The banking and financial services industry is undergoing a significant transformation with the rise of artificial intelligence (AI). Large Language Models (LLMs) have shown immense potential in revolutionizing various aspects of finance, from customer service to investment analysis. However, traditional LLMs face challenges when it comes to providing accurate, timely, and trustworthy information in the fast-paced and highly regulated world of finance. This is where Retrieval-Augmented Generation (RAG) comes into play, promising to enhance AI capabilities in banking by combining the power of LLMs with context retrieval.
Understanding Retrieval-Augmented Generation (RAG)
Retrieval-Augmented Generation (RAG) is an innovative approach that integrates the strengths of LLMs with the ability to retrieve and incorporate relevant contextual information. RAG systems work by first processing a user's query and then retrieving pertinent information from a vast knowledge base. This retrieved context is then combined with the original query to generate a more accurate, comprehensive, and contextually relevant response.
The key advantages of RAG over traditional LLMs include:
Improved accuracy: By incorporating real-time, relevant information, RAG reduces the risk of generating outdated or incorrect responses.
Enhanced comprehensiveness: RAG enables AI systems to provide more detailed and nuanced answers by drawing from a wider range of contextual data.
Increased trustworthiness: The ability to trace and cite the sources of information used in generating responses enhances transparency and trust in AI-driven financial services.
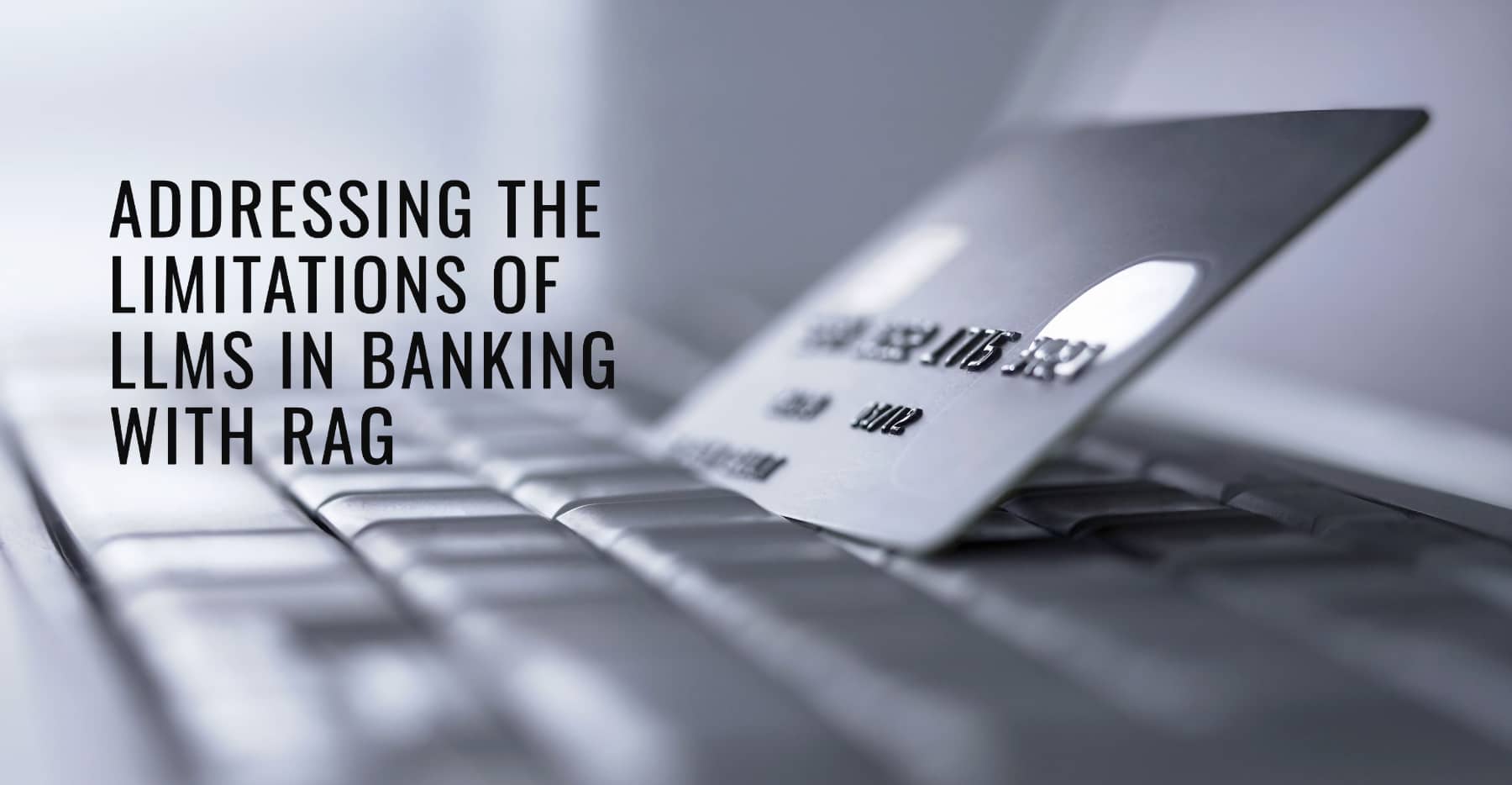
Addressing the Limitations of LLMs in Banking with RAG
Traditional LLMs face several limitations when applied to the banking and finance industry:
Hallucination: LLMs may generate fictitious or inconsistent information, which can lead to incorrect financial advisories and erode trust in AI-driven services.
Knowledge cut-off: LLMs are trained on static datasets and may not have access to the most recent information, resulting in outdated or irrelevant responses.
Lack of transparency: The "black box" nature of LLMs makes it difficult to understand the reasoning behind their outputs, which is crucial in the highly regulated financial sector.
RAG addresses these limitations by:
Reducing hallucination: By grounding responses in retrieved contextual information, RAG minimizes the risk of generating fictitious or inconsistent information.
Overcoming knowledge cut-off: RAG enables AI systems to access and incorporate the most up-to-date information, ensuring the timeliness and relevance of financial advisories.
Enhancing transparency: RAG's ability to cite the sources of information used in generating responses improves transparency and accountability in AI-driven financial services.
Key Advantages of RAG for Banking and Financial Services
The implementation of RAG in banking and financial services offers several key advantages:
Enhanced decision-making: RAG provides comprehensive and timely information, enabling financial professionals to make more informed and accurate decisions.
Improved accuracy and credibility: By incorporating relevant contextual information, RAG enhances the accuracy and credibility of financial analysis and advisories.
Continuous learning and flexibility: RAG allows AI systems to adapt to evolving financial landscapes by continuously updating their knowledge bases with the latest information.
Operational efficiencies and strategic advantages: RAG streamlines processes and provides valuable insights, leading to improved operational efficiency and competitive advantages in the financial industry.
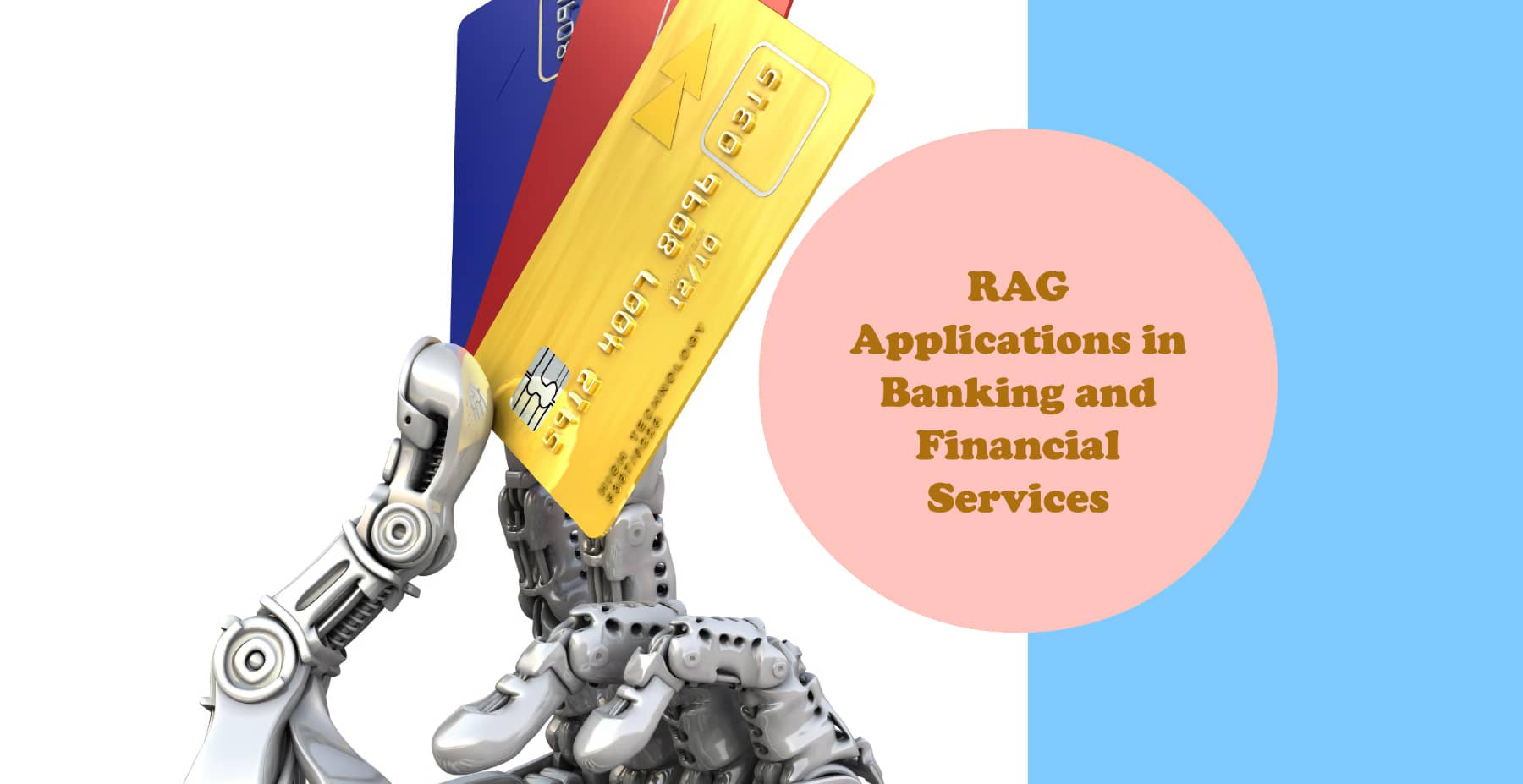
RAG Applications in Banking and Financial Services
RAG has numerous applications across various domains of banking and financial services:
Financial Sentiment Analysis
RAG can enhance financial sentiment analysis by incorporating real-time news, social media data, and market trends to provide more accurate and timely investment insights. By analyzing vast amounts of unstructured data and combining it with the power of LLMs, RAG enables AI systems to deliver more nuanced and contextually relevant sentiment analysis.
Private Company Screening
RAG can streamline the private company screening process by quickly identifying and summarizing relevant information from various sources, such as financial reports, news articles, and industry databases. This enables investors and financial institutions to make more informed decisions when evaluating potential investments or partnerships.
Know Your Customer (KYC) and Background Checks
RAG can significantly improve the efficiency and accuracy of Know Your Customer (KYC) and background checks by automating the retrieval and analysis of relevant information from multiple sources. By incorporating real-time data and ensuring compliance with the latest regulations, RAG helps financial institutions minimize risks and maintain trust in their services.
ESG and Sustainability Research
RAG can support ESG (Environmental, Social, and Governance) and sustainability research by providing comprehensive and up-to-date information on companies' ESG performance, risks, and opportunities. By integrating data from various sources, such as sustainability reports, news articles, and regulatory filings, RAG enables financial institutions to make more informed and responsible investment decisions.
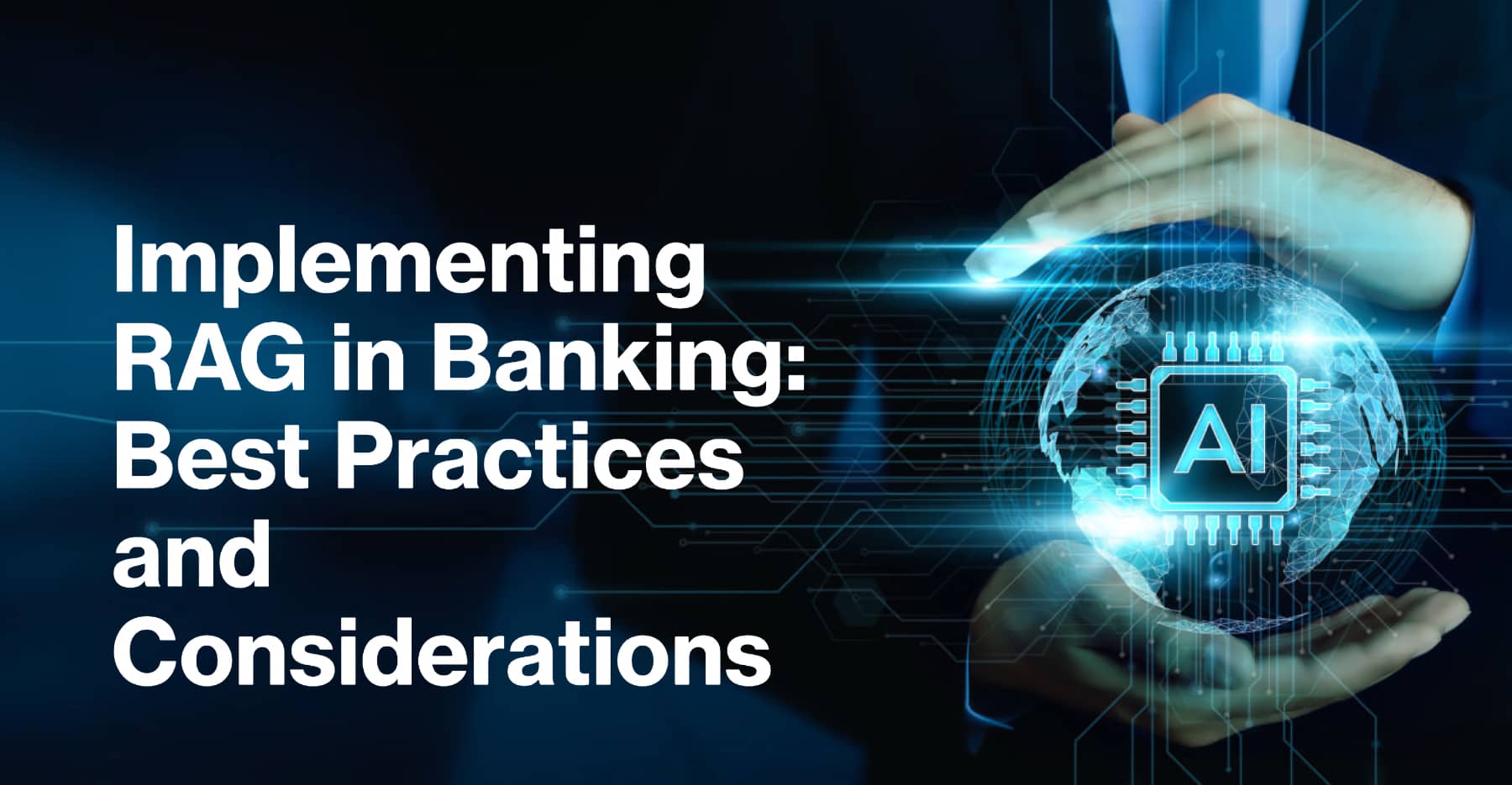
Implementing RAG in Banking: Best Practices and Considerations
To successfully implement RAG in banking and financial services, organizations should consider the following best practices:
Choosing the right LLMs and datasets: Select LLMs and datasets that are well-suited for financial applications, taking into account factors such as domain-specific knowledge and performance on financial tasks.
Instruction tuning: Fine-tune RAG models using instruction-following datasets to align their outputs with the specific requirements and conventions of the banking industry.
Integration with existing workflows: Seamlessly integrate RAG into existing banking workflows and systems to ensure smooth adoption and maximize its benefits.
Continuous monitoring and updating: Regularly monitor and update RAG models to ensure they remain accurate, relevant, and aligned with the latest industry developments and regulations.
Best Practice | Description |
Choosing the right LLMs and datasets | Select LLMs and datasets well-suited for financial applications, considering domain-specific knowledge and performance |
Instruction tuning | Fine-tune RAG models using instruction-following datasets to align outputs with banking requirements |
Integration with existing workflows | Seamlessly integrate RAG into existing banking workflows and systems for smooth adoption and maximum benefits |
Continuous monitoring and updating | Regularly monitor and update RAG models to ensure accuracy, relevance, and alignment with industry developments |
Real-World Examples of RAG in Banking
Let's explore some real-world examples of how RAG can be applied in banking and financial services:
Case Study 1: Enhancing Financial Advisories with RAG
A leading investment bank implements RAG to enhance its financial advisory services. By integrating real-time market data, news, and analyst reports, the RAG-powered system provides clients with more accurate, timely, and comprehensive investment recommendations. This leads to increased client satisfaction, higher assets under management, and a competitive edge in the market.
Case Study 2: Improving Private Company Screening Efficiency with RAG
A venture capital firm adopts RAG to streamline its private company screening process. The RAG system automatically retrieves and analyzes relevant information from various sources, including financial reports, news articles, and industry databases. This enables the firm to quickly identify promising investment opportunities, conduct thorough due diligence, and make more informed investment decisions.
Case Study 3: Streamlining KYC Processes with RAG
A global bank implements RAG to enhance its Know Your Customer (KYC) processes. By leveraging RAG's ability to retrieve and analyze data from multiple sources in real-time, the bank automates and accelerates its customer onboarding and risk assessment procedures. This results in improved compliance, reduced operational costs, and a better customer experience.
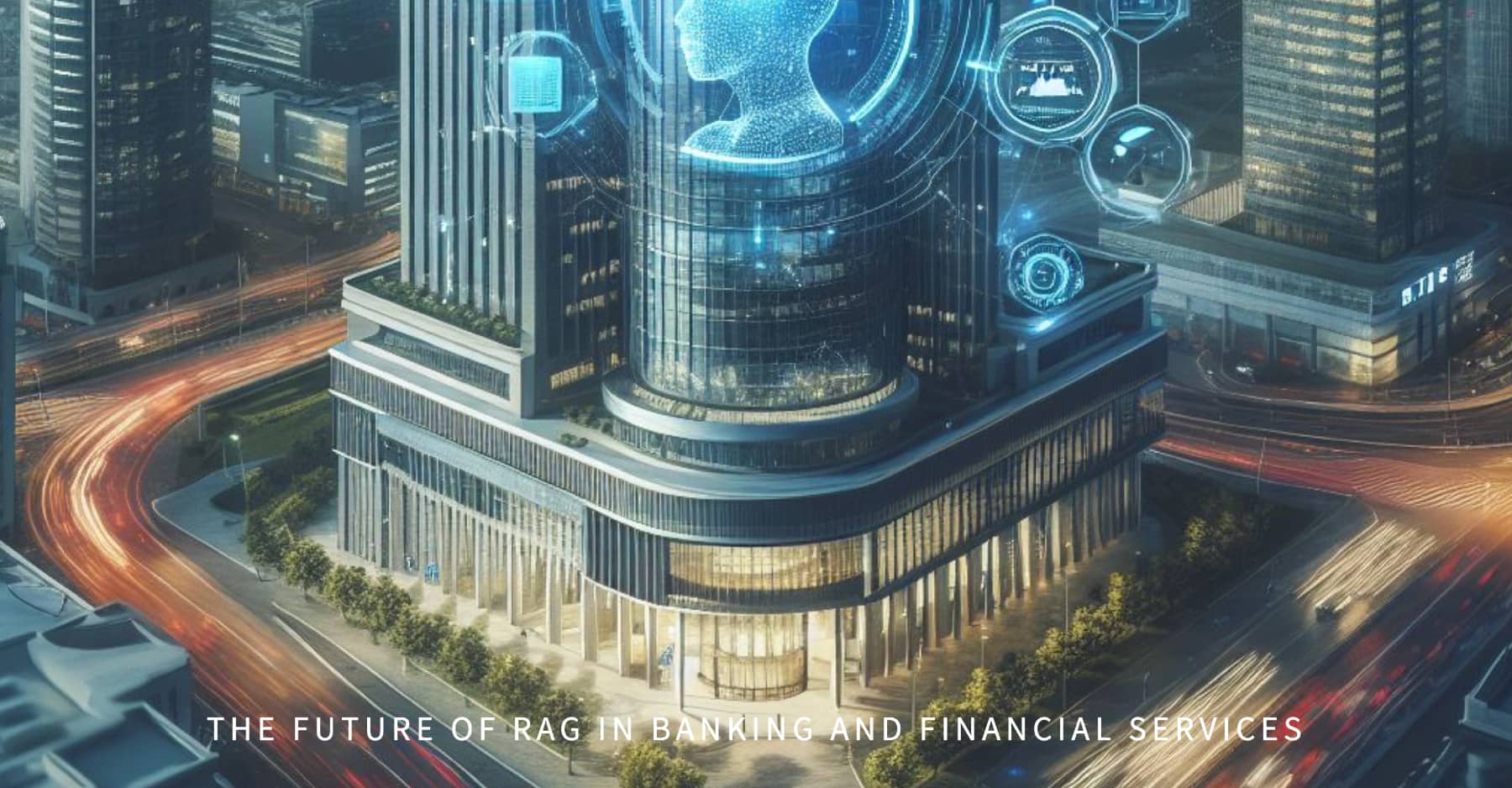
The Future of RAG in Banking and Financial Services
As AI continues to evolve, the future of RAG in banking and financial services looks promising:
Emerging trends and innovations: RAG will likely benefit from advancements in natural language processing, knowledge representation, and retrieval techniques, further enhancing its capabilities and performance.
Potential impact on the banking industry: The widespread adoption of RAG has the potential to transform the banking landscape by enabling more accurate, efficient, and trustworthy financial services, ultimately benefiting both financial institutions and their clients.
Preparing for the future: To stay competitive in the AI-driven banking landscape, financial institutions must embrace RAG and other cutting-edge technologies, investing in talent, infrastructure, and partnerships to harness their full potential.
Conclusion
Retrieval-Augmented Generation (RAG) represents a significant leap forward in the application of AI in banking and financial services. By addressing the limitations of traditional Large Language Models (LLMs) and incorporating contextual information retrieval, RAG enables more accurate, comprehensive, and trustworthy AI-driven financial services.
The benefits of RAG in banking are numerous, from enhancing decision-making and improving the accuracy of financial analysis to streamlining processes and driving operational efficiencies. As the financial industry continues to evolve, adopting RAG and other innovative AI technologies will be crucial for staying competitive and meeting the growing demands of customers and regulators alike.
Financial institutions that embrace RAG and invest in its implementation will be well-positioned to reap the benefits of this transformative technology, delivering more accurate, timely, and trustworthy services to their clients. The future of banking lies in the successful integration of AI, and Retrieval-Augmented Generation is poised to play a pivotal role in shaping this exciting new era of financial services.