In today's fast-paced digital landscape, the growing demand for efficient and accurate enterprise search has become a critical factor for businesses across all industries. As consumers become accustomed to the convenience of "on-demand" experiences and instantaneous results, the pressure on organizations to deliver relevant information quickly and precisely has never been greater. This is where Retrieval-Augmented Generation (RAG) comes into play, offering a groundbreaking solution to transform enterprise search strategies.
The Need for Enhanced Search Capabilities
Traditional keyword-based searches often fall short in providing comprehensive and relevant results, leading to frustration and reduced productivity among users. Organizations are increasingly recognizing the need for more sophisticated search methods that can understand context, deliver accurate information, and maximize the value of their data assets.
Introducing Retrieval-Augmented Generation (RAG)
Retrieval-Augmented Generation (RAG) is an innovative approach that combines the power of information retrieval with the skill of natural language generation. By leveraging advanced retrieval models and generative models, RAG enables enterprises to retrieve relevant data from vast repositories and generate contextualized responses, bridging the gap between information retrieval and human understanding.
The Transformative Power of RAG
The impact of RAG on enterprise search strategies is far-reaching and transformative. By harnessing the capabilities of RAG, organizations can:
Benefit | Description |
Improve Search Accuracy | RAG enhances search relevance and precision by understanding context and delivering more accurate results. |
Maximize Resources | RAG automates complex search processes, reduces manual effort, and optimizes resource allocation, leading to cost savings and improved operational efficiency. |
Enhance Decision-Making | RAG-powered insights from extensive data sets enable informed, data-driven decision-making, promoting agility and adaptability in dynamic business environments. |
Drive ROI | Implementing RAG can yield significant returns on investment through improved search accuracy, reduced search time, and lower operational costs. |
Understanding Retrieval-Augmented Generation (RAG)
To fully grasp the transformative potential of Retrieval-Augmented Generation (RAG) in enterprise search strategies, it is essential to understand its core principles and how it differs from traditional search methods.
The Core Principles of RAG
RAG is built upon two fundamental components: retrieval models and generative models. These components work in harmony to deliver a powerful and efficient search experience.
Retrieval Models: Retrieval models are responsible for finding relevant information within large data repositories. They utilize sophisticated algorithms to identify and extract the most pertinent data based on the user's query.
Generative Models: Generative models, often powered by advanced Large Language Models (LLMs), take the retrieved information and generate human-like, contextualized responses. These models possess the ability to understand the nuances of language and provide coherent, meaningful outputs.
The RAG Process: Combining Information Retrieval and Natural Language Generation
RAG seamlessly integrates information retrieval and natural language generation to deliver a superior search experience. The process can be summarized as follows:
A user submits a query to the RAG system.
The retrieval model searches through the enterprise's data repositories, identifying the most relevant information based on the query.
The retrieved information is then passed to the generative model.
The generative model analyzes the retrieved data, understands its context, and generates a human-like response that directly addresses the user's query.
The generated response is returned to the user, providing them with accurate and contextually relevant information.
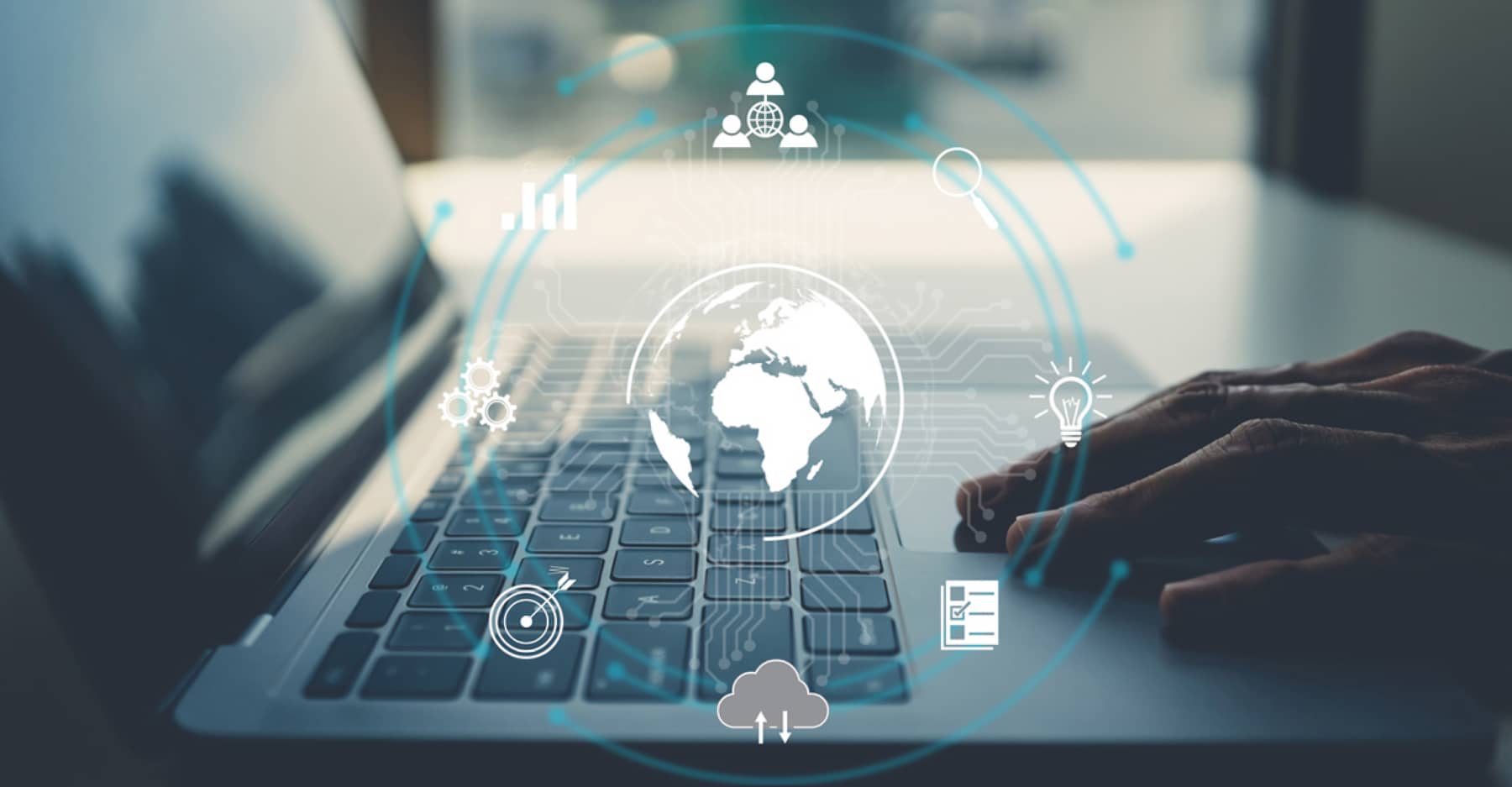
Advantages of RAG over Traditional Search Methods
RAG offers several key advantages over traditional search methods, making it a superior choice for enterprises looking to optimize their search strategies.
Traditional Search | RAG |
Keyword-based matching | Contextual understanding |
Limited relevance | High relevance and precision |
Static results | Dynamic, generated responses |
Inefficient resource utilization | Optimized resource allocation |
Lacks adaptability | Adaptable to new information |
By leveraging the power of retrieval models and generative models, RAG enables enterprises to overcome the limitations of traditional search methods. It delivers search relevance, search precision, and adaptability, ensuring that users receive the most accurate and up-to-date information in response to their queries.
RAG's Impact on Search Relevance and Precision
One of the most significant advantages of Retrieval-Augmented Generation (RAG) is its ability to transform search relevance and precision. By leveraging advanced retrieval models and generative models, RAG enables enterprises to deliver highly accurate and contextually relevant search results to their users.
Enhancing Search Accuracy through Contextual Understanding
Traditional keyword-based searches often struggle to capture the true intent behind a user's query, leading to irrelevant or incomplete results. RAG addresses this challenge by employing contextual understanding to interpret the meaning and context of search queries.
Through the use of Large Language Models (LLMs) and sophisticated natural language processing (NLP) techniques, RAG can analyze the nuances of language and understand the user's intent. This contextual understanding allows the system to refine search queries and retrieve the most relevant information from the enterprise's data repositories.
Improving the User Experience with More Relevant Results
The enhanced search relevance and precision achieved through RAG directly translate to an improved user experience. When users receive accurate and contextually relevant search results, they can quickly find the information they need, without having to sift through irrelevant or outdated content.
This improved user experience has far-reaching benefits for enterprises:
Increased user satisfaction and engagement
Reduced time spent searching for information
Enhanced productivity and efficiency
Greater trust in the search system and the information it provides
Case Studies Demonstrating RAG's Impact on Search Relevance
Several organizations have already witnessed the transformative power of RAG in improving their search relevance and precision. Let's take a look at a few case studies:
Industry | Case Study | Impact |
E-commerce | A leading online retailer implemented RAG to enhance its product search functionality. By leveraging contextual understanding, the system was able to deliver highly relevant product recommendations, leading to a 20% increase in conversion rates and a 15% boost in average order value. | - Increased conversion rates - Higher average order value - Improved customer satisfaction |
Healthcare | A major healthcare provider adopted RAG to optimize its electronic health record (EHR) search capabilities. With RAG, physicians and healthcare professionals were able to quickly retrieve relevant patient information, leading to a 25% reduction in search time and improved patient care outcomes. | - Reduced search time - Enhanced patient care - Increased efficiency |
Financial Services | A global financial institution implemented RAG to streamline its internal knowledge base search. The system's ability to understand complex financial terminology and provide accurate, context-aware results led to a 30% reduction in search-related support tickets and increased employee productivity. | - Decreased support tickets - Improved employee productivity - Better knowledge sharing |
These case studies demonstrate the tangible impact of RAG on search relevance and precision across various industries. By delivering more accurate and contextually relevant search results, RAG empowers enterprises to unlock the full potential of their data assets and drive meaningful business outcomes.
RAG's Role in Resource Optimization and Cost-Efficiency
In addition to enhancing search relevance and precision, Retrieval-Augmented Generation (RAG) plays a crucial role in optimizing resources and driving cost-efficiency within enterprises. By automating complex search processes and reducing manual effort, RAG enables organizations to streamline their operations and achieve significant cost savings.
Automating Complex Search Processes
One of the key advantages of RAG is its ability to automate complex search processes. Traditional search methods often require significant manual effort, with employees spending countless hours sifting through large volumes of data to find relevant information. RAG eliminates this burden by automating the search process, leveraging advanced retrieval models and generative models to quickly and accurately identify the most pertinent data.
This automation not only saves time but also frees up valuable human resources to focus on higher-value tasks, such as analysis, decision-making, and innovation. By reducing the manual effort required for search-related activities, RAG enables enterprises to optimize their workforce and improve overall productivity.
Reducing Manual Effort and Optimizing Resource Allocation
The automation capabilities of RAG extend beyond simple search processes. RAG can also be integrated with other enterprise systems, such as content management platforms, customer relationship management (CRM) tools, and knowledge management solutions. By seamlessly connecting these systems, RAG enables the automatic retrieval and generation of relevant information across various business functions.
This integration further reduces manual effort and optimizes resource allocation, as employees no longer need to switch between multiple systems or manually search for information in different databases. RAG provides a unified and intelligent search experience, delivering the right information to the right people at the right time.
Cost-Saving Benefits and Improved Operational Efficiency
The resource optimization and automation capabilities of RAG directly translate to significant cost savings for enterprises. By reducing the time and effort required for search-related tasks, organizations can achieve the following cost-saving benefits:
Cost-Saving Benefit | Description |
Reduced Labor Costs | RAG eliminates the need for manual search efforts, reducing the number of hours employees spend on search-related tasks. This translates to lower labor costs and increased productivity. |
Improved Efficiency | With RAG, employees can quickly access the information they need, without spending time navigating through irrelevant data. This improved efficiency leads to faster decision-making, reduced project timelines, and overall operational excellence. |
Decreased Infrastructure Costs | RAG's ability to efficiently retrieve and generate relevant information reduces the need for extensive storage and processing infrastructure. This leads to lower hardware and maintenance costs, as well as reduced energy consumption. |
Minimized Opportunity Costs | By freeing up employees' time from manual search tasks, RAG allows them to focus on higher-value activities that drive innovation and growth. This minimizes the opportunity costs associated with suboptimal resource utilization. |
The cost-saving benefits of RAG are not limited to a specific industry or business size. Organizations across various sectors, from healthcare and finance to manufacturing and retail, can leverage RAG to optimize their resources and improve their bottom line.
For example, a global consulting firm implemented RAG to streamline its internal knowledge sharing processes. By automating the retrieval and generation of relevant information from its vast knowledge base, the firm was able to reduce the time consultants spent searching for information by 40%. This translated to an annual cost saving of over $5 million, while simultaneously improving the quality and timeliness of client deliverables.
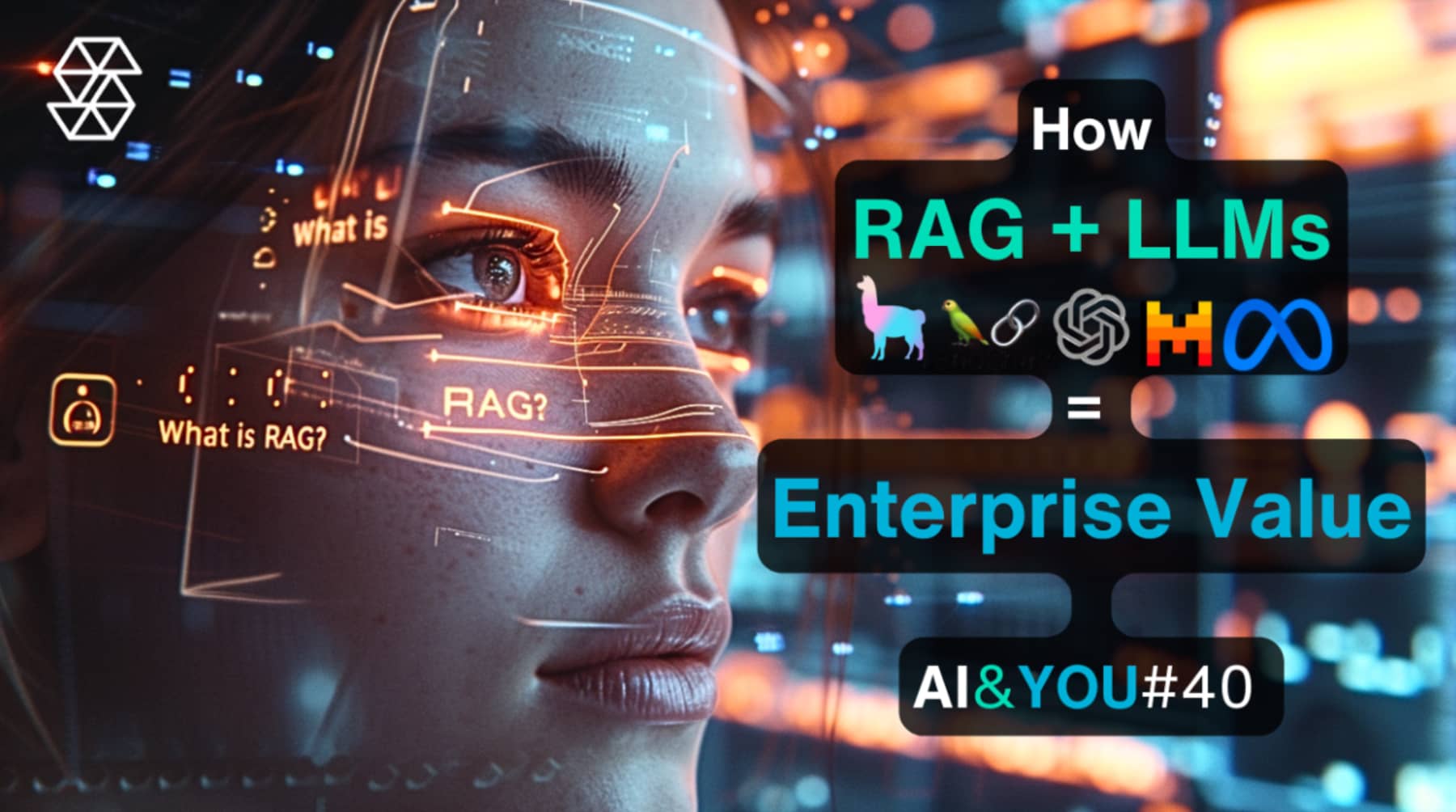
RAG's Influence on Decision-Making and Business Intelligence
Beyond optimizing resources and driving cost-efficiency, Retrieval-Augmented Generation (RAG) has a profound impact on decision-making and business intelligence within enterprises. By leveraging RAG-powered insights from extensive data sets, organizations can make informed, data-driven decisions that promote agility and adaptability in dynamic business environments.
Leveraging RAG-Powered Insights from Extensive Data Sets
RAG's ability to retrieve and generate relevant information from vast data repositories provides enterprises with a powerful tool for uncovering valuable insights. By analyzing structured and unstructured data from various sources, such as customer interactions, market trends, and operational metrics, RAG can identify patterns, correlations, and opportunities that might otherwise go unnoticed.
These RAG-powered insights can be used to:
Identify emerging market trends and customer preferences
Detect potential risks and challenges
Uncover new revenue streams and growth opportunities
Optimize operational processes and resource allocation
Enhance product development and innovation
With RAG, enterprises can harness the full potential of their data assets, turning raw information into actionable intelligence that drives strategic decision-making.
Enabling Data-Driven Decision-Making
RAG empowers organizations to make data-driven decisions by providing decision-makers with timely, accurate, and relevant information. Instead of relying on gut instincts or limited data points, executives can leverage RAG-generated insights to make informed choices based on comprehensive and up-to-date data.
This data-driven approach to decision-making offers several key benefits:
Benefit | Description |
Improved Accuracy | RAG ensures that decisions are based on accurate and relevant information, reducing the risk of errors or misinterpretations. |
Faster Decision-Making | With RAG, decision-makers can quickly access the information they need, enabling them to make timely decisions in fast-paced business environments. |
Greater Confidence | Data-driven decisions backed by RAG-powered insights provide decision-makers with greater confidence in their choices, as they are supported by robust data analysis. |
Enhanced Collaboration | RAG facilitates data-driven collaboration across departments and functions, as all stakeholders have access to the same high-quality information. |
Promoting Agility and Adaptability in Dynamic Business Environments
In today's rapidly evolving business landscape, agility and adaptability are essential for success. RAG plays a crucial role in helping enterprises navigate dynamic market conditions by providing real-time insights and enabling quick decision-making.
By continuously monitoring and analyzing data from various sources, RAG can alert decision-makers to emerging trends, potential disruptions, and new opportunities. This real-time intelligence allows organizations to proactively adapt their strategies, pivot their operations, and seize new growth opportunities before their competitors.
For example, a leading e-commerce company used RAG to monitor customer sentiment and market trends during the COVID-19 pandemic. By analyzing data from social media, customer reviews, and sales metrics, the company was able to quickly identify shifting consumer preferences and adjust its product offerings and marketing strategies accordingly. As a result, the company experienced a 30% increase in online sales and gained a significant market share during the pandemic.
RAG's influence on decision-making and business intelligence extends beyond real-time adaptability. By providing a comprehensive view of an organization's data landscape, RAG enables enterprises to develop long-term strategies that are grounded in data-driven insights. This strategic approach ensures that organizations are well-positioned to capitalize on future opportunities and maintain a competitive edge in their respective markets.
Comparative Analysis of RAG Platform Solutions
As enterprises increasingly recognize the transformative potential of Retrieval-Augmented Generation (RAG) in their search strategies, the market has responded with a range of powerful platform solutions. In this section, we will provide a comparative analysis of leading RAG platforms, examining their key features, strengths, and unique selling points.
Azure AI Search
Azure AI Search is a comprehensive RAG solution that leverages Microsoft's robust cloud infrastructure and advanced AI capabilities. Key features of Azure AI Search include:
Advanced indexing and query capabilities
Seamless integration with Azure Open AI and Language Understanding models
Extensive documentation and support for RAG design patterns
High security and reliability backed by Azure's cloud infrastructure
Azure AI Search is an excellent choice for organizations already invested in the Microsoft ecosystem, as it seamlessly integrates with other Azure services and provides a familiar development environment.
AWS Kendra and Amazon SageMaker JumpStart
AWS Kendra is a highly scalable RAG platform that combines natural language processing (NLP) with machine learning to deliver powerful search experiences. With Kendra, enterprises can:
Automatically index and search across multiple data sources
Leverage pre-built connectors for popular data repositories
Benefit from intelligent ranking and relevance tuning
Integrate with Amazon Comprehend for advanced NLP capabilities
In addition to Kendra, Amazon SageMaker JumpStart provides a streamlined way to implement RAG using pre-built models and workflows. With SageMaker JumpStart, data scientists and developers can quickly deploy RAG solutions without the need for extensive machine learning expertise.
Google Cloud Search
Google Cloud Search is a fully managed RAG platform that leverages Google's extensive experience in search and AI. Key features of Google Cloud Search include:
Powerful query understanding and relevance ranking
Integration with Google's Knowledge Graph for enhanced context understanding
Support for multiple data sources and file formats
Advanced security and compliance features
Google Cloud Search is particularly well-suited for enterprises that rely heavily on Google Workspace applications, as it seamlessly integrates with tools like Gmail, Drive, and Docs.
IBM Watson Discovery
IBM Watson Discovery is an AI-powered RAG platform that combines advanced NLP with machine learning to deliver intelligent search experiences. With Watson Discovery, enterprises can:
Automatically extract insights from unstructured data sources
Benefit from pre-built industry-specific models and ontologies
Leverage natural language queries for intuitive search interactions
Integrate with IBM's broader Watson AI ecosystem
Watson Discovery is an excellent choice for organizations in regulated industries, such as healthcare and finance, as it offers robust security and compliance features.
Databricks
Databricks is a unified data analytics platform that supports RAG workloads through its integration with popular open-source frameworks and libraries. With Databricks, enterprises can:
Build and deploy RAG models using Python, R, and SQL
Leverage the power of Apache Spark for distributed data processing
Benefit from a collaborative and scalable environment for data science and engineering
Integrate with a wide range of data sources and machine learning tools
Databricks is particularly well-suited for organizations with complex data infrastructures and a strong focus on data science and machine learning.
Anthropic and OpenAI
Anthropic and OpenAI are leading providers of state-of-the-art language models and RAG technologies. While they do not offer end-to-end RAG platforms, their models and APIs can be integrated into existing search infrastructures to deliver advanced NLP capabilities.
Anthropic is known for its focus on safe and ethical AI development, making it an attractive choice for enterprises with strong corporate social responsibility commitments. OpenAI, on the other hand, is renowned for its cutting-edge research and powerful language models, such as GPT-3.
The table below provides a quick comparison of the key features and strengths of each RAG platform solution:
Platform | Key Features | Strengths |
Azure AI Search | - Advanced indexing and querying - Integration with Azure AI services - Extensive RAG design support | - Seamless integration with Microsoft ecosystem - Robust security and reliability |
AWS Kendra | - Intelligent ranking and relevance tuning - Pre-built connectors for data sources - Integration with Amazon Comprehend | - Highly scalable and flexible - Intelligent document processing |
Google Cloud Search | - Powerful query understanding - Integration with Knowledge Graph - Support for multiple data sources | - Seamless integration with Google Workspace - Advanced security and compliance |
IBM Watson Discovery | - Pre-built industry-specific models - Natural language querying - Integration with Watson AI ecosystem | - Robust security and compliance - Ideal for regulated industries |
Databricks | - Support for popular open-source frameworks - Distributed data processing with Apache Spark - Collaborative data science environment | - Ideal for complex data infrastructures - Strong focus on data science and machine learning |
Anthropic | - Safe and ethical AI development - Advanced language models | - Commitment to responsible AI - Flexible integration options |
OpenAI | - State-of-the-art language models (e.g., GPT-3) - Cutting-edge research | - Powerful generative capabilities - Broad applicability across industries |
Choosing the right RAG platform solution depends on various factors, including an organization's existing technology stack, industry-specific requirements, and AI maturity level. By carefully evaluating the features and strengths of each platform, enterprises can select the solution that best aligns with their search strategies and business objectives.
Measuring and Assessing RAG's ROI
As enterprises invest in Retrieval-Augmented Generation (RAG) to transform their search strategies, it is crucial to measure and assess the return on investment (ROI) of these initiatives. By establishing clear key performance indicators (KPIs) and tracking the impact of RAG on various aspects of the business, organizations can validate the value of their investments and make informed decisions about future deployments.
Key Performance Indicators (KPIs) for Evaluating RAG's ROI
To effectively measure the ROI of RAG implementations, enterprises should focus on a set of well-defined KPIs that align with their specific business objectives. Some of the most important KPIs to consider include:
Search Accuracy: This KPI measures the percentage of search queries that return relevant and accurate results. By tracking improvements in search accuracy, enterprises can quantify the impact of RAG on user satisfaction and productivity.
Search Time Reduction: This KPI measures the average time it takes for users to find the information they need using RAG-powered search. By comparing search times before and after RAG implementation, enterprises can demonstrate the efficiency gains achieved through the technology.
Operational Cost Savings: This KPI tracks the reduction in operational costs associated with search-related activities, such as manual data processing, content curation, and IT support. By quantifying the cost savings achieved through RAG, enterprises can demonstrate the financial value of their investments.
Other important KPIs to consider include user adoption rates, employee satisfaction scores, and the impact of RAG on specific business metrics, such as customer retention, sales conversion rates, and time-to-market for new products or services.
Case Studies Showcasing Successful RAG Implementations and ROI
To further illustrate the potential ROI of RAG, let's examine a few case studies that showcase successful implementations across different industries:
Global Financial Services Firm: A leading financial services company implemented RAG to improve the accuracy and efficiency of its investment research processes. By leveraging RAG to quickly identify relevant market insights and analyst reports, the firm was able to reduce research time by 45% and increase the quality of its investment recommendations. As a result, the firm achieved a 12% increase in portfolio returns and a 20% reduction in research-related costs.
Multinational Retail Corporation: A large retail company deployed RAG to optimize its e-commerce search experience. By using RAG to deliver more relevant and personalized product recommendations, the company achieved a 25% increase in online conversion rates and a 15% increase in average order value. Additionally, the company reduced its customer support costs by 30% due to fewer inquiries related to product findability.
Global Healthcare Provider: A leading healthcare organization used RAG to improve the accuracy and speed of its medical literature search capabilities. By enabling doctors and researchers to quickly find relevant clinical studies and patient case histories, the organization reduced the average time spent on literature reviews by 50%. This efficiency gain allowed the organization to accelerate its drug development pipeline and bring new treatments to market 20% faster than before.
These case studies demonstrate the tangible ROI that enterprises can achieve by implementing RAG in their search strategies. By carefully tracking KPIs and measuring the impact of RAG on key business metrics, organizations can build a strong business case for continued investment in this transformative technology.
Industry | Company Type | RAG Impact | ROI |
Financial Services | Global Financial Services Firm | - 45% reduction in research time - Increased quality of investment recommendations | - 12% increase in portfolio returns - 20% reduction in research-related costs |
Retail | Multinational Retail Corporation | - 25% increase in online conversion rates - 15% increase in average order value - 30% reduction in customer support costs | - Significant revenue growth - Improved customer satisfaction - Lower support costs |
Healthcare | Global Healthcare Provider | - 50% reduction in literature review time - Accelerated drug development pipeline | - 20% faster time-to-market for new treatments - Increased R&D productivity |
As the adoption of RAG continues to grow across industries, it is important for enterprises to prioritize the measurement and assessment of ROI to ensure the long-term success and sustainability of their RAG initiatives. By tying RAG investments to concrete business outcomes and demonstrating clear value creation, organizations can justify further expansion and optimization of their RAG-powered search strategies.
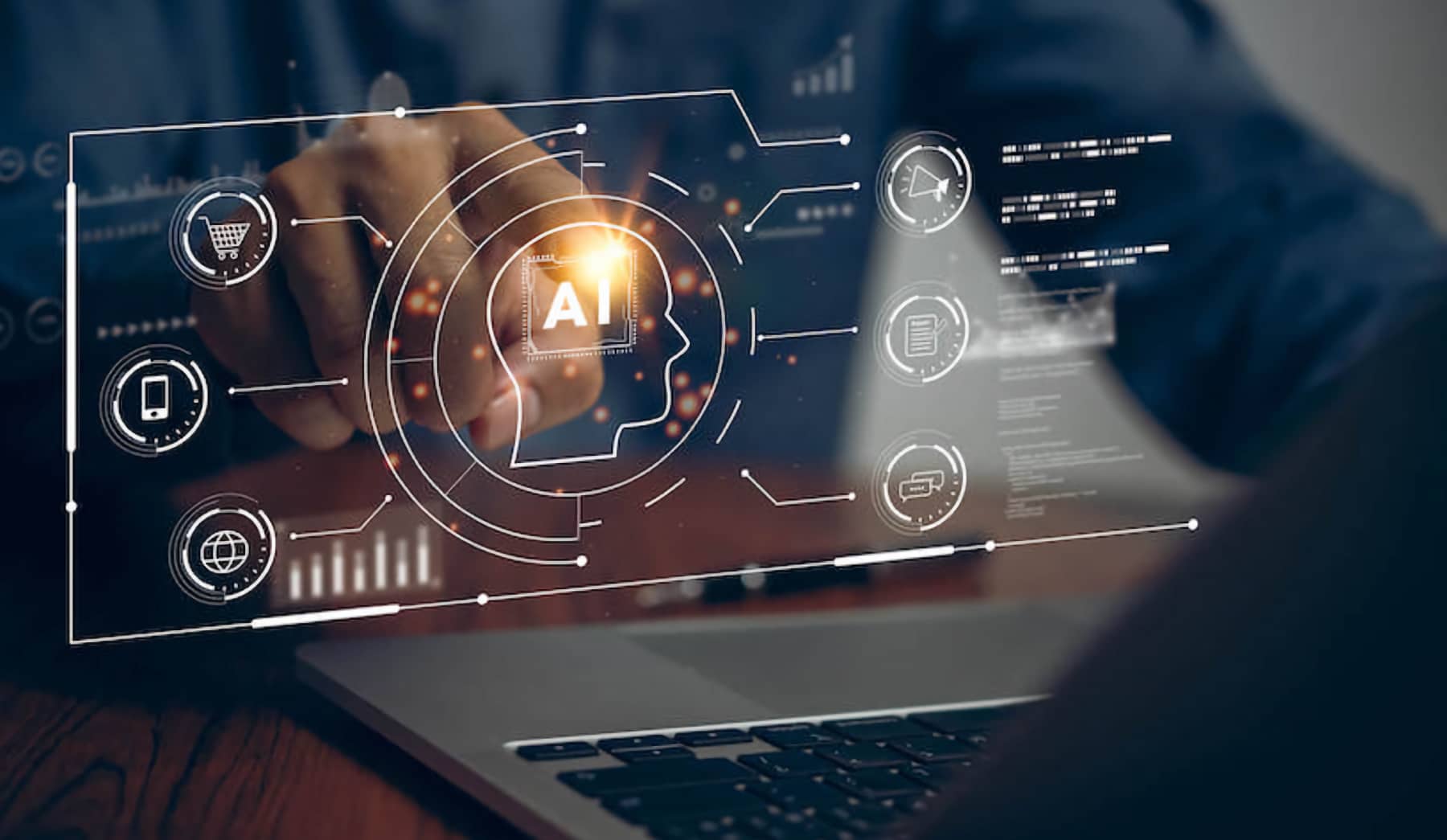
Challenges and Considerations in Implementing RAG
While Retrieval-Augmented Generation (RAG) offers significant benefits for enterprise search strategies, organizations must also navigate a range of challenges and considerations when implementing this technology. By proactively addressing these issues, enterprises can ensure a smooth and successful deployment of RAG and maximize the value of their investments.
Data Privacy and Security Concerns
One of the primary challenges in implementing RAG is ensuring the privacy and security of sensitive data. As RAG systems process and analyze large volumes of enterprise data, organizations must put robust safeguards in place to protect against unauthorized access, data breaches, and other security threats.
To address these concerns, enterprises should:
Implement strong access controls and authentication mechanisms
Encrypt data both at rest and in transit
Regularly audit and monitor RAG systems for potential vulnerabilities
Ensure compliance with relevant data protection regulations, such as GDPR and HIPAA
By prioritizing data privacy and security, organizations can build trust with their stakeholders and minimize the risk of costly data breaches or reputational damage.
Data Quality and Integration Complexities
Another significant challenge in implementing RAG is ensuring the quality and consistency of the data used to train and operate the system. Poor data quality, such as incomplete, inaccurate, or inconsistent information, can significantly impact the performance and reliability of RAG models.
To overcome this challenge, enterprises should invest in robust data governance and data quality initiatives, including:
Establishing clear data standards and definitions
Implementing data validation and cleansing processes
Regularly monitoring and measuring data quality metrics
Leveraging data quality tools and automation technologies
In addition to data quality, organizations must also navigate the complexities of integrating RAG with their existing IT infrastructures and data sources. This may require significant effort to develop custom connectors, APIs, and data pipelines to ensure seamless data flow and interoperability.
The Need for Specialized Skills and Cultural Shifts
Implementing RAG often requires a range of specialized skills and expertise, including data science, machine learning, natural language processing, and software engineering. Many organizations may lack these skills in-house, making it necessary to invest in talent acquisition, training, and development initiatives.
Moreover, successfully adopting RAG may require significant cultural shifts within the organization, as employees adapt to new ways of working and collaborating with AI-powered systems. Change management and communication efforts are crucial to ensure buy-in and support from stakeholders across the enterprise.
To address these challenges, organizations can:
Partner with external experts and consultants to fill skill gaps and accelerate implementation
Develop internal training and upskilling programs to build RAG expertise over time
Foster a culture of innovation and experimentation to encourage adoption of new technologies
Communicate the benefits and potential impact of RAG clearly and consistently to all stakeholders
Challenge | Mitigation Strategies |
Data Privacy and Security | - Implement access controls and encryption - Regularly audit and monitor RAG systems - Ensure compliance with data protection regulations |
Data Quality and Integration | - Establish data standards and definitions - Implement data validation and cleansing processes - Leverage data quality tools and automation |
Specialized Skills and Cultural Shifts | - Partner with external experts and consultants - Develop internal training and upskilling programs - Foster a culture of innovation and experimentation - Communicate benefits and impact clearly to stakeholders |
By proactively addressing these challenges and considerations, enterprises can lay the foundation for successful RAG implementations and unlock the full potential of this transformative technology.
As RAG continues to evolve and mature, it is crucial for organizations to stay informed about the latest developments and best practices in the field. In the next section, we will explore the future of RAG in enterprise search and discuss how organizations can position themselves for long-term success in this rapidly evolving landscape.
The Future of RAG in Enterprise Search
As Retrieval-Augmented Generation (RAG) continues to transform enterprise search strategies, it is crucial for organizations to stay ahead of the curve and anticipate future developments in this rapidly evolving field. By understanding the key trends and innovations shaping the future of RAG, enterprises can position themselves for long-term success and maintain a competitive edge in their industries.
Continuous Evolution of RAG Technologies
One of the most significant trends in the future of RAG is the continuous evolution of the underlying technologies, such as natural language processing (NLP), machine learning (ML), and deep learning (DL). As these technologies advance, RAG systems will become increasingly sophisticated, enabling even more accurate and contextually relevant search experiences.
Some of the key areas of innovation to watch include:
Advances in language models: The development of more powerful and efficient language models, such as GPT-4 and beyond, will enable RAG systems to better understand and generate human-like language, improving the quality and naturalness of search results.
Multimodal RAG: The integration of multiple data modalities, such as text, images, and voice, will allow RAG systems to process and generate more diverse and rich search experiences, enhancing user engagement and satisfaction.
Explainable AI: As RAG systems become more complex, there will be a growing need for explainable AI techniques that provide transparency into how search results are generated, building trust and accountability with users.
Integration with Advancing AI and Machine Learning
Another important trend in the future of RAG is the increasing integration with broader AI and machine learning technologies. As organizations invest in building comprehensive AI ecosystems, RAG will become a key component of these larger systems, enabling seamless data flow and insights across various business functions.
For example, RAG could be integrated with:
Intelligent automation platforms: RAG could power intelligent search and retrieval capabilities within robotic process automation (RPA) and intelligent automation platforms, enabling more efficient and accurate processing of unstructured data.
Predictive analytics and forecasting: RAG could be combined with predictive analytics and forecasting models to provide more proactive and anticipatory search experiences, helping users find information before they even realize they need it.
Conversational AI and chatbots: RAG could be integrated with conversational AI and chatbot platforms to provide more natural and interactive search experiences, allowing users to access information through voice commands and natural language queries.
Setting New Benchmarks for Excellence in the Digital Age
As RAG technologies continue to advance and mature, they will set new benchmarks for excellence in enterprise search and knowledge management. Organizations that successfully harness the power of RAG will be able to deliver unparalleled user experiences, improve operational efficiency, and drive innovation across their businesses.
To stay ahead of the curve and capitalize on the future of RAG, enterprises should:
Invest in ongoing research and development: Organizations should dedicate resources to staying informed about the latest advances in RAG and related technologies, and invest in ongoing research and development to stay at the forefront of innovation.
Foster a culture of continuous learning: Enterprises should cultivate a culture of continuous learning and upskilling, ensuring that their employees have the knowledge and skills necessary to work effectively with RAG systems and drive their evolution over time.
Collaborate with industry partners: Organizations should actively seek out partnerships and collaborations with leading technology vendors, research institutions, and industry consortia to access cutting-edge expertise and resources in the field of RAG.
Key Trend | Implications for Enterprise Search |
Continuous Evolution of RAG Technologies | - More accurate and contextually relevant search experiences - Integration of multiple data modalities - Growing need for explainable AI techniques |
Integration with Advancing AI and Machine Learning | - Seamless data flow and insights across business functions - Integration with intelligent automation, predictive analytics, and conversational AI - More proactive and anticipatory search experiences |
Setting New Benchmarks for Excellence | - Unparalleled user experiences - Improved operational efficiency - Driving innovation across the enterprise |
By embracing these trends and investing in the future of RAG, organizations can position themselves at the forefront of the digital age, unlocking new levels of insight, efficiency, and innovation in their enterprise search strategies.
Embracing the Transformative Power of RAG for Enterprise Search Excellence
Throughout this article, we have explored the profound impact of Retrieval-Augmented Generation (RAG) on enterprise search strategies. From its core principles and technical foundations to its transformative potential across various business functions, RAG emerges as a powerful catalyst for innovation, efficiency, and competitive advantage in the digital age.
By harnessing the synergistic combination of advanced information retrieval and natural language generation, RAG enables organizations to unlock the full value of their data assets, delivering more accurate, relevant, and contextually aware search experiences. The impact of RAG extends far beyond incremental improvements in search accuracy and speed, fundamentally reshaping the way enterprises approach knowledge management, decision-making, and customer engagement.
As we have seen through real-world case studies and ROI analyses, the successful implementation of RAG can yield significant benefits, including increased operational efficiency, enhanced employee productivity, improved customer satisfaction, and accelerated innovation cycles. However, realizing these benefits requires a strategic and holistic approach, one that addresses key challenges such as data privacy, data quality, and the need for specialized skills and cultural adaptations.
Looking ahead, the future of RAG is marked by rapid advancements in underlying technologies, deeper integration with broader AI and machine learning ecosystems, and the emergence of new benchmarks for excellence in enterprise search. To stay at the forefront of this transformative journey, organizations must proactively invest in ongoing research and development, foster a culture of continuous learning, and forge strategic partnerships with industry leaders and innovators.
In conclusion, RAG represents a pivotal opportunity for enterprises to redefine the boundaries of what is possible in search and knowledge discovery. By embracing the transformative power of RAG and aligning it with their broader digital transformation initiatives, organizations can unlock unprecedented levels of insight, agility, and competitive differentiation. The future belongs to those who dare to reimagine enterprise search, harnessing the power of RAG to drive innovation, efficiency, and growth in the digital age.
The time to act is now. As the pace of technological change continues to accelerate, enterprises that hesitate risk being left behind. By embarking on the RAG journey today, organizations can lay the foundation for long-term success, building the capabilities and competencies needed to thrive in an increasingly complex and dynamic business landscape.
So let us seize this moment, embrace the transformative potential of RAG, and chart a course towards a future of unparalleled enterprise search excellence. The rewards are immense, the opportunities vast, and the impact truly transformative. Together, let us redefine the boundaries of what is possible, unlocking the full power of knowledge and insight for the benefit of our organizations, our customers, and society as a whole.