In today's fast-paced business landscape, data-driven decision-making has become a critical factor for success. According to a recent study, 96% of business leaders agree that AI and machine learning can significantly improve decision-making processes. As companies face challenges such as tough competition, evolving customer demands, and data silos, the need for powerful tools like AI analytics is growing rapidly.
Challenge | Traditional Methods | AI Analytics Solution |
Abundant data | Limited processing capabilities | Efficient processing of large datasets |
Insights extraction | Manual analysis | Automated pattern recognition |
Decision-making | Gut instinct | Data-driven insights |
AI analytics offers a revolutionary approach to business decision-making by leveraging advanced technologies such as machine learning, natural language processing (NLP), neural networks, and deep learning. These tools enable businesses to process vast amounts of structured and unstructured data, uncover hidden patterns, and generate actionable insights in real-time.
In this article, we will explore how to use AI in business analytics to drive targeted decisions that foster growth and success. We'll delve into the key features of AI analytics, its benefits, and practical steps for implementing these cutting-edge tools in your organization. By harnessing the power of AI, businesses can gain a competitive edge, optimize operations, and deliver personalized experiences that delight customers and boost the bottom line.
What are AI Analytics?
AI analytics is the process of using advanced machine learning and AI techniques to extract valuable insights from large and complex datasets. Unlike traditional data analysis methods, AI analytics leverages the power of algorithms to identify patterns, predict outcomes, and make data-driven decisions.
Key Features of AI Analytics
Machine Learning
AI algorithms learn from data to identify patterns and trends
Continuously improves accuracy and performance over time
Enables automated analysis of vast amounts of data
Predictive Modeling
Utilizes historical data and current trends to forecast future outcomes
Helps businesses anticipate customer behavior, market shifts, and potential risks
Allows proactive decision-making and strategic planning
Natural Language Processing (NLP)
Enables AI to understand and analyze unstructured data like text and voice
Extracts insights from customer feedback, social media, and other text-based sources
Facilitates sentiment analysis and customer understanding
Neural Networks and Deep Learning
Advanced AI techniques inspired by the human brain
Capable of processing and analyzing large, complex datasets
Enables sophisticated applications like image and speech recognition
Feature | Traditional Analytics | AI Analytics |
Data processing | Manual, time-consuming | Automated, efficient |
Pattern recognition | Limited, prone to human bias | Advanced, unbiased |
Predictive capabilities | Basic, relies on historical data | Sophisticated, incorporates real-time data |
Unstructured data analysis | Difficult, often overlooked | Seamless, provides valuable insights |
By leveraging these key features, AI analytics empowers businesses to make targeted decisions based on data-driven insights. It enables organizations to process and analyze vast amounts of data efficiently, uncover hidden patterns, and generate actionable intelligence that drives growth and success.
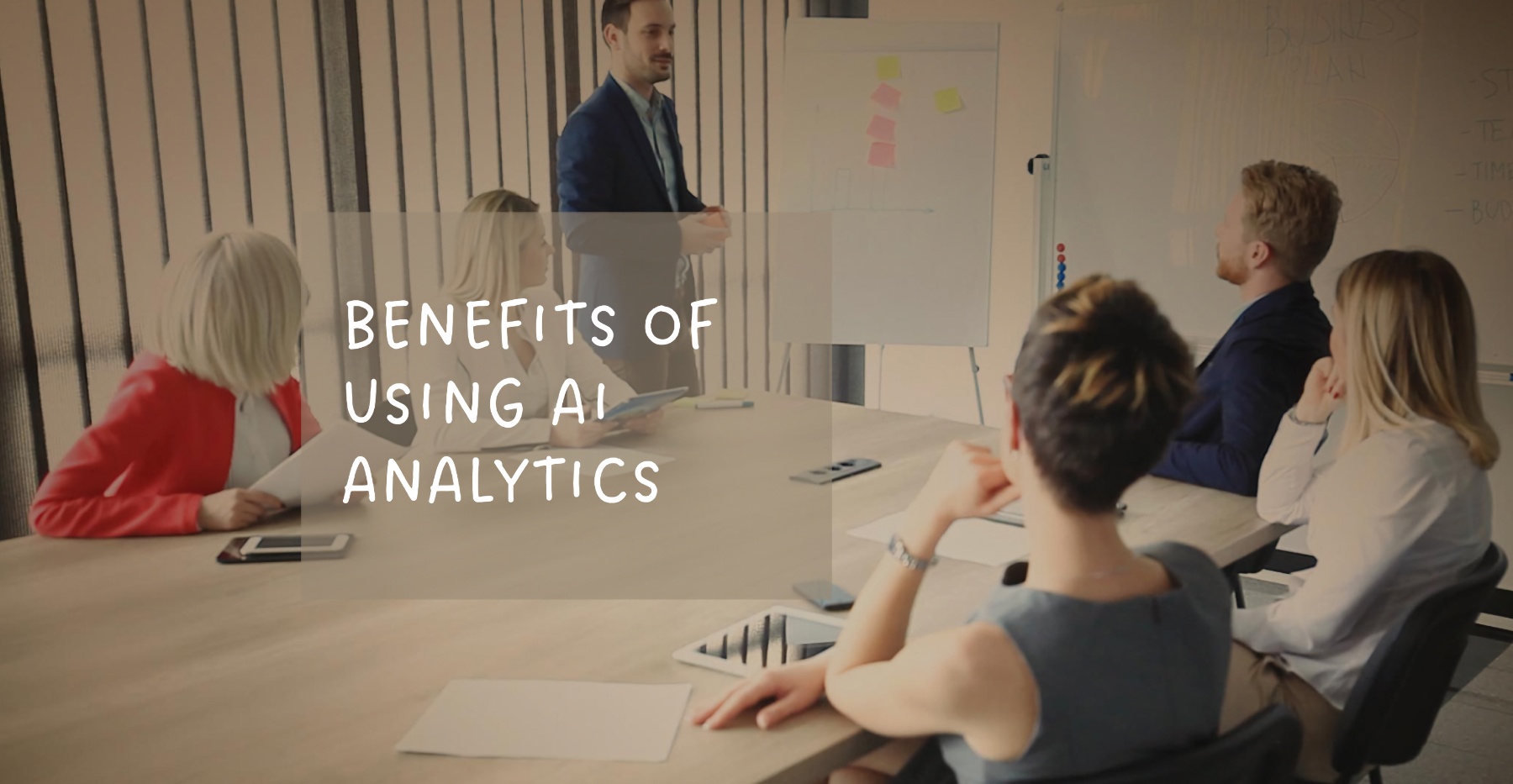
Benefits of Using AI Analytics for Targeted Business Decisions
Implementing AI analytics in your organization can lead to numerous benefits that drive targeted decision-making and business success. Let's explore some of the key advantages:
Improved Customer Insights
AI analytics enables businesses to gain a deeper understanding of their customers by analyzing vast amounts of data from various sources. By leveraging machine learning and NLP, AI can uncover valuable insights into customer preferences, needs, and behavior. This knowledge empowers organizations to:
Develop products and services that better align with customer demands
Create targeted marketing campaigns that resonate with specific audience segments
Enhance customer service by anticipating and addressing customer needs proactively
Enhanced Operational Efficiency
AI-driven analytics can help streamline business processes and optimize operations. By analyzing data from various sources, such as supply chain, production, and logistics, AI can identify inefficiencies and provide data-driven recommendations for improvement. Some key areas where AI analytics can enhance operational efficiency include:
Predictive maintenance: Anticipating equipment failures and scheduling maintenance proactively
Inventory optimization: Ensuring optimal stock levels based on demand forecasting and supply chain data
Resource allocation: Optimizing workforce and resource distribution based on real-time data and predictive modeling
Data-Driven Risk Management
AI analytics enables businesses to identify and mitigate potential risks by analyzing vast amounts of data from various sources. By leveraging predictive analytics and machine learning, organizations can:
Monitor market trends and competitor activity to identify potential threats and opportunities
Detect fraudulent activities and anomalies in financial transactions
Assess credit risk and make informed lending decisions
Personalized Experiences
One of the most significant benefits of AI analytics is its ability to enable personalized experiences for customers. By analyzing customer data, such as browsing history, purchase behavior, and social media interactions, AI can provide tailored recommendations and experiences. This personalization can lead to:
Increased customer satisfaction and loyalty
Higher engagement and conversion rates
Improved customer lifetime value
Benefit | Traditional Analytics | AI Analytics |
Customer insights | Limited, based on demographic data | Deep, based on behavior and preferences |
Operational efficiency | Reactive, relies on historical data | Proactive, utilizes real-time data and predictive modeling |
Risk management | Manual, prone to human error | Automated, data-driven, and real-time |
Personalization | Broad, segment-based | Granular, individual-level |
By harnessing the power of AI analytics, businesses can make targeted decisions that drive growth, mitigate risks, and enhance customer experiences. The ability to process and analyze vast amounts of data in real-time enables organizations to stay ahead of the competition and adapt to the ever-changing business landscape.
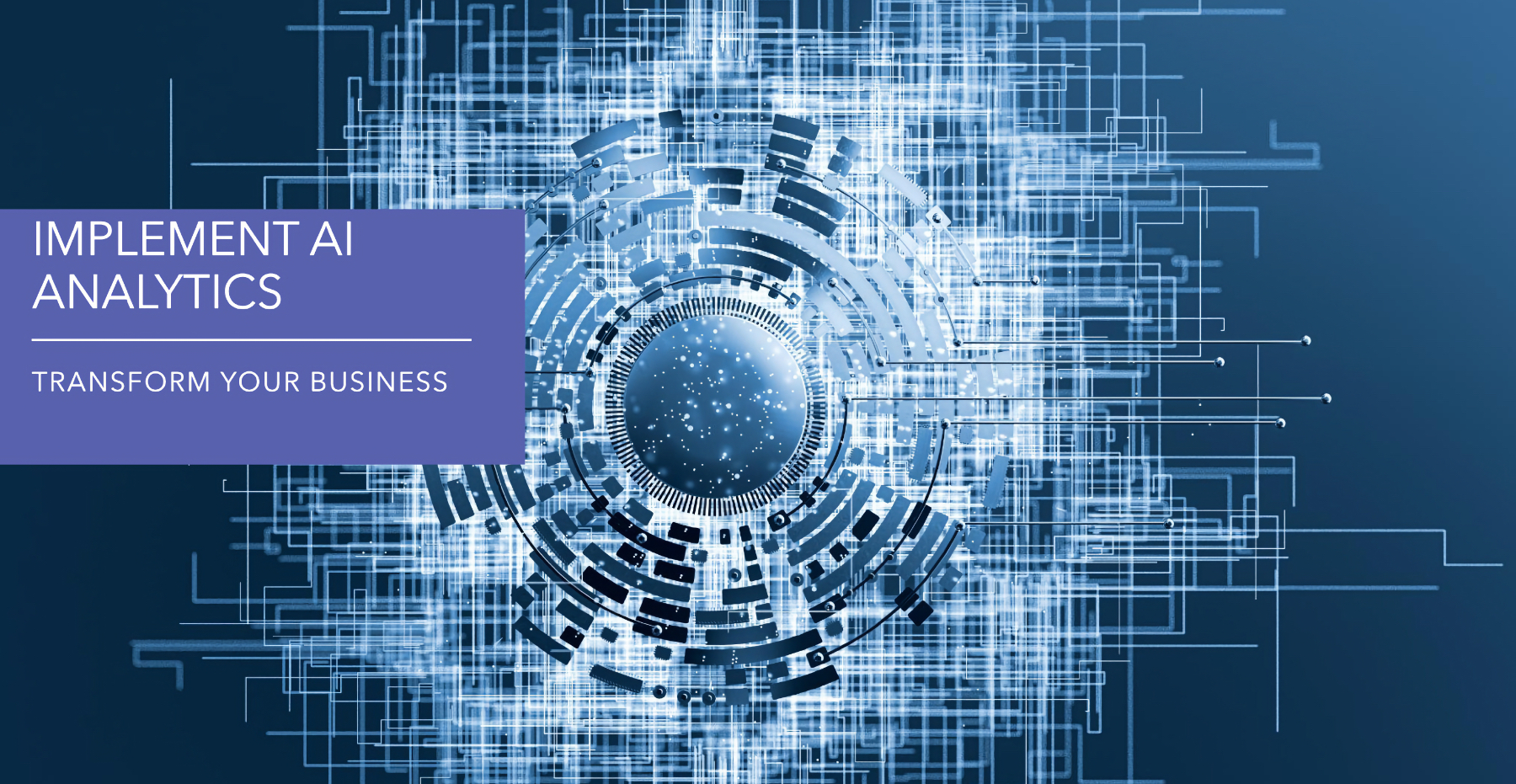
How to Implement AI Analytics in Your Business
Implementing AI analytics in your organization requires a strategic approach that aligns with your business goals and resources. Here are the key steps to successfully integrate AI-driven decision-making into your operations:
Define Your Goals
Before embarking on your AI analytics journey, it's crucial to clearly define your business objectives. Identify the specific areas where data-driven insights can make the most significant impact, such as:
Enhancing customer experience
Optimizing supply chain and inventory management
Improving marketing campaign effectiveness
Streamlining financial processes and risk management
Identify Relevant Data
Once you have defined your goals, the next step is to identify and collect relevant data from various sources. This may include:
Customer data (e.g., demographics, purchase history, feedback)
Financial records (e.g., sales, expenses, cash flow)
Operational data (e.g., production, inventory, logistics)
Market research and competitor analysis
Ensure that the data is clean, accurate, and properly formatted for analysis.
Choose the Right AI Tools
There are numerous AI analytics tools available in the market, each with its own strengths and limitations. When selecting an AI tool, consider factors such as:
Compatibility with your existing systems and data sources
Scalability to accommodate future growth and data volume
User-friendliness and ease of integration into your workflows
Cost and return on investment (ROI)
Some popular AI analytics tools include:
Tool | Key Features |
Sprout Social | Social media analytics, sentiment analysis, competitor analysis |
Power BI | Data visualization, predictive modeling, real-time dashboards |
MonkeyLearn | Text analysis, customer feedback analysis, sentiment analysis |
Tableau | Data visualization, predictive analytics, real-time collaboration |
Sisense | Data integration, predictive analytics, natural language processing |
Integrate with Existing Systems
To maximize the value of AI analytics, it's essential to seamlessly integrate the AI tools with your existing business systems. This integration ensures a smooth flow of data and enables real-time analysis and decision-making. Consider the following integration points:
Customer Relationship Management (CRM) systems
Enterprise Resource Planning (ERP) systems
Marketing automation platforms
Supply chain management systems
Build a Team with the Right Skills
Implementing AI analytics requires a team with a diverse set of skills, including:
Data scientists and analysts to develop and refine AI models
IT professionals to ensure seamless integration and data security
Domain experts to provide business context and interpret results
Change management specialists to drive adoption and organizational alignment
Invest in training and development to ensure your team has the necessary skills and knowledge to leverage AI analytics effectively.
By following these steps and leveraging the right tools and expertise, businesses can successfully implement AI analytics and harness its power for targeted decision-making. The key is to start small, iterate based on results, and continuously refine your approach to maximize the value of AI-driven insights.
Case Studies and Examples
To illustrate the transformative power of AI analytics in driving targeted business decisions, let's explore some real-world examples across various industries:
Healthcare: Predictive Analytics for Disease Detection
A leading healthcare provider leveraged AI analytics to improve the early detection of diseases. By analyzing patient data, including medical records, genetic information, and lifestyle factors, the AI system was able to identify patterns and risk factors associated with specific diseases. This enabled doctors to provide proactive care and intervene early, resulting in improved patient outcomes and reduced healthcare costs.
Retail: Inventory Optimization and Customer Experience
A major retail chain implemented AI analytics to optimize their inventory management and enhance customer experience. By analyzing sales data, customer behavior, and supply chain information, the AI system provided real-time insights into demand forecasting and inventory levels. This enabled the retailer to reduce stockouts, minimize waste, and ensure the right products were available at the right time. Additionally, by leveraging NLP and sentiment analysis, the retailer was able to analyze customer feedback and social media conversations to identify areas for improvement and personalize the shopping experience.
Finance: Fraud Detection and Risk Assessment
A global financial institution employed AI analytics to combat fraud and improve risk assessment. By analyzing vast amounts of transactional data, the AI system was able to detect patterns and anomalies indicative of fraudulent activities. This enabled the institution to proactively prevent fraud and minimize financial losses. Furthermore, by leveraging machine learning algorithms, the AI system was able to assess credit risk more accurately, enabling data-driven lending decisions and reducing default rates.
Manufacturing: Predictive Maintenance and Quality Control
A leading manufacturing company implemented AI analytics to optimize their production processes and improve quality control. By analyzing sensor data from equipment and production lines, the AI system was able to predict potential machine failures and schedule proactive maintenance. This reduced downtime, increased overall equipment effectiveness (OEE), and minimized maintenance costs. Additionally, by leveraging computer vision and deep learning, the AI system was able to detect defects and quality issues in real-time, enabling quick corrective actions and reducing waste.
Industry | Application | Benefits |
Healthcare | Disease detection | Early intervention, improved patient outcomes |
Retail | Inventory optimization | Reduced stockouts, minimized waste |
Finance | Fraud detection | Proactive prevention, minimized losses |
Manufacturing | Predictive maintenance | Reduced downtime, increased OEE |
These case studies demonstrate the diverse applications and benefits of AI analytics across various industries. By leveraging AI-driven insights, businesses can make targeted decisions that drive efficiency, reduce costs, and enhance customer experiences. As more organizations embrace AI analytics, we can expect to see even more innovative use cases and success stories in the future.
Unleashing the Power of AI Analytics for Smarter Business Decisions
In today's data-driven world, AI analytics has emerged as a game-changer for businesses looking to make targeted, informed decisions. By leveraging advanced technologies like machine learning, natural language processing, and deep learning, organizations can harness the power of their data to gain deep insights, optimize processes, and drive innovation.
Throughout this article, we have explored the key features and benefits of AI analytics, including improved customer understanding, enhanced operational efficiency, data-driven risk management, and personalized experiences. We have also outlined a strategic approach to implementing AI analytics in your business, from defining goals and identifying relevant data to choosing the right tools and building a skilled team.
The real-world case studies and examples showcased the transformative impact of AI analytics across various industries, from healthcare and retail to finance and manufacturing. These success stories demonstrate the vast potential of AI-driven decision-making in driving business growth, reducing costs, and creating competitive advantages.
As the volume and complexity of data continue to grow, the importance of AI analytics will only increase. Businesses that embrace this technology and embed it into their decision-making processes will be better positioned to navigate the challenges and opportunities of the future.
By harnessing the power of AI analytics, organizations can unlock the full potential of their data, make smarter decisions, and achieve their strategic objectives. The journey to AI-driven decision-making may be complex, but the rewardsâin terms of efficiency, innovation, and customer satisfactionâare well worth the effort.