify enhancement opportunities or new applications of AI.
Provide sandbox tools for accessible prototyping without bottlenecks. Reward sharing of insights unlocked, not just utilization of existing reports.
Funding "Model Marketplaces"
Centralize access to reusable libraries of pretrained models, frameworks and pipelines.
Shift from always custom building to remixing and fine-tuning existing components. Curate based on performance and business impact.
Engineering for Responsible AI
Set checks and balances for automation vs augmentation. Mandate transparency not just accuracy.
Build trust by enabling staff to easily interpret predictions affecting them. Facilitate responsible data collection.
Never Stop Reskilling
Continually expose more staff to basics of data concepts, analytics tools, and AI interpretability.
Rotate department leaders through immersive experiences to motivate spreading capabilities wider and deeper.
Achieving outsized returns from AI investments relies on ingraining this forward-looking, entrepreneurial posture across the organization.
The playbook detailed here serves as guideposts for structuring and sequencing this transformation - but realizing the full value requires pushing AI implementation steps from an agenda item to a cultural cornerstone.
Implementing AI to Unlock Data-Driven Transformation
The promise of AI to revolutionize business is indisputable. However, technical feasibility alone does not guarantee effective adoption or positive ROI. Thoughtful planning and change management are indispensable.
This guide has aimed to demystify AI implementation by providing an actionable playbook - assessing your organization's readiness, formulating a pragmatic roadmap, determining the optimal methodology, and embracing integration as a catalyst for continuous improvement.
Success requires grounding in clear business objectives, organizational readiness for emerging technologies, and high-quality data. Strategy must align diverse stakeholders to balance short-term returns with long-term investments into infrastructure, while still moving aggressively.
Carefully orchestrating proof of concepts into pilots, and pilots into production systems allows accumulating experience. However the real breakthrough comes from ultimately fostering a culture hungry to incorporate predictive intelligence into daily decisions and workflows.
The future will undoubtedly bring unforeseen advances in artificial intelligence. Yet the foundations and frameworks described here will offer durable guidance. With eyes wide open to both profound opportunities and risks, thoughtful adoption of AI promises to shape tomorrow's data-driven enterprises.