Custom AI solutions refer to artificial intelligence systems that are built to address specific business challenges and requirements. Unlike off-the-shelf AI tools which provide generic functionality, custom AI software is fine-tuned to enhance processes and insights unique to an organization.
The true power of AI lies in its customization potential. With the right data and objectives, custom AI development can revolutionize everything from customer experiences to operational efficiency across diverse industries:
Manufacturing: Custom computer vision solutions conduct visual quality checks and detect defects.
Healthcare: Custom NLP algorithms extract insights from patient health records.
Finance: Customized AI models detect fraud, predict market movements, and inform trading strategies.
Retail: Custom conversational AI chatbots provide personalized recommendations and customer support.
The versatility of custom solutions enables the transformation of entire business models - not just individual tasks.
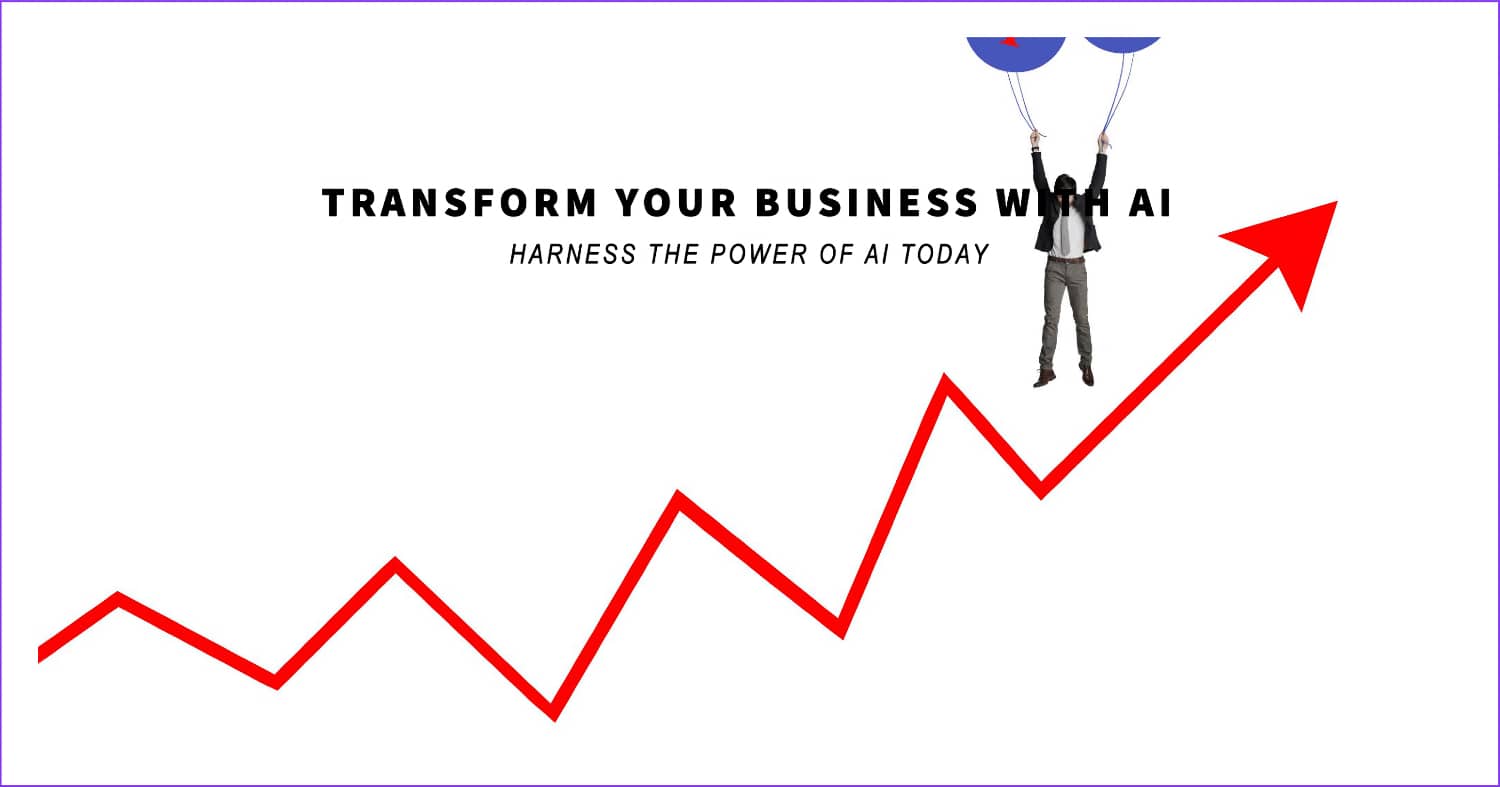
Harnessing the Power of AI for Business Transformation
Custom AI solutions unlock tremendous potential to enhance business capabilities when strategically implemented.
Integrating tailored artificial intelligence throughout operations and processes facilitates data-driven decision making and innovation at scale:
Sales and Marketing: AI-generated insights on buyer personas, demand forecasting, and pricing optimization provide competitive advantages by elevating campaign performance.
Operations: Optimized workflows, predictive maintenance, and anomaly detection enabled by customized models drive efficiency gains and cost savings.
Products: Enhanced personalization, quality control innovations, and accelerated feature development powered by tailored computer vision and natural language processing create differentiation.
Custom AI software also advances customer experiences through conversational AI systems like personalized chatbots tailored to business needs using domain-specific data. This boosts satisfaction and loyalty.
Additionally, developing in-house AI solutions tailored to proprietary data secures competitive edges and concentrates value.
However, business leaders must clearly identify focus areas for AI transformation aligned to strategic goals. Prioritizing high-impact initiatives maximizes returns on investments in customization. Cross-functional coordination is also key.
The surge in AI adoption is ultimately driven by the quest for innovations and advantages unique to each organization. Custom solutions make it possible to turn AI into transformative business capabilities. But concerted efforts are required to harness the full potential.
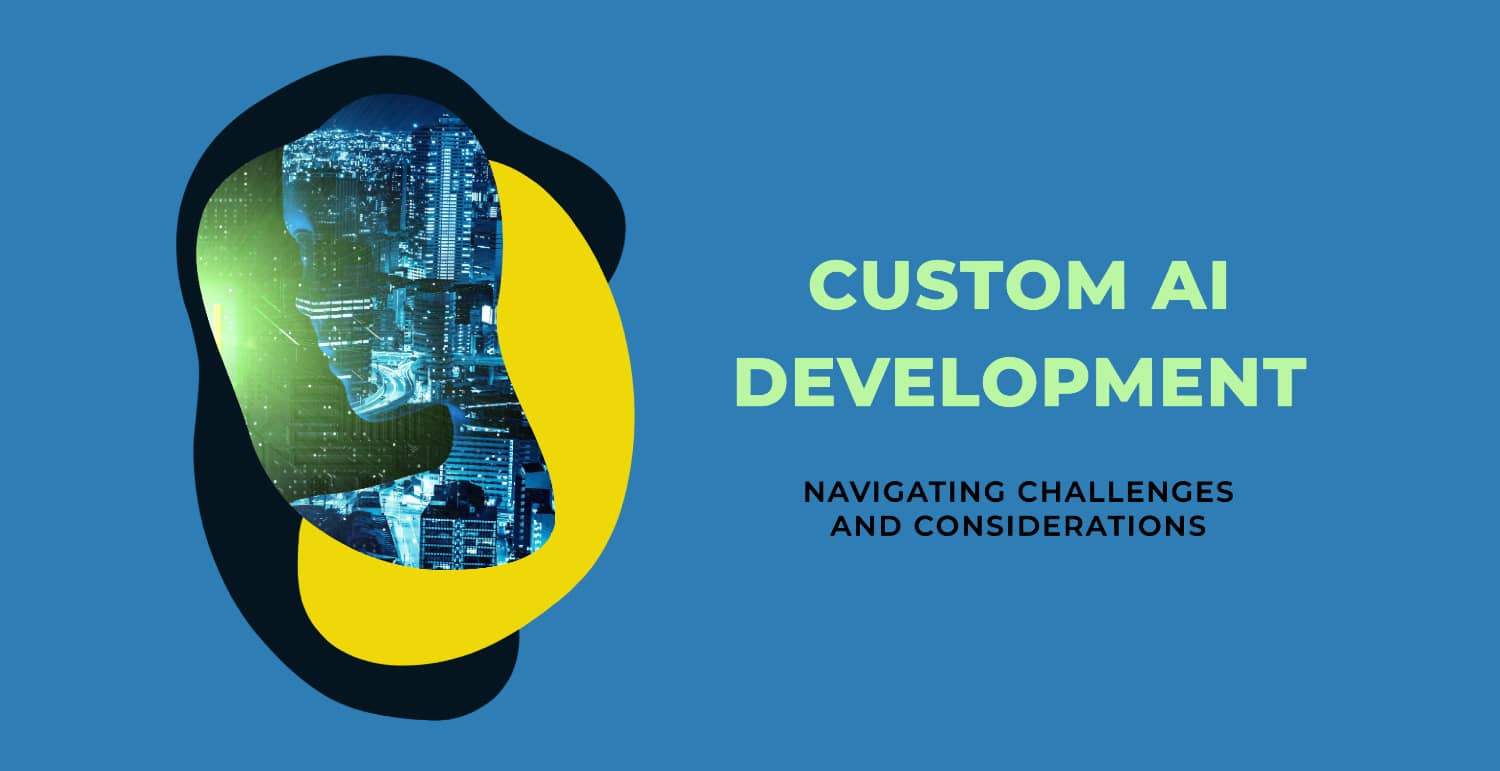
Navigating the Challenges and Considerations of Custom AI Development
However, leaders must address key challenges in custom AI development to drive successful implementations:
Data Collection and Labeling: Relevant, high-quality, unbiased and balanced data is crucial for training accurate AI models. Data annotation also requires substantial expertise.
ML Model Development: Selecting, testing, and optimizing the right machine learning algorithms and techniques for given tasks takes specialized skills.
Monitoring and Maintenance: Continuously tracking performance indicators and updating models ensures reliability over time while avoiding technical debt.
Customization necessitates aligning AI objectives with business goals through interdisciplinary collaboration among domain experts, data scientists, engineers and IT teams.
AI consulting services that provide guidance on best practices can accelerate this process. But ultimately, business leaders drive progress by directing teams and investments towards high-priority initiatives.
Other considerations include:
Evaluating total cost of ownership tradeoffs between custom solutions vs off-the-shelf tools
Ensuring scalability of infrastructure and operations for enterprise-wide adoption
Protecting data security through governance policies and access controls
Upskilling talent and expanding capabilities to support ongoing enhancements
Achieving ROI on customized AI relies on navigating organizational and operational nuances - not just technical complexity. Cross-functional orchestration combined with managed experimentation and gradual scaling enables sustainable success.
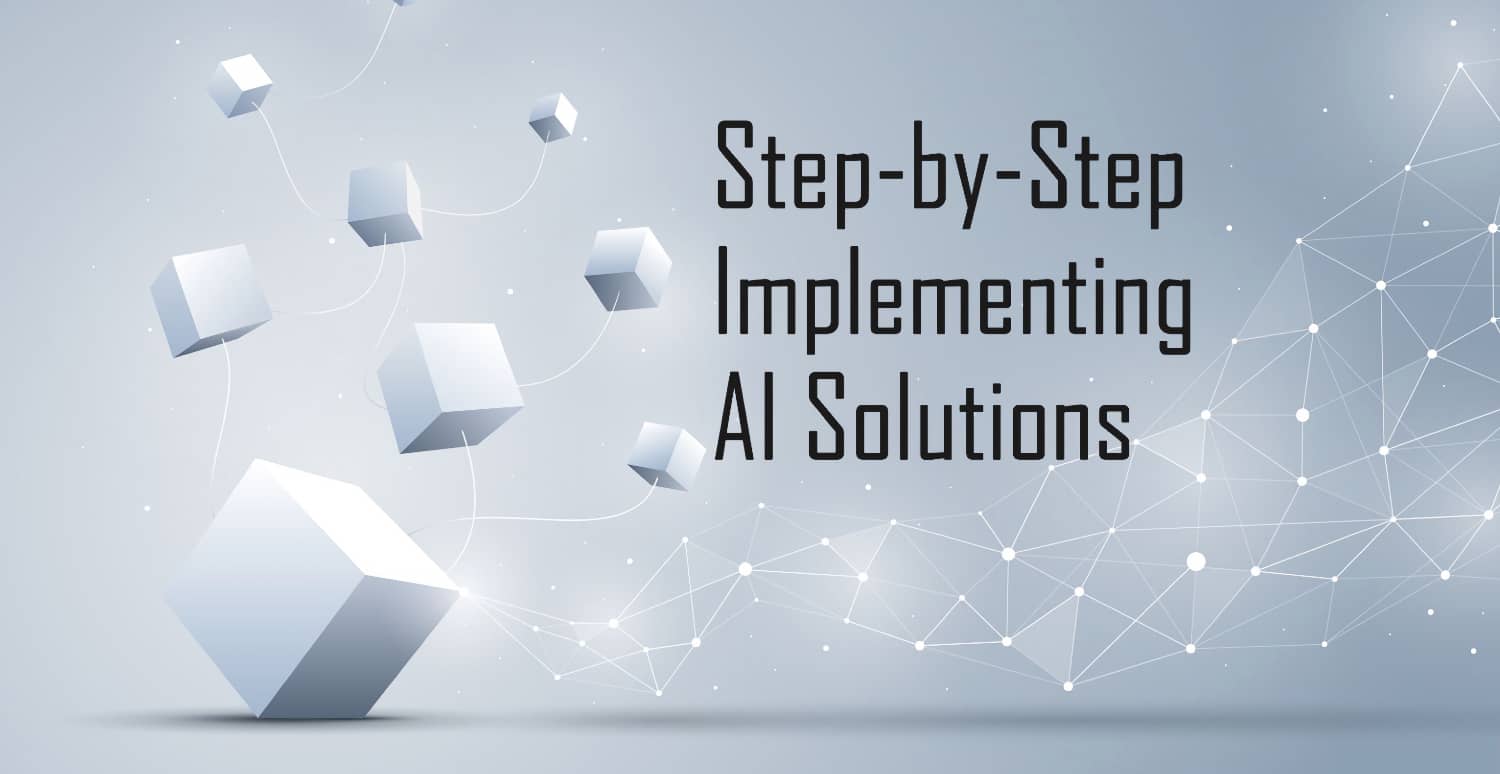
A Step-by-Step Guide to Implementing Custom AI Solutions
Successfully rolling out customized AI entails a systematic approach:
Define business challenges, objectives and data requirements through cross-functional workshops. Prioritize high-impact use cases.
Assemble relevant datasets and preprocess data to ensure quality and suitability for model development.
Label, annotate and validate data for training AI models according to project needs. Obtain external support as needed.
Select and test appropriate AI algorithms with guidance from ML development partners. Evaluate multiple techniques.
Train, evaluate and fine-tune AI models iteratively to optimize performance on key indicators.
Integrate optimized models into business processes through APIs and pipelines.
Monitor performance indicators and model drift to ensure reliability. Create feedback loops between usage data and model improvement efforts.
Retrain and update models periodically to sustain competitiveness. Explore ensemble approaches combining outputs of multiple models.
This lifecycle methodology ensures custom solutions achieve optimal and lasting results. The key is taking an agile, experimental approach while maintaining alignment with overarching business objectives at each step.
AI consultants and seasoned partners provide invaluable support throughout this process - from strategizing roadmaps to troubleshooting implementations. Custom AI is a continuous optimization practice.
Case Studies of Successful Custom AI Implementation
Real-world examples showcase the diverse applications and tremendous impact of tailored AI solutions across sectors:
A healthcare provider developed a custom NLP solution to automate clinical documentation analysis - improving clinician productivity by 20% and patient outcomes.
An online retailer implemented customized recommendations using purchase history and clickstream data - increasing cross-sells by 12% and revenue by 8%.
A bank trained custom AI models to detect fraudulent transactions in real-time - saving over $2 million annually.
A manufacturer deployed automated visual inspection with custom computer vision - reducing defect rates by 30% and operational costs.
Industry | Use Case | Impact |
Healthcare | Clinical documentation analysis | 20% improved clinician productivity |
Retail | Personalized recommendations | 12% increased cross-sells |
Banking | Fraud detection | $2M+ annual savings |
Manufacturing | Visual quality inspection | 30% reduced defects |
These examples demonstrate the versatile applications and tremendous ROI delivered by tailored AI solutions across sectors.
The key success factor is alignment with unique business objectives coupled with strong execution integrating customized models into workflows. With the right approach, the possibilities are endless.
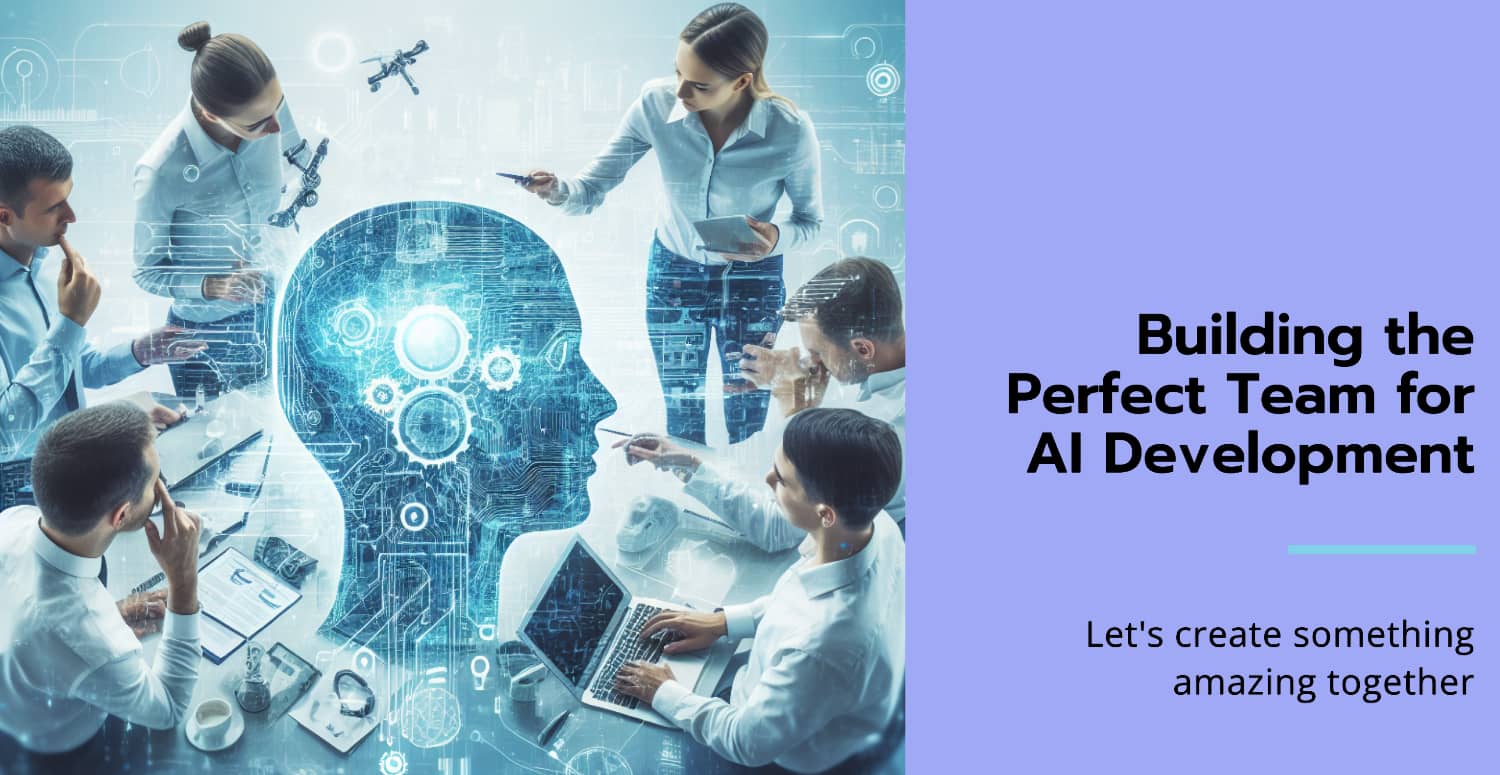
Building the Team for Custom AI Development
Successfully leveraging custom AI software requires coordination across a multifunctional team:
Data Scientists: Architect solutions, develop and optimize models.
Machine Learning Engineers: Build data infrastructure and pipelines.
Domain Experts: Contextualize business challenges and interpret model results.
IT Teams: Facilitate integration and monitor production systems.
Project Managers: Coordinate cross-functional collaboration and track progress.
Organizations also need to assess capabilities for:
Data collection, labeling and annotation
Cloud infrastructure and MLOps
Ongoing model maintenance
Strategic partnerships accelerate progress:
AI Consultants: Provide guidance on technology capabilities, best practices and implementation roadmaps.
ML Development Companies: Handle specialized tasks like computer vision and NLP.
Freelancers: Cost-effective talent for development and data labeling.
With leading expertise in modern approaches like deep learning and natural language processing, custom AI development partners fast-track high-impact implementations while transferring knowledge.
Ultimately, aligning priorities across stakeholders from technologists to business leaders ensures initiatives stay on track to achieve ROI - with the flexibility to refine based on insights gained during initial pilots. A collaborative culture and growth mindset are key.
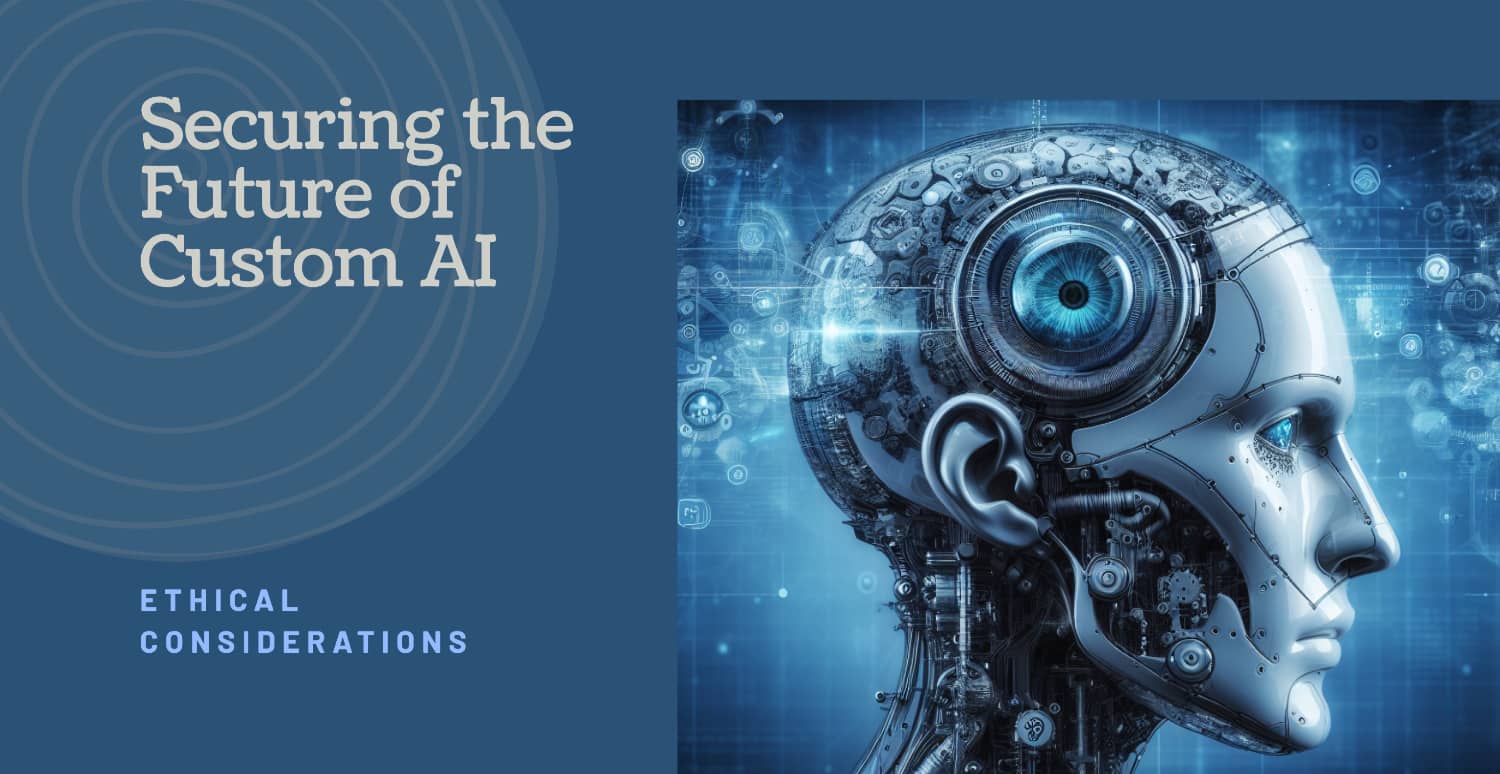
Securing the Future of Custom AI with Ethical Considerations
As AI becomes further embedded in business processes, accountability and transparency are crucial - especially for custom systems that impact key decisions or outcomes.
Prioritizing ethics and establishing robust data governance practices early in the custom AI development lifecycle facilitates trustworthy and socially responsible AI adoption at scale while mitigating risks like bias.
Key considerations include:
Ensuring datasets used for training models are inclusive, balanced and representative
Implementing rigorous model testing procedures focused on fairness and bias detection
Enabling accountability through documentation, reporting and auditing of AI systems
Protecting sensitive personal data through de-identification and access controls
Communicating transparently with stakeholders on system logic, use cases and limitations
Partners like AI consultants can provide guidance for organizations to instill responsible AI practices that align with emerging regulations globally around algorithmic transparency and accountability.
But ultimately, business leaders drive culture and policies to ensure custom solutions augment human intelligence - not undermine it. With conscientious leadership, customized AI can elevate business capabilities and social good simultaneously.
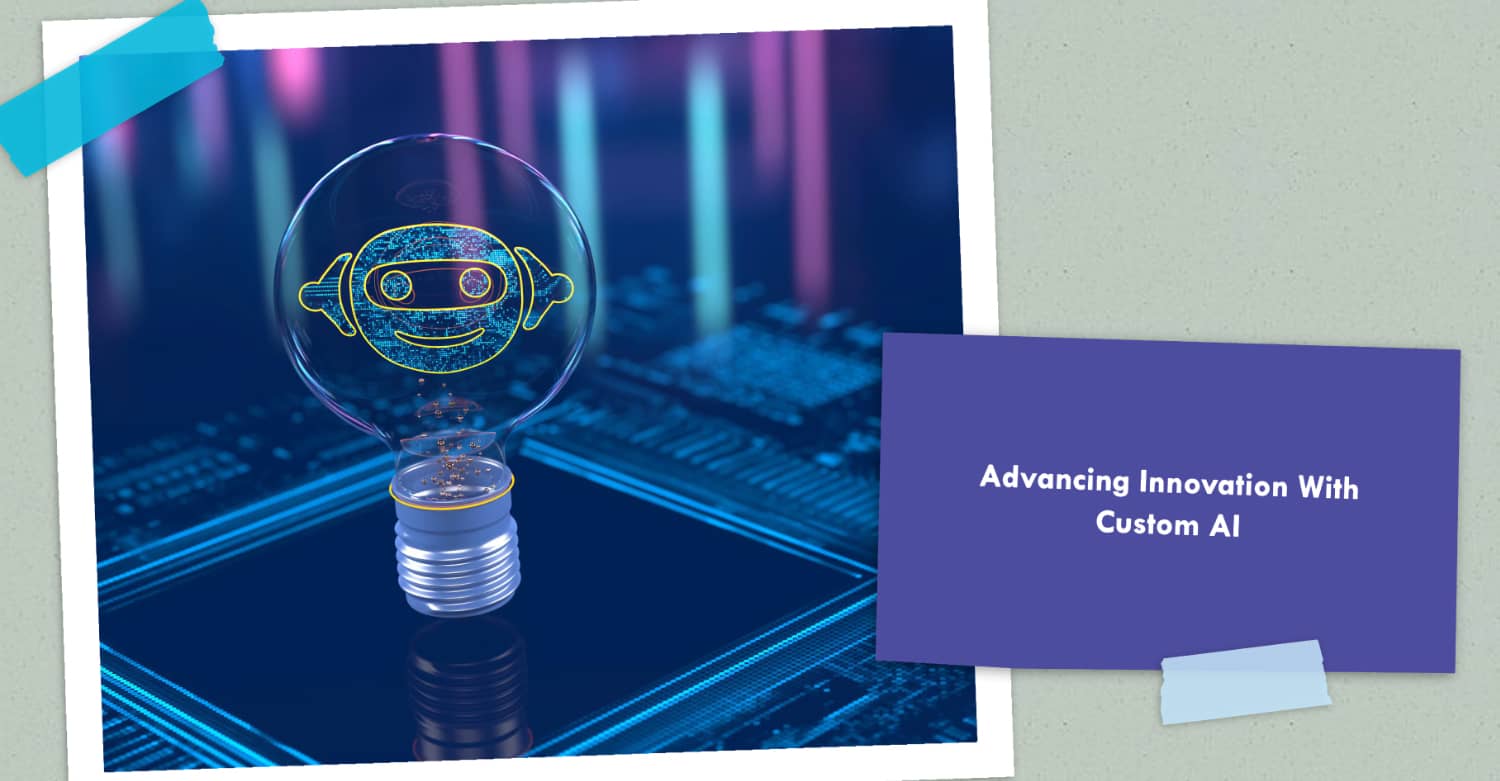
The Future of Custom AI: Advancing Innovation and Driving Competitiveness
Rapid advances in AI research and cloud-based development platforms will further democratize access to customization potential:
Automated Machine Learning (AutoML) streamlines model building by automatically applying different algorithms and hyperparameters. This allows non-experts to develop tailored solutions faster.
MLOps integrates and operationalizes the machine learning lifecycle - enabling continuous delivery of updates to production custom AI software.
Few-shot learning techniques facilitate training models with limited data. This expands customization reach to more niche applications.
These innovations combined with emerging techniques like neuro-symbolic AI and transformer architectures will shape the next frontier of possibilities with customized AI.
As off-the-shelf AI becomes table stakes in business operations, custom solutions will be the ultimate differentiator:
Underpinning competitive advantages through proprietary data. Unlocking new revenue streams. Accelerating optimizations unique to specific organizations. And ultimately elevating customer experiences through hyper-personalization.
Organizations that embrace custom development now will sustain market leadership well into the future. Partnering with specialized AI consultants and ML providers kickstarts progress.
Custom AI promises to remain at the forefront driving the next waves of innovation - while steering responsible tech progress positively impacting both businesses and society. The possibilities are boundless for leaders embracing customization with strategic vision and principled implementation.