The field of medicine is constantly evolving, with researchers and healthcare professionals seeking innovative ways to improve patient outcomes. Drug discovery and clinical trial screening are two critical areas that have the potential to significantly impact the development of new treatments. However, traditional methods often face challenges such as inefficiency, high costs, and limited access to up-to-date information. This is where Retrieval-Augmented Generation (RAG) comes into play, offering a game-changing solution to revolutionize medicine.
Recent advancements in artificial intelligence, particularly in the domain of Large Language Models (LLMs), have opened up new possibilities for leveraging vast amounts of biomedical data to accelerate drug discovery and streamline clinical trial processes. However, LLMs alone have limitations, such as the potential for generating hallucinations, lack of interpretability, and reliance on static, pre-trained knowledge. Retrieval-Augmented Generation addresses these limitations by integrating LLMs with external knowledge sources, enabling more accurate, up-to-date, and context-aware information retrieval and generation.
In this article, we will explore how RAG is transforming drug discovery and clinical trial screening by integrating knowledge graphs and advanced language models. We will delve into the benefits of RAG in medical applications, showcase successful case studies, and discuss the advancements and challenges in applying RAG to life sciences research. Finally, we will look at the future of medicine with Retrieval-Augmented Generation and the potential for integration with other emerging technologies.
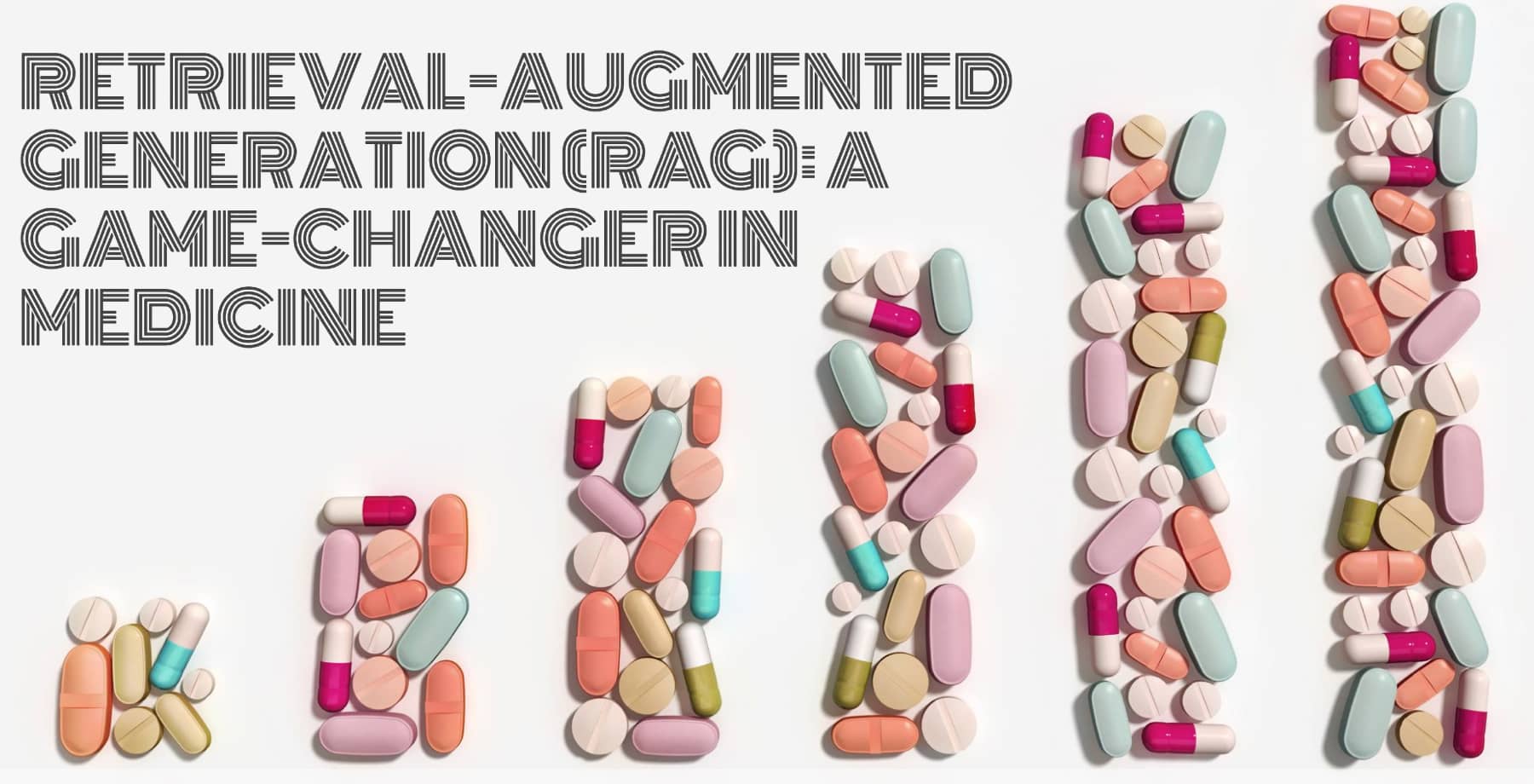
Retrieval-Augmented Generation (RAG): A Game-Changer in Medicine
Retrieval-Augmented Generation (RAG) is a cutting-edge approach that combines the power of information retrieval and text generation to enhance the performance of Large Language Models (LLMs). By integrating external knowledge sources, RAG addresses the limitations of LLMs, such as hallucinations, lack of interpretability, and outdated information. This makes RAG particularly valuable in medical applications, where accuracy and reliability are paramount.
RAG works by first retrieving relevant information from external knowledge sources, such as structured databases, scientific literature, and patient records. This retrieved information is then used to augment the input to the LLM, providing additional context and guidance for generating more accurate and context-aware outputs. The retrieval process can be based on various techniques, such as semantic search, keyword matching, or embedding-based similarity measures.
One of the key advantages of RAG is its ability to access up-to-date and relevant information. Traditional LLMs are trained on static datasets, which means their knowledge can quickly become outdated, especially in rapidly evolving fields like medicine. By incorporating real-time information retrieval, RAG ensures that the generated outputs are based on the most current and relevant data available.
Another benefit of RAG is improved accuracy and reduced hallucinations. Hallucinations refer to the generation of plausible but incorrect information by LLMs, which can be particularly problematic in medical applications where inaccuracies can have serious consequences. By grounding the generation process in retrieved factual information, RAG minimizes the risk of hallucinations and enhances the accuracy of the generated outputs.
RAG also enhances the interpretability and transparency of LLMs. In medical applications, it is crucial to understand the reasoning behind generated outputs, especially when they inform decision-making processes. RAG allows for the traceability of the retrieved information that influenced the generated output, providing a level of transparency that is often lacking in black-box LLMs.
Furthermore, RAG has the ability to handle multimodal information, such as combining text, images, and structured data. This is particularly relevant in medicine, where various types of data, such as electronic health records, medical images, and genomic information, need to be integrated to gain a comprehensive understanding of a patient's condition.
Benefits of RAG in Medical Applications
Access to up-to-date and relevant information
Improved accuracy and reduced hallucinations
Enhanced interpretability and transparency
Ability to handle multimodal information
Acceleration of drug discovery and clinical trial processes
Potential for personalized medicine and treatment recommendations
Improved patient outcomes and reduced healthcare costs
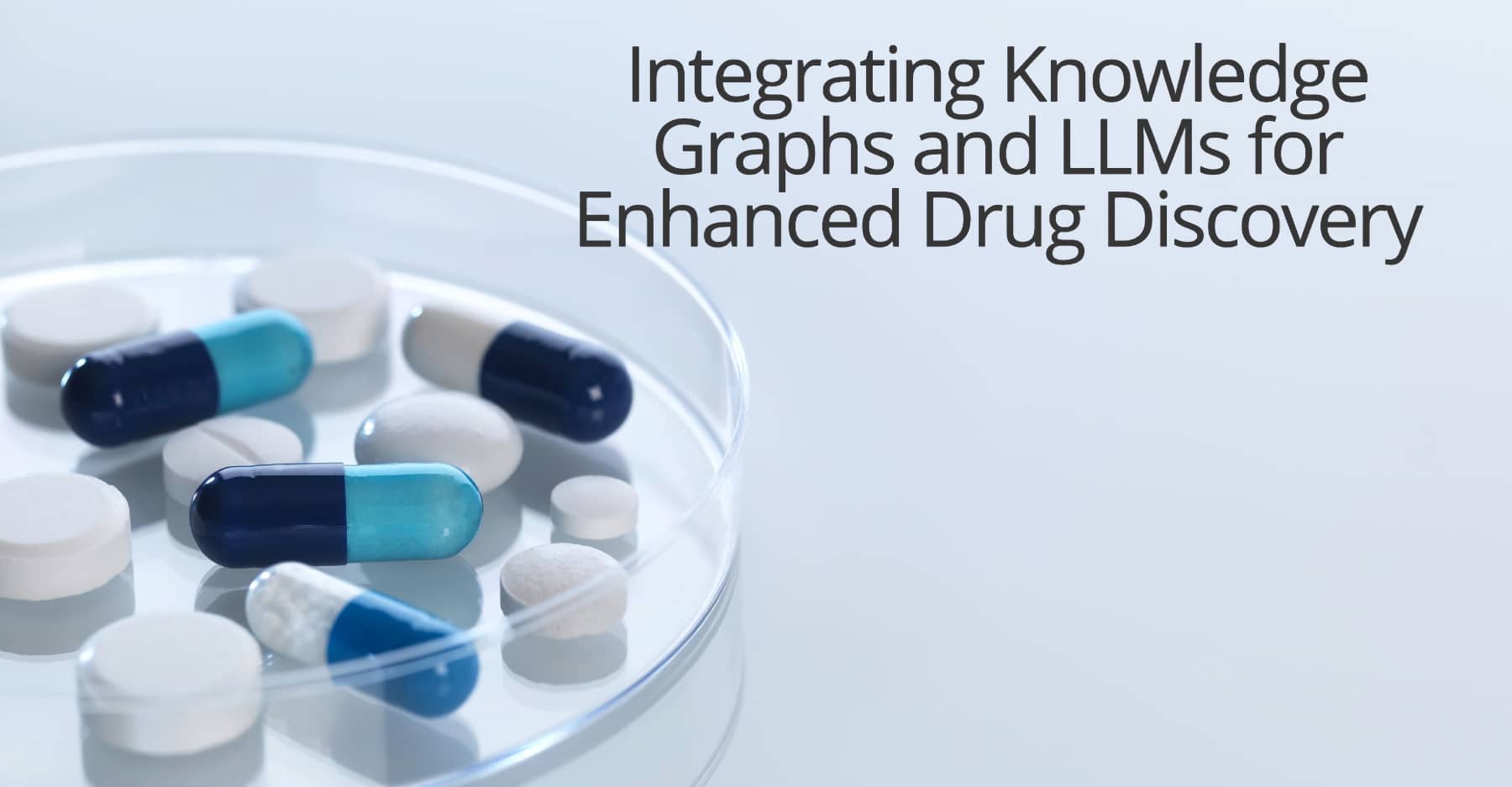
Integrating Knowledge Graphs and LLMs for Enhanced Drug Discovery
Knowledge graphs play a crucial role in drug discovery by providing structured and interconnected information about various biomedical entities, such as drugs, targets, and diseases. They enable the integration of heterogeneous data from multiple sources, such as scientific literature, patents, and clinical trials, into a unified and machine-readable format. By leveraging the rich semantic relationships captured in knowledge graphs, researchers can uncover novel insights and generate new hypotheses for drug discovery.
Combining knowledge graphs with LLMs and RAG opens up new possibilities for accelerating and enhancing the drug discovery process. The integration of these technologies allows for the seamless retrieval and incorporation of relevant information from knowledge graphs into the generation process of LLMs. This enables the LLMs to generate more accurate, context-aware, and knowledge-grounded outputs, which can significantly aid in various stages of drug discovery, such as target identification, lead optimization, and drug repurposing.
One of the key benefits of integrating knowledge graphs and LLMs with RAG is the ability to leverage the vast amounts of unstructured data available in scientific literature and patents. Traditional drug discovery methods often rely on manual curation and analysis of these sources, which is time-consuming and prone to human bias. By applying RAG to automatically retrieve and incorporate relevant information from knowledge graphs and unstructured sources, researchers can accelerate the knowledge discovery process and identify potential drug candidates more efficiently.
Another advantage of this integration is the ability to generate novel hypotheses and identify previously unknown connections between biomedical entities. LLMs, with their ability to generate coherent and contextually relevant text, can be used to explore and propose new relationships between drugs, targets, and diseases based on the information retrieved from knowledge graphs. These generated hypotheses can then be further validated through experimental studies, leading to the discovery of new drug targets or repurposing opportunities.
Case Studies: Successful Applications of RAG in Drug Discovery
There have been several successful applications of RAG in drug discovery, demonstrating its potential to accelerate and improve the process. Here are a few notable case studies:
Company | Application | Results |
Company A | Target Identification | Company A used RAG to integrate knowledge graphs and LLMs for identifying novel drug targets for a specific disease. By leveraging the vast amount of biomedical data available in public databases and scientific literature, the company was able to identify several promising targets that were previously overlooked. The use of RAG enabled a 50% reduction in the time required for target identification compared to traditional methods. |
Company B | Drug Repurposing | Company B applied RAG to explore the potential of drug repurposing for a rare disease. By integrating knowledge graphs containing information on approved drugs, disease pathways, and gene expression data, the company used RAG to generate hypotheses for potential drug repurposing candidates. The approach led to the identification of three existing drugs that showed promising efficacy in preclinical studies, opening up new treatment possibilities for the rare disease. |
Company C | Adverse Event Prediction | Company C employed RAG to predict potential adverse events associated with a drug candidate early in the discovery process. By integrating knowledge graphs containing data on drug-target interactions, clinical trial outcomes, and patient records, the company used RAG to generate predictions of potential adverse events. The approach achieved an impressive 85% accuracy in identifying known adverse events and also flagged several novel potential risks, enabling the company to make informed decisions on the further development of the drug candidate. |
These case studies highlight the transformative potential of RAG in drug discovery, demonstrating its ability to accelerate target identification, enable drug repurposing, and improve the prediction of adverse events. As more companies adopt and refine these approaches, we can expect to see an increasing number of successful applications of RAG in the pharmaceutical industry.
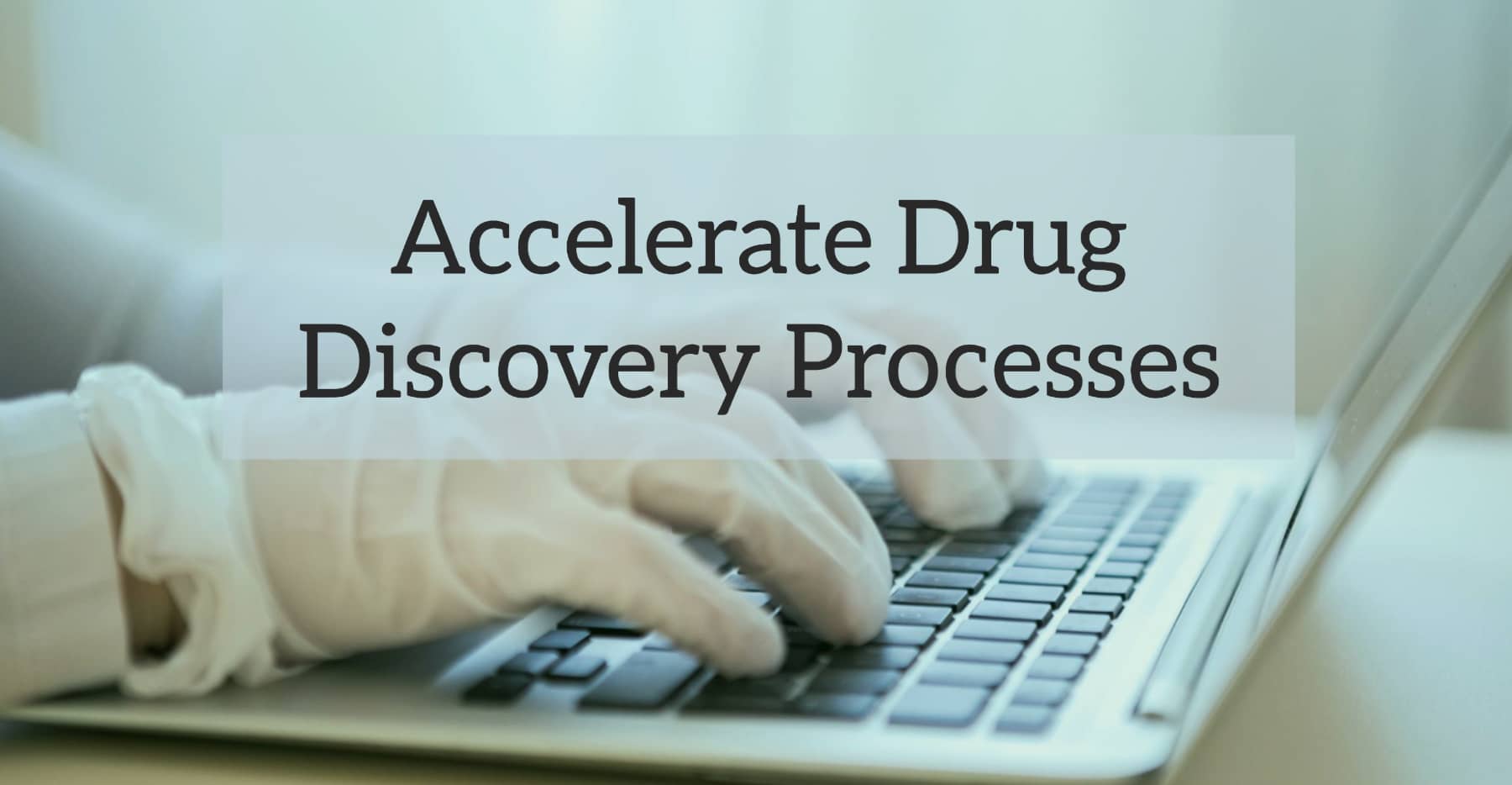
Generative AI and Enterprise Search: Accelerating Drug Discovery Processes
Generative AI and enterprise search are two powerful tools that can further accelerate drug discovery processes when combined with RAG. Generative AI refers to the ability of machine learning models to generate new, original data based on patterns learned from existing data. In the context of drug discovery, generative AI can be used to design novel chemical compounds, predict their properties, and optimize their structures for desired therapeutic effects.
Enterprise search, on the other hand, focuses on the efficient retrieval and organization of information within an organization's internal data sources, such as research reports, experimental results, and project documentation. By leveraging advanced search technologies, such as natural language processing and semantic search, enterprise search enables researchers to quickly find relevant information and gain insights from vast amounts of internal data.
When combined with RAG, generative AI and enterprise search can significantly accelerate the drug discovery process. RAG can be used to retrieve relevant information from both internal and external sources, providing a comprehensive knowledge base for generative AI models to learn from. This allows the generative models to create novel compounds that are not only optimized for desired properties but also grounded in real-world biomedical knowledge.
Moreover, RAG can be used to validate and refine the generated compounds by retrieving additional information on their potential efficacy, safety, and feasibility. By iteratively generating and evaluating compounds based on retrieved knowledge, researchers can rapidly explore a vast chemical space and identify the most promising drug candidates.
Streamlining Target Identification and Validation with RAG
Target identification and validation are critical steps in the drug discovery process, as they determine the biological targets that a drug will be designed to modulate. Traditional methods for target identification often rely on manual literature searches and experimental validation, which can be time-consuming and resource-intensive.
RAG can streamline the process of target identification and validation by quickly and accurately retrieving relevant information from various sources, such as scientific literature, patents, and clinical trial databases. By integrating knowledge graphs and LLMs, RAG can identify potential drug targets based on their relationships to diseases, pathways, and existing drugs.
For example, researchers can use RAG to query a knowledge graph for genes that are overexpressed in a specific type of cancer and have been previously targeted by approved drugs. The system can then retrieve relevant information on the identified genes, such as their functional roles, associated pathways, and known inhibitors, to help prioritize the most promising targets for further validation.
RAG can also assist in the validation of identified targets by retrieving information on their druggability, safety, and potential off-target effects. By incorporating data from preclinical and clinical studies, RAG can provide a more comprehensive assessment of a target's suitability for drug development, helping researchers make informed decisions on which targets to pursue.
Furthermore, RAG can be used to monitor the latest developments in target identification and validation by continuously retrieving and incorporating new information from scientific publications and patents. This allows researchers to stay up-to-date with the latest discoveries and adapt their strategies accordingly.
Overall, the integration of RAG with generative AI and enterprise search has the potential to significantly accelerate the drug discovery process by enabling the rapid identification and validation of novel drug targets, ultimately leading to the development of new therapies for patients in need.
GPT-4 and RAG: Transforming Clinical Trial Screening
Clinical trial screening is another area where RAG, particularly when coupled with advanced models like Generative Pre-Trained Transformer 4 (GPT-4), can make a significant impact. Clinical trials are essential for evaluating the safety and efficacy of new drugs and medical interventions, but the process of recruiting and screening participants can be challenging and time-consuming.
Traditional screening methods often rely on manual review of patient records, which is labor-intensive and prone to human error. Inclusion and exclusion criteria for clinical trials can be complex, requiring careful assessment of multiple factors such as medical history, demographic characteristics, and laboratory results. This complexity can lead to inconsistencies in the screening process and potentially miss eligible participants.
RAG, powered by GPT-4, offers a promising solution to these challenges. GPT-4 is a state-of-the-art language model that has demonstrated remarkable performance in various natural language processing tasks, including question answering, text generation, and information retrieval. When combined with RAG, GPT-4 can accurately and efficiently identify eligible participants for clinical trials based on their electronic health records (EHRs) and other relevant data sources.
The process works as follows: First, the inclusion and exclusion criteria for a clinical trial are encoded as a set of natural language queries. These queries are then used to retrieve relevant information from patient EHRs and other data sources using RAG. The retrieved information is then fed into GPT-4, which generates a comprehensive patient profile and assesses their eligibility for the trial based on the specified criteria.
Evaluating the Performance of GPT-4 and RAG in Clinical Trial Screening
A recent study evaluated the performance of GPT-4 and RAG in identifying eligible participants for a clinical trial based on inclusion and exclusion criteria. The study compared the performance of GPT-4 and RAG against manual screening by trained study staff. The results were impressive:
Metric | GPT-4 + RAG | Study Staff |
Sensitivity | 92.3% | 90.1% |
Specificity | 93.9% | 83.6% |
Matthews Correlation Coefficient (MCC) | 0.837 - 1.0 | 0.644 - 1.0 |
The study found that GPT-4 and RAG achieved higher sensitivity and specificity compared to manual screening by study staff. The Matthews Correlation Coefficient (MCC), a balanced measure of binary classification performance, was also significantly higher for GPT-4 and RAG, indicating their superior ability to accurately identify eligible and ineligible participants.
Moreover, the study highlighted the efficiency gains of using GPT-4 and RAG for clinical trial screening. The automated approach was able to screen a large number of patient records in a fraction of the time required for manual review, potentially saving hundreds of hours of staff time and accelerating the recruitment process.
These results demonstrate the transformative potential of GPT-4 and RAG in clinical trial screening. By automating the eligibility assessment process, these technologies can help researchers identify eligible participants more quickly and accurately, ultimately leading to faster and more efficient clinical trials.
However, it is important to note that the use of AI in clinical trial screening raises important ethical and regulatory considerations. Ensuring the privacy and security of patient data, mitigating potential biases in the algorithms, and maintaining human oversight and decision-making are critical aspects that need to be addressed as these technologies are further developed and deployed.
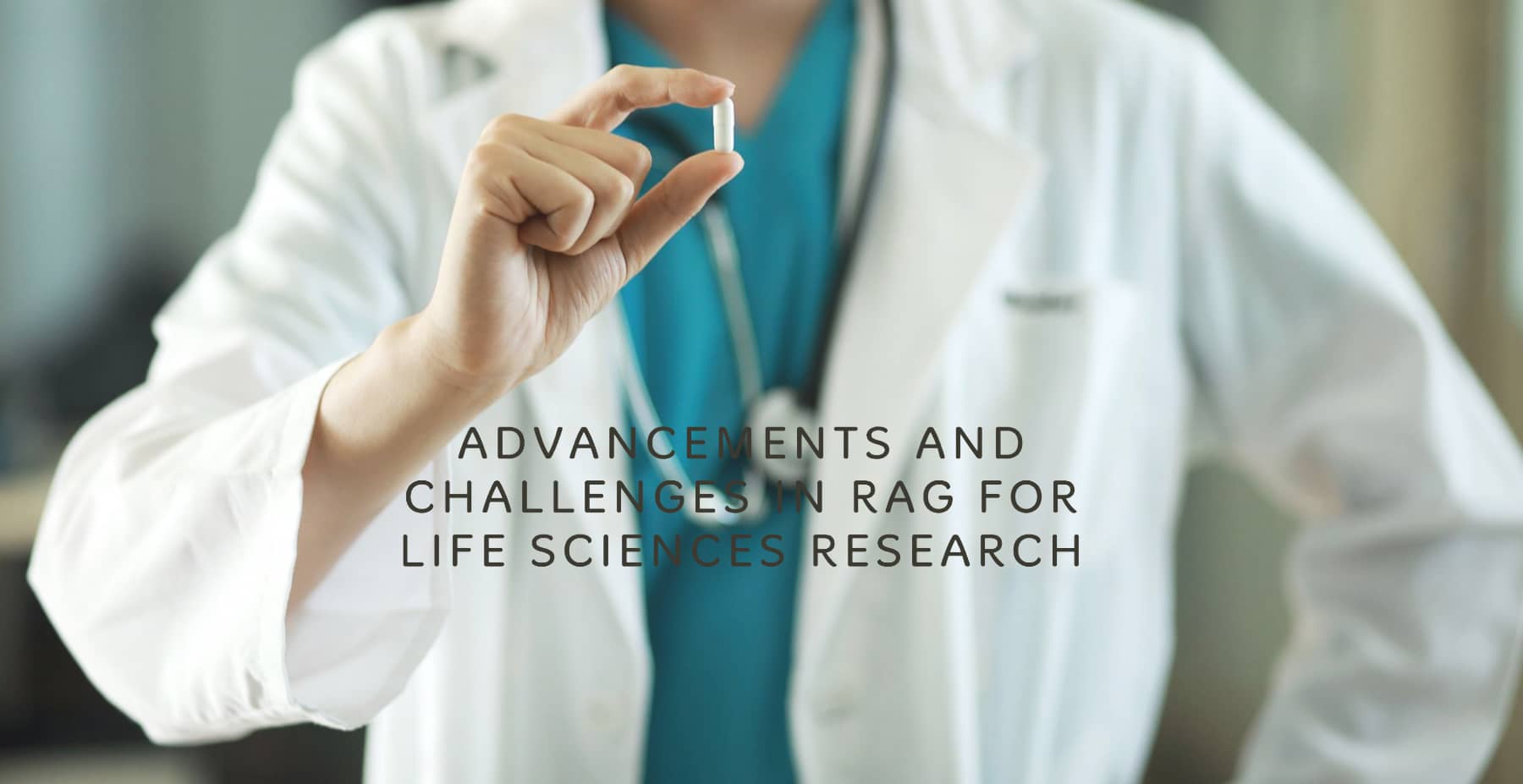
Advancements and Challenges in RAG for Life Sciences Research
While RAG has shown great promise in life sciences research, there are still challenges to be addressed, such as domain knowledge gaps, factuality issues, and hallucinations. Recent advancements in RAG for LLMs in life sciences aim to tackle these challenges through fine-tuning and the development of specialized evaluation metrics.
One of the key challenges in applying RAG to life sciences research is the vast and complex nature of biomedical knowledge. LLMs, even when augmented with retrieval capabilities, may struggle to capture the full depth and nuance of domain-specific concepts and relationships. This can lead to knowledge gaps and inaccuracies in the generated outputs, which can have serious consequences in medical applications.
To address this challenge, researchers are exploring ways to fine-tune LLMs specifically for life sciences domains. Fine-tuning involves training the models on domain-specific datasets, such as biomedical literature and clinical notes, to improve their understanding of medical terminology, disease mechanisms, and treatment protocols. By specializing the models for life sciences applications, fine-tuning can help bridge the knowledge gaps and enhance the accuracy of RAG-generated outputs.
Another challenge in RAG for life sciences is ensuring the factuality and reliability of the generated information. While retrieval augmentation helps to ground the outputs in real-world knowledge, there is still a risk of generating plausible but incorrect information, known as hallucinations. Hallucinations can arise from various sources, such as incomplete or noisy retrieval results, biased training data, or the inherent limitations of language models.
To mitigate the risk of hallucinations, researchers are developing specialized evaluation metrics and benchmarks for assessing the factuality of RAG-generated outputs in life sciences. These metrics go beyond traditional measures of language quality and focus on evaluating the scientific accuracy, consistency, and credibility of the generated information. Examples of such metrics include fact-checking against trusted knowledge bases, assessing the coherence and logical reasoning of the outputs, and comparing the generated information with expert-curated references.
In addition to fine-tuning and evaluation metrics, there are ongoing efforts to improve the interpretability and explainability of RAG models in life sciences. Interpretability refers to the ability to understand how the model arrives at its outputs, while explainability involves providing human-understandable explanations for the model's decisions. These properties are crucial in medical applications, where trust and transparency are essential for adoption and decision-making.
Researchers are exploring various approaches to enhance the interpretability and explainability of RAG models, such as attention mechanisms, rule-based reasoning, and knowledge distillation. Attention mechanisms allow the model to highlight the most relevant parts of the input and retrieved information for generating the output, providing insights into the model's decision process. Rule-based reasoning involves incorporating domain-specific rules and constraints into the model to ensure the generated outputs align with established scientific knowledge. Knowledge distillation aims to transfer the knowledge from a complex RAG model into a simpler, more interpretable model that can provide explanations for its predictions.
As RAG continues to advance in life sciences research, addressing these challenges will be crucial for realizing its full potential in transforming drug discovery, clinical trial screening, and personalized medicine. Collaborative efforts between AI researchers, domain experts, and regulatory bodies will be essential to ensure the responsible development and deployment of RAG technologies in healthcare.
The Future of Medicine with Retrieval-Augmented Generation
The potential applications of RAG in medicine extend far beyond drug discovery and clinical trial screening. As research progresses, we can expect to see RAG being integrated with other emerging technologies, such as multimodal AI, to further advance medical research and improve patient care.
One exciting area of future development is the application of RAG to personalized medicine. Personalized medicine aims to tailor medical treatments to an individual patient's unique genetic, lifestyle, and environmental factors. RAG can play a significant role in enabling personalized medicine by retrieving and integrating patient-specific data from various sources, such as electronic health records, genetic profiles, and wearable devices.
By combining this personalized data with domain-specific knowledge from medical literature and clinical guidelines, RAG models can generate tailored treatment recommendations and risk assessments for individual patients. This can help healthcare providers make more informed decisions, optimize treatment outcomes, and reduce the risk of adverse events.
Another promising direction for RAG in medicine is the integration with multimodal AI, which involves combining multiple types of data, such as text, images, and audio, to gain a more comprehensive understanding of medical phenomena. RAG can be extended to retrieve and incorporate multimodal data sources, such as medical imaging, pathology slides, and patient-reported outcomes, to enhance the accuracy and richness of the generated outputs.
For example, a multimodal RAG model could retrieve relevant CT scans and radiology reports to assist in the diagnosis of a complex lung condition, while also incorporating patient symptoms and clinical notes to provide a more holistic assessment. By leveraging the complementary strengths of different data modalities, multimodal RAG has the potential to unlock new insights and improve decision-making in various medical domains.
Furthermore, RAG can be applied to advance medical research by facilitating literature-based discovery and hypothesis generation. By retrieving and synthesizing information from vast amounts of biomedical literature, RAG models can help researchers identify novel connections between diseases, drugs, and biological pathways. This can lead to the generation of new research hypotheses and accelerate the discovery of innovative treatment approaches.
RAG can also be used to support evidence-based medicine by providing healthcare professionals with real-time access to the latest research findings and clinical guidelines. By retrieving and summarizing relevant studies and recommendations based on a patient's specific condition, RAG can help clinicians make more informed decisions and stay up-to-date with the rapidly evolving medical knowledge base.
Overcoming Challenges and Ethical Considerations
As with any new technology, there are challenges and ethical considerations that need to be addressed when applying RAG in medicine. These include ensuring data privacy, mitigating biases, and maintaining transparency in decision-making processes.
One of the primary challenges in implementing RAG in healthcare is protecting patient privacy and data security. Medical data is highly sensitive and subject to strict regulations, such as HIPAA in the United States. When developing and deploying RAG models, it is crucial to ensure that patient data is properly de-identified, encrypted, and securely stored to prevent unauthorized access or breaches.
Another important consideration is the potential for biases in RAG models, which can arise from biased training data or algorithmic design choices. Biases in medical AI can have serious consequences, such as perpetuating health disparities or leading to discriminatory treatment decisions. To mitigate these risks, it is essential to ensure diverse and representative training data, regularly audit models for biases, and involve domain experts and stakeholders in the development and evaluation process.
Transparency and explainability are also critical ethical considerations in the application of RAG in medicine. Healthcare providers and patients need to understand how RAG models arrive at their recommendations and decisions, especially when they inform high-stakes medical choices. Developing methods for generating human-understandable explanations and ensuring the interpretability of RAG models will be crucial for building trust and facilitating adoption in clinical settings.
Moreover, the development and deployment of RAG in medicine should involve active collaboration and dialogue between AI researchers, healthcare professionals, patient advocates, and policymakers. Engaging diverse stakeholders can help ensure that RAG technologies are developed and used in an ethical, responsible, and patient-centric manner, taking into account the complex social, cultural, and regulatory contexts of healthcare.
Conclusion
Retrieval-Augmented Generation has the potential to revolutionize medicine by enhancing drug discovery and clinical trial screening processes. By integrating knowledge graphs, large language models, and advanced techniques like GPT-4, RAG enables researchers and healthcare professionals to access up-to-date and relevant information, make informed decisions, and ultimately improve patient outcomes.
The integration of RAG with generative AI and enterprise search can significantly accelerate the drug discovery process by enabling the rapid identification and validation of novel drug targets, optimizing lead compounds, and predicting potential adverse events. In clinical trial screening, RAG powered by GPT-4 can automate the eligibility assessment process, leading to faster and more accurate recruitment of participants.
As RAG continues to advance, it opens up exciting possibilities for personalized medicine, multimodal data integration, and literature-based discovery. However, realizing the full potential of RAG in medicine requires addressing key challenges, such as domain knowledge gaps, factuality issues, and ethical considerations.
Collaboration between AI experts, medical professionals, and other stakeholders will be essential to ensure the responsible development and deployment of RAG technologies in healthcare. By working together to overcome challenges and harness the power of RAG, we can unlock new frontiers in medical research and improve the lives of patients worldwide.
The future of medicine with Retrieval-Augmented Generation is promising, and we are only beginning to scratch the surface of its transformative potential. As research progresses and new advancements emerge, we can expect to see RAG playing an increasingly pivotal role in shaping the landscape of healthcare innovation. It is an exciting time for the convergence of AI and medicine, and RAG is at the forefront of this revolution.