Generative AI is rapidly transforming the landscape of business technology, offering unprecedented opportunities for innovation and efficiency. As organizations worldwide begin to recognize its potential, generative AI is set to reshape industries, redefine customer interactions, and revolutionize products and services. Its applications range from automating creative processes to enhancing decision-making capabilities.
However, effectively integrating generative AI into business strategies requires more than just technological adoption; it necessitates a deep reconsideration of existing business models and strategies. This article explores the importance of flexible model choices in scaling generative AI and provides best practices for successful implementation.
The Importance of Model Choices
In the dynamic world of generative AI, one-size-fits-all approaches are inadequate. Having a spectrum of model choices is necessary to:
Spur Innovation: A diverse palette of models fosters innovation by bringing distinct strengths to tackle a wide array of problems and adapt to evolving business needs and customer expectations.
Customize for Competitive Advantage: A range of models allows companies to tailor AI applications for niche requirements, providing a competitive edge.
Accelerate Time to Market: A diverse portfolio of models can expedite the development process, allowing companies to introduce AI-powered offerings rapidly.
Stay Flexible in the Face of Change: Various model choices allow businesses to pivot quickly and effectively when new trends or strategic shifts occur, maintaining agility and resilience.
Optimize Costs Across Use Cases: Different models have varying cost implications. By accessing a range of models, businesses can select the most cost-effective option for each application.
Mitigate Risks: A diverse portfolio of models helps mitigate concentration risks, helping to ensure that businesses remain resilient to the shortcomings or failure of one specific approach.
Comply with Regulations: A broad selection of models allows businesses to navigate the complex regulatory terrain and choose models that meet legal and ethical standards.
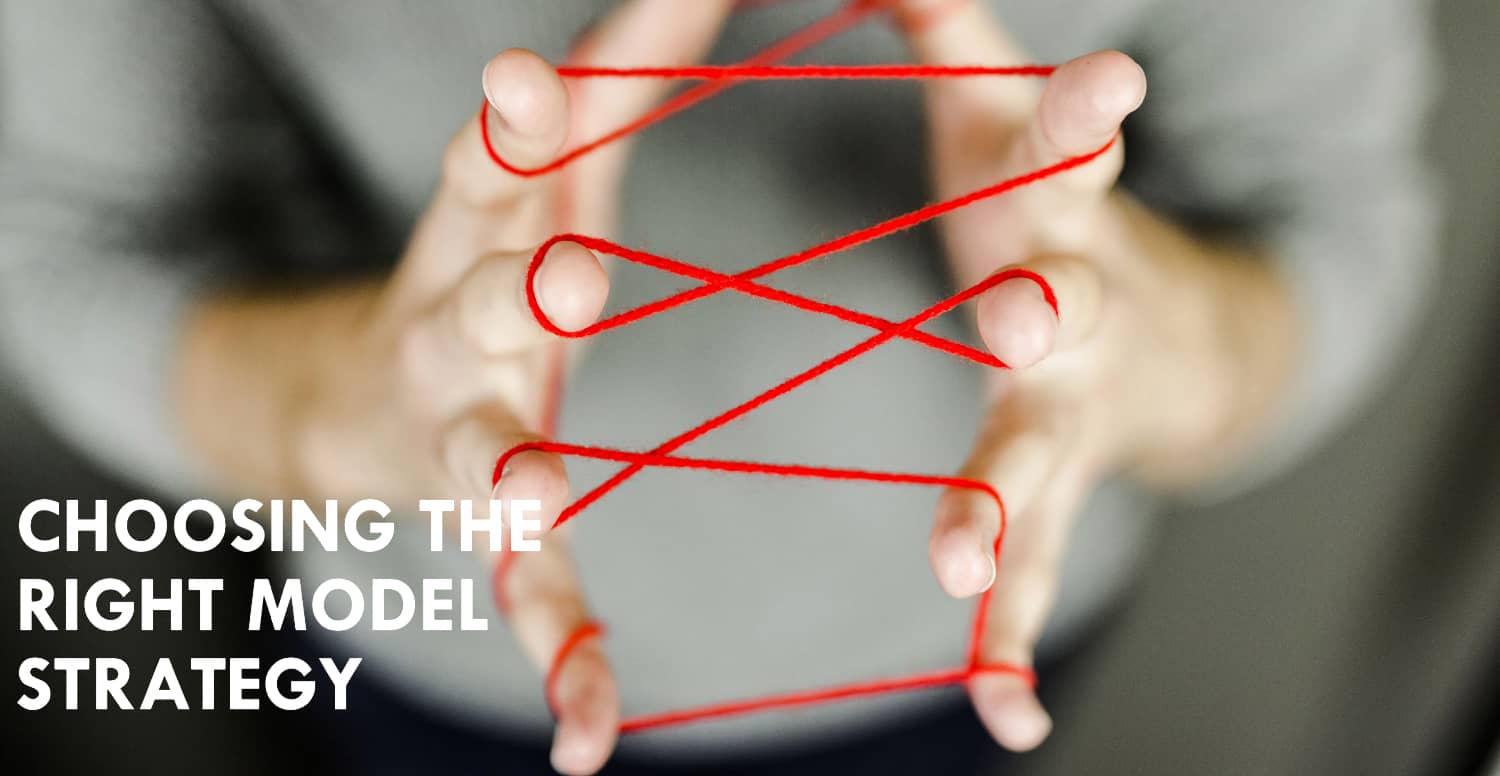
Choosing the Right Model Strategy
Businesses face a critical decision: use pre-built models, develop custom models, or fine-tune existing models. Each choice has its benefits and drawbacks, influenced by cost, expertise, time-to-market, and business needs.
Model Strategy | Pros | Cons |
---|---|---|
Developing Custom Models | - Tailored solutions | - Significant investment |
Fine-Tuning Pre-Built Models | - Leverage sophisticated AI | - Computational resources |
Adopting Pre-Built Models | - Cost-efficient | - Less customization |
For most businesses, using pre-built models with techniques like Retrieval Augmented Generation (RAG) and prompt engineering is recommended. This strategy allows for effective AI solutions tailored to specific problems without the overhead of building or fine-tuning models.
Hardware Investment: Rent vs. Own
When integrating generative AI, deciding between renting GPU hardware through cloud providers and owning hardware outright impacts both financials and technological agility.
Consideration | Renting GPUs | Owning GPUs |
---|---|---|
Cost | Pay-as-you-go | Significant upfront investment |
Scalability | Easily scale up or down | Limited by owned hardware |
Technological Agility | Access to latest GPU models | Requires frequent upgrades |
Control | Less control over hardware and software | Full control over hardware and software environment |
Renting GPU hardware through cloud providers offers advantages in scalability, cost efficiency, and technological agility. This approach maintains technological flexibility, manages costs effectively, and ensures AI implementations are supported by state-of-the-art hardware.
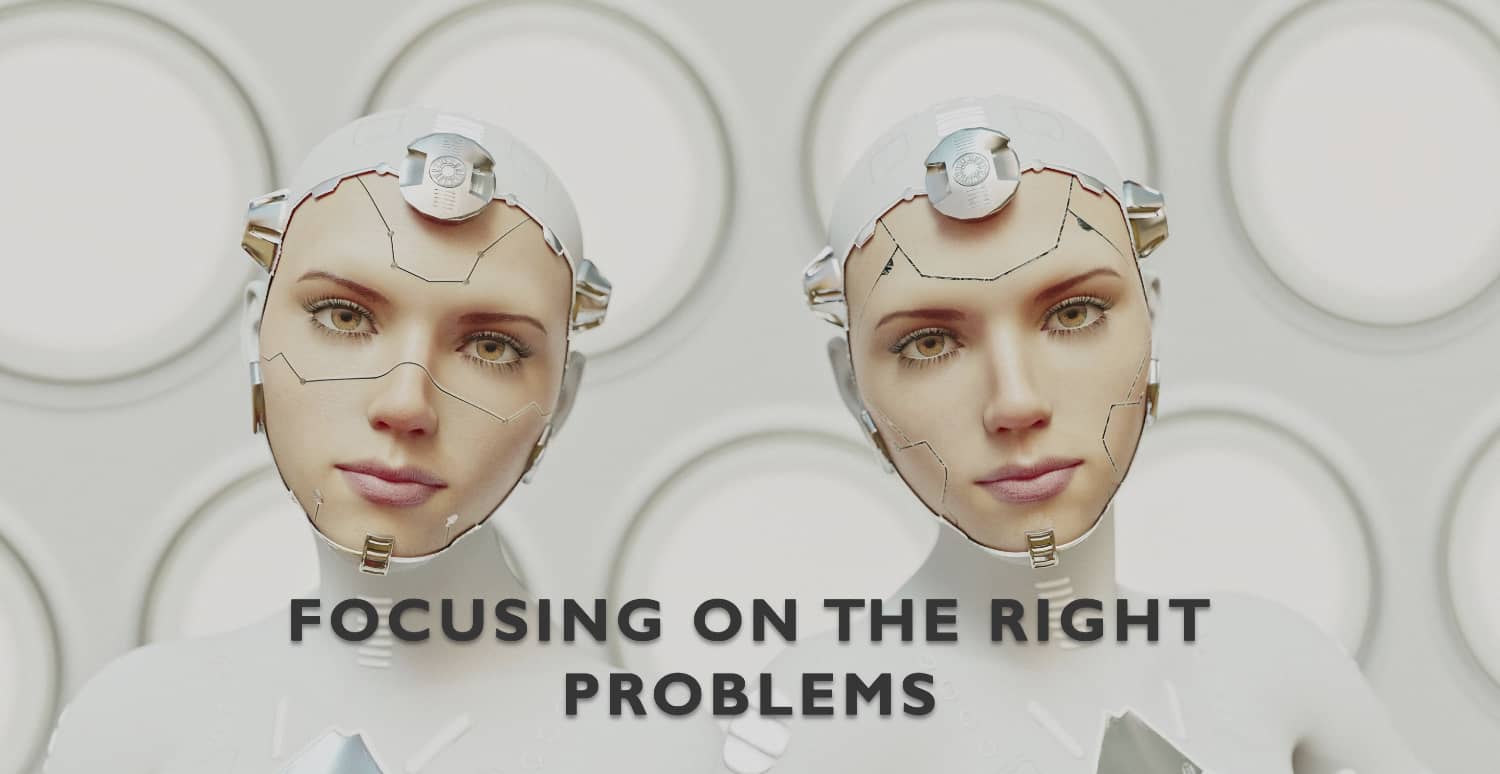
Focusing on the Right Problems
Identifying challenges that align with AI capabilities and add significant value is crucial for leveraging generative AI. Focusing on complex, high-impact problems can enhance competitive advantage and provide tangible benefits.
Importance of Targeting High-Impact Challenges: Generative AI excels at addressing complex issues requiring nuanced understanding and multi-faceted solutions. By targeting problems critical to core operations or strategic goals, businesses can leverage AI to drive substantial improvements in efficiency, customer satisfaction, and innovation.
Advantages of Expanding Context Windows in AI Models: Modern generative AI models, such as those built on the GPT architecture, increasingly handle larger context windows. This capability allows them to understand and process information over broader narratives or datasets, leading to more sophisticated reasoning and problem-solving abilities.
Adopting a Product Mindset in AI Strategy: Integrating AI strategy with a product operating model enables businesses to identify and prioritize problems that are most impactful. This alignment ensures that AI initiatives are closely tied to creating customer value and enhancing product offerings, driving more focused and effective AI deployments.
IBM watsonx Model Library
IBM watsonx offers a range of proprietary, open source, and third-party models, providing clients with a spectrum of choices to select the model that best fits their unique business, regional, and risk preferences.
Model Type | Examples |
---|---|
Proprietary Models | IBM Granite |
Open Source Models | GPT, BERT, YOLO |
Third-Party Models | Anthropic Claude, Cohere |
IBMî Graniteâ⢠is a flagship series of enterprise-grade models developed by IBM Researchî. These models feature an optimal mix of trust, performance, and cost-effectiveness attributes, enabling businesses to succeed in their generative AI initiatives.
Best Practices for Scaling Generative AI
To successfully scale generative AI, Gartner outlines 10 best practices:
Prioritize Use Cases: Continuously prioritize use cases aligned to the organization's AI ambition and measure business value.
Evaluate Build vs. Buy: Create a decision framework for build vs. buy, evaluating model training, security, integration, and pricing.
Plan for Scalability: Pilot use cases with an eye towards future scalability needs around data, privacy, security, etc.
Design Composable Architecture: Design a composable platform architecture to improve flexibility and avoid vendor lock-in.
Prioritize Responsible AI: Put responsible AI principles at the forefront across fairness, ethics, privacy, compliance, etc. Evaluate risk mitigation tools.
Invest in Data and AI Literacy: Invest in data and AI literacy programs across functions and leadership.
Instill Robust Data Engineering: Instill robust data engineering practices like knowledge graphs and vector embeddings.
Enable Human-AI Collaboration: Enable seamless human-AI collaboration with human-in-the-loop and communities of practice.
Apply FinOps Practices: Apply FinOps practices to monitor, audit, and optimize generative AI costs.
Adopt Agile Approach: Adopt an agile, product-centric approach with continuous updates based on user feedback.
Generative AI represents a fundamental shift in the services market, driven by its potential to perform complex reasoning, planning, and execution. As businesses harness these capabilities, they are discovering new ways to enhance customer interactions, transform service offerings, and streamline operations.