In today's rapidly evolving business landscape, artificial intelligence (AI) has become a crucial driver of innovation and efficiency across industries. As enterprises seek to harness the power of AI to gain a competitive edge, a groundbreaking technology called Retrieval-Augmented Generation (RAG) is emerging as a game-changer. RAG represents a significant leap forward in the field of enterprise AI, promising to revolutionize how businesses leverage their data to drive better decision-making and enhance customer experiences.
The Growing Importance of AI in Enterprises
The adoption of AI technologies has been on a steep upward trajectory in recent years, with enterprises recognizing the immense potential for automation, optimization, and insight generation. From streamlining operations and improving efficiency to personalizing customer interactions and uncovering new market opportunities, AI has become an indispensable tool for businesses looking to thrive in the digital age.
Industry | AI Applications |
Healthcare | Predictive diagnostics, personalized treatment plans, drug discovery |
Finance | Fraud detection, risk assessment, investment analysis |
Retail | Personalized recommendations, inventory management, demand forecasting |
Manufacturing | Predictive maintenance, quality control, supply chain optimization |
Introducing Retrieval-Augmented Generation (RAG)
Retrieval-Augmented Generation (RAG) is a cutting-edge approach that combines the power of retrieval mechanisms and natural language processing (NLP) to enable more accurate, relevant, and context-aware responses from AI systems. By integrating real-time data retrieval capabilities with advanced language generation models, RAG empowers enterprises to unlock the full potential of their data assets and deliver superior AI-driven experiences.
Purpose and Scope of the Article
This article aims to provide a comprehensive overview of Retrieval-Augmented Generation and its transformative impact on enterprise AI. We will delve into the key concepts and components of RAG, explore its advantages over traditional AI models, and showcase real-world applications across various industries. Furthermore, we will discuss the implementation considerations, best practices, and future potential of RAG in driving business success.
By the end of this article, readers will have a deep understanding of how RAG is revolutionizing enterprise AI and why it is poised to become an essential component of any organization's AI strategy. Whether you are a business leader, AI practitioner, or simply curious about the latest advancements in enterprise AI, this article will provide valuable insights and practical guidance on harnessing the power of Retrieval-Augmented Generation.
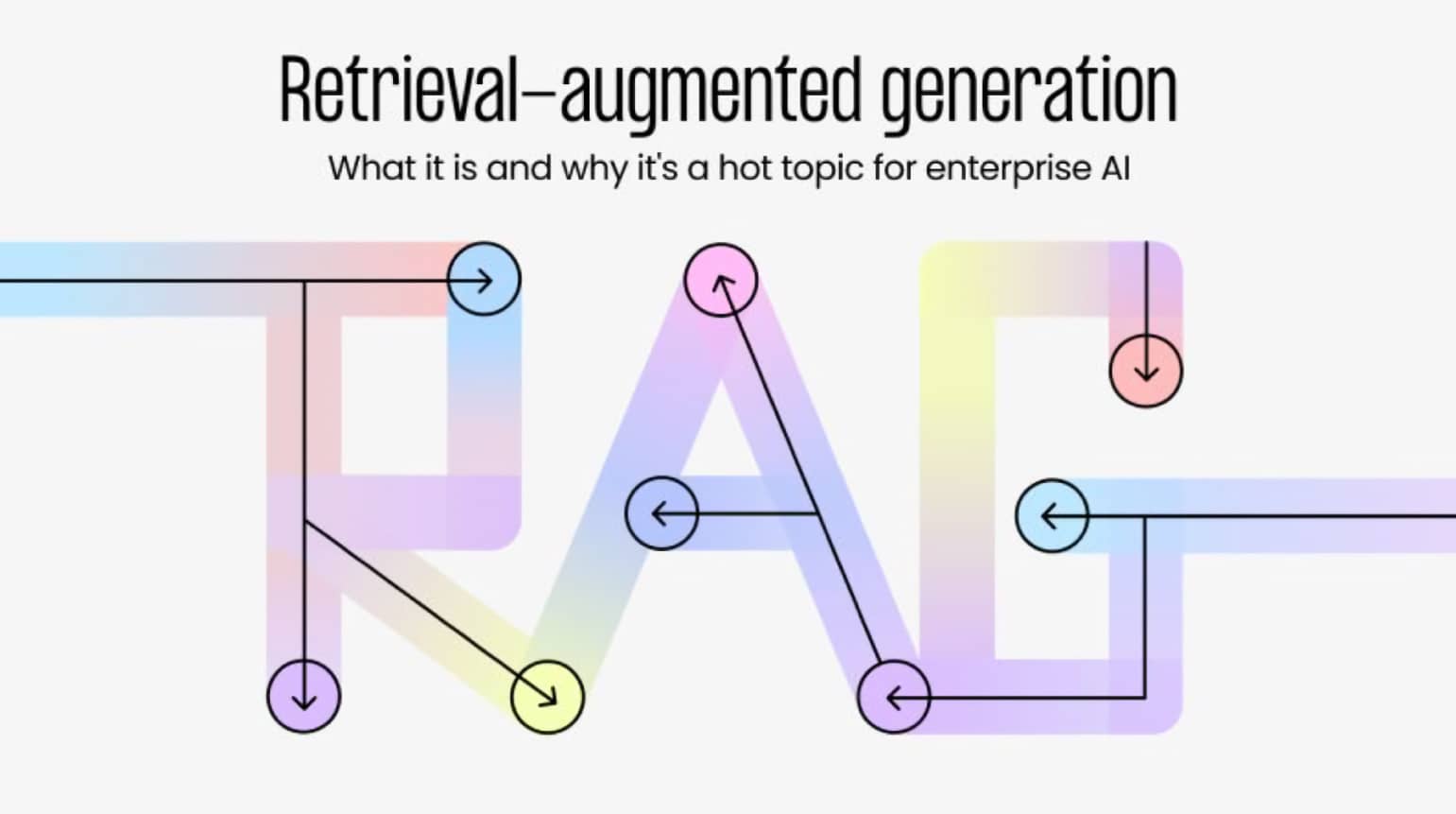
What is Retrieval-Augmented Generation (RAG)?
Retrieval-Augmented Generation (RAG) is an innovative approach that bridges the gap between data retrieval and language generation in AI systems. It combines the strengths of semantic search, vector databases, and natural language processing (NLP) to deliver more accurate, relevant, and up-to-date responses to user queries.
Definition and Key Concepts
At its core, RAG is a technique that enhances the capabilities of large language models (LLMs) by integrating them with a powerful retrieval mechanism. This retrieval mechanism allows the AI system to access and utilize external data sources in real-time, enabling it to provide more informative and contextually appropriate responses.
The key concepts underlying RAG include:
Semantic search: RAG leverages advanced semantic search techniques to identify and retrieve the most relevant information from vast data repositories. By understanding the intent behind user queries, RAG can surface the most pertinent data points to inform its responses.
Vector databases: RAG utilizes vector databases, which store data in a high-dimensional vector space, to efficiently index and search through large volumes of information. This enables quick and accurate retrieval of relevant data based on semantic similarity.
Natural language processing: RAG employs state-of-the-art NLP techniques to process and understand user queries, as well as to generate coherent and fluent responses. By leveraging the power of LLMs, RAG can engage in natural and contextually relevant conversations.
Components of RAG: Retriever and Generator
RAG consists of two primary components: the Retriever and the Generator. These components work together seamlessly to enable the AI system to retrieve relevant information and generate appropriate responses.
Retriever: The Retriever is responsible for searching through the vector database and identifying the most relevant data points based on the user's query. It employs sophisticated algorithms to measure the semantic similarity between the query and the stored information, ensuring that the most pertinent data is retrieved.
Generator: The Generator is typically a large language model that takes the retrieved information as input and generates a coherent and contextually appropriate response. It leverages the power of NLP to understand the context of the query and craft a response that is both informative and engaging.
By combining the strengths of the Retriever and the Generator, RAG enables enterprises to build AI systems that can provide accurate, up-to-date, and contextually relevant responses to a wide range of user queries.
How RAG Bridges Data and Language Models
One of the key advantages of RAG is its ability to bridge the gap between data and language models. Traditional language models rely solely on the information they were trained on, which can become outdated or limited in scope. RAG, on the other hand, allows language models to access and utilize external data sources in real-time, ensuring that the generated responses are based on the most current and relevant information available.
By seamlessly integrating data retrieval and language generation, RAG enables enterprises to leverage their vast data assets to enhance the performance and utility of their AI systems. This opens up new possibilities for knowledge-intensive tasks, such as market research, customer support, and content creation, where access to up-to-date and relevant information is critical.
Moreover, RAG's ability to retrieve information from domain-specific data sources enables enterprises to build AI systems that are tailored to their specific industry or use case. This level of customization and specificity is essential for delivering high-quality and actionable insights that drive business value.
Advantages of RAG in Enterprise AI
The integration of Retrieval-Augmented Generation (RAG) in enterprise AI systems offers numerous advantages that can significantly enhance the quality and effectiveness of AI-driven solutions. Let's explore some of the key benefits of RAG in the enterprise context.
Enhancing Accuracy and Relevance of Information
One of the primary advantages of RAG is its ability to enhance the accuracy and relevance of the information provided by AI systems. By leveraging semantic search capabilities and contextual response generation, RAG ensures that the retrieved data is not only factually correct but also pertinent to the user's specific query.
RAG's semantic search capabilities allow it to understand the intent behind user queries and retrieve information that is semantically related, rather than relying solely on keyword matching. This enables the AI system to provide more targeted and relevant responses, improving the overall user experience and satisfaction.
Furthermore, RAG's contextual response generation takes into account the retrieved information, the user's query, and the broader context of the conversation to craft responses that are coherent, informative, and engaging. This level of contextual awareness is crucial for building trust and credibility in AI-driven interactions.
Leveraging Up-to-Date External Data Sources
Another significant advantage of RAG is its ability to leverage up-to-date external data sources in real-time. Traditional AI systems often rely on static, pre-trained datasets, which can quickly become outdated in today's fast-paced business environment. RAG, on the other hand, enables real-time data retrieval from diverse sources, ensuring that the generated responses are based on the most current and relevant information available.
By accessing and integrating external data sources, such as market reports, news articles, social media feeds, and customer feedback, RAG empowers enterprises to make informed decisions based on the latest market trends, customer preferences, and competitive landscape. This real-time data integration is particularly valuable in industries such as finance, healthcare, and e-commerce, where timely and accurate information is critical for success.
Improving Efficiency in Knowledge-Intensive Tasks
RAG also offers significant improvements in efficiency for knowledge-intensive tasks within enterprises. By streamlining information retrieval and augmenting content creation, RAG enables employees to focus on higher-value activities, such as analysis, strategy development, and decision-making.
With RAG, employees can quickly access relevant information from vast data repositories without spending hours searching through documents or databases manually. The semantic search capabilities of RAG ensure that the most pertinent information is surfaced quickly, saving valuable time and effort.
Moreover, RAG can assist in content creation tasks, such as generating reports, summaries, or product descriptions. By leveraging the retrieved information and natural language generation capabilities, RAG can automatically generate draft content that is accurate, informative, and aligned with the desired context. This not only speeds up the content creation process but also ensures consistency and quality across different pieces of content.
The efficiency gains brought about by RAG can have a significant impact on productivity, allowing enterprises to scale their operations, serve more customers, and drive innovation at a faster pace.
Advantage | Description |
Accuracy and Relevance | RAG enhances the accuracy and relevance of information provided by AI systems through semantic search and contextual response generation. |
Up-to-Date Data | RAG enables real-time retrieval of up-to-date information from external data sources, ensuring that generated responses are based on the latest and most relevant data. |
Efficiency | RAG streamlines information retrieval and augments content creation, improving efficiency in knowledge-intensive tasks and allowing employees to focus on higher-value activities. |
By harnessing the power of RAG, enterprises can build AI systems that are more accurate, relevant, and efficient, ultimately driving better business outcomes and customer experiences.
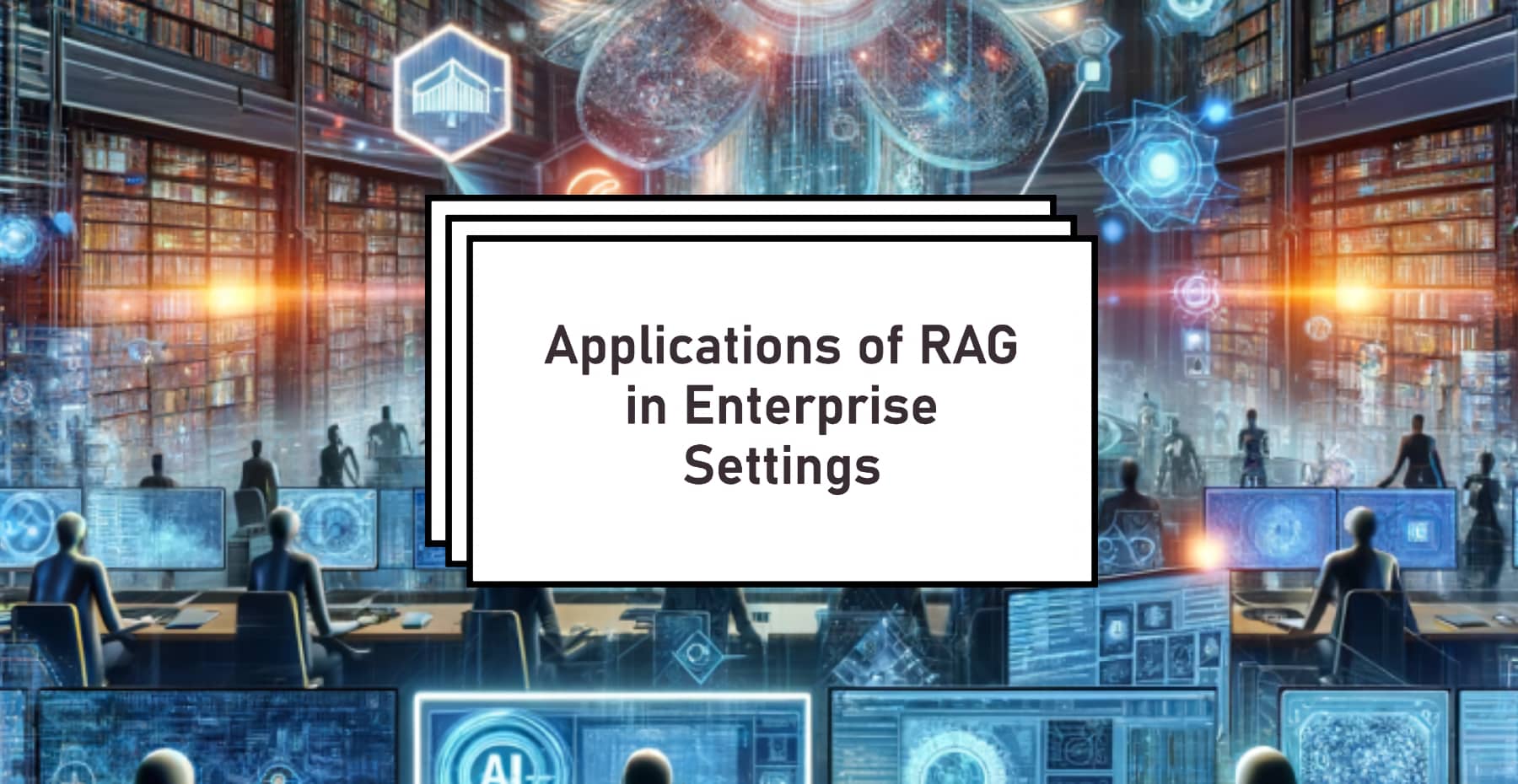
Applications of RAG in Enterprise Settings
The versatility and power of Retrieval-Augmented Generation (RAG) make it applicable to a wide range of enterprise settings. Let's explore some of the key areas where RAG can deliver significant value and transform business processes.
Semantic Search and Information Retrieval
One of the most prominent applications of RAG in enterprises is in the domain of semantic search and information retrieval. RAG's ability to understand the intent behind user queries and retrieve relevant information from vast data repositories makes it a game-changer for various industries.
Market Research and Analysis
In the realm of market research and analysis, RAG can significantly streamline the process of gathering and synthesizing relevant market insights. By leveraging semantic search capabilities, RAG can quickly identify and retrieve key information from diverse sources, such as industry reports, news articles, and social media mentions. This enables market researchers to access up-to-date and comprehensive data, allowing them to make informed decisions and stay ahead of the competition.
Legal Document Search and Review
In the legal industry, RAG can revolutionize the way legal professionals search and review documents. With the ability to understand legal terminology and context, RAG can efficiently retrieve relevant case law, contracts, and other legal documents from extensive databases. This not only saves time and effort but also ensures that legal teams have access to the most pertinent information when building cases or providing legal advice.
Customer Service and Support
Another key application of RAG in enterprises is in the area of customer service and support. By leveraging RAG's capabilities, businesses can deliver more personalized and efficient customer experiences, leading to increased customer satisfaction and loyalty.
Personalized Responses to Inquiries
RAG enables customer service chatbots and virtual assistants to provide personalized responses to customer inquiries. By retrieving relevant information from customer profiles, purchase histories, and product databases, RAG can generate contextually appropriate and tailored responses. This level of personalization helps to build stronger customer relationships and enhances the overall customer experience.
Access to Real-Time Product and Service Information
RAG also empowers customer service representatives with access to real-time product and service information. By integrating with inventory management systems, product catalogs, and knowledge bases, RAG can quickly retrieve up-to-date information about product specifications, availability, pricing, and troubleshooting guides. This enables customer service teams to provide accurate and timely assistance, reducing response times and improving customer satisfaction.
Content Creation and Marketing
RAG can also play a significant role in content creation and marketing efforts within enterprises. By leveraging the power of natural language generation and information retrieval, RAG can assist in producing high-quality and engaging content that resonates with target audiences.
Generating Relevant and Engaging Content
RAG can aid in the creation of relevant and engaging content by retrieving information from various sources and generating draft content based on the retrieved data. Whether it's writing blog posts, product descriptions, or social media updates, RAG can ensure that the generated content is accurate, informative, and aligned with the desired context. This not only saves time and effort for content creators but also helps to maintain consistency and quality across different pieces of content.
Aligning with Current Trends and Audience Preferences
RAG's ability to access and analyze real-time data from external sources, such as social media feeds and customer feedback, enables marketers to stay attuned to current trends and audience preferences. By understanding what topics and formats resonate with target audiences, RAG can assist in creating content that is more likely to engage and convert. This data-driven approach to content creation helps enterprises to optimize their marketing efforts and achieve better results.
Industry | RAG Application |
Market Research | Semantic search and retrieval of market insights from diverse sources |
Legal | Efficient search and review of legal documents, case law, and contracts |
Customer Service | Personalized responses to inquiries and access to real-time product information |
Content Marketing | Generation of relevant and engaging content aligned with current trends and audience preferences |
By leveraging RAG in these enterprise settings, businesses can unlock new levels of efficiency, personalization, and data-driven decision-making, ultimately driving growth and success in today's competitive landscape.
Implementing RAG in Enterprise AI Systems
To successfully implement Retrieval-Augmented Generation (RAG) in enterprise AI systems, organizations need to consider several key factors and best practices. Let's explore the essential aspects of RAG implementation, including data quality and management, integration with existing systems, and ethical considerations.
Data Quality and Management Considerations
One of the most critical factors in the success of RAG implementation is the quality and management of the data used for retrieval and generation. Enterprises must ensure that the data fed into RAG systems is accurate, relevant, and up-to-date to guarantee the reliability and effectiveness of the generated outputs.
Ensuring Data Accuracy and Relevance
To maintain data accuracy and relevance, enterprises should establish robust data governance processes and regularly review and update their data sources. This involves implementing data validation and cleansing techniques to identify and correct any errors, inconsistencies, or outdated information. Additionally, enterprises should carefully curate and select data sources that are most pertinent to their specific industry, use case, and target audience.
Structuring Data for Efficient Retrieval
Another key consideration in data management for RAG is structuring the data in a way that facilitates efficient retrieval. This involves organizing and indexing the data using appropriate taxonomies, ontologies, and metadata schemas. By structuring the data in a logical and searchable manner, enterprises can ensure that RAG systems can quickly and accurately retrieve the most relevant information for a given query.
Integration with Existing Enterprise Systems and Workflows
To maximize the value of RAG in enterprise AI systems, it is crucial to seamlessly integrate RAG capabilities with existing systems and workflows. This integration enables RAG to access and leverage the wealth of data and knowledge already present within the organization.
Enterprises should carefully assess their current IT infrastructure, data sources, and business processes to identify integration points and requirements. This may involve developing APIs, connectors, or middleware components to enable smooth data flow and interoperability between RAG and other enterprise systems, such as customer relationship management (CRM) platforms, enterprise resource planning (ERP) systems, and content management systems (CMS).
Moreover, integrating RAG into existing workflows requires close collaboration between IT teams, business units, and end-users. It is essential to involve stakeholders from various departments in the implementation process to ensure that RAG is aligned with their specific needs and requirements. This collaborative approach helps to foster adoption, drive business value, and ensure the long-term success of RAG within the enterprise.
Ethical and Privacy Concerns
As with any AI technology, the implementation of RAG in enterprises raises important ethical and privacy concerns. Organizations must navigate these challenges responsibly and establish clear guidelines and safeguards to protect sensitive data and ensure the ethical use of RAG.
Adhering to Data Privacy Regulations
Enterprises must ensure that their RAG implementation complies with relevant data privacy regulations, such as the General Data Protection Regulation (GDPR) or the California Consumer Privacy Act (CCPA). This involves obtaining necessary consents, implementing data protection measures, and providing transparency about how personal data is collected, used, and stored within the RAG system.
Maintaining Transparency in AI Decision-Making
Another critical ethical consideration is maintaining transparency in AI decision-making processes that involve RAG. Enterprises should strive to provide clear explanations and justifications for the outputs generated by RAG systems, especially when those outputs inform important business decisions or impact customers. This transparency helps to build trust, mitigate potential biases, and ensure accountability in the use of RAG.
Consideration | Description |
Data Quality | Ensure data accuracy, relevance, and currency through robust data governance processes |
Data Structuring | Organize and index data using appropriate taxonomies, ontologies, and metadata schemas for efficient retrieval |
System Integration | Integrate RAG capabilities with existing enterprise systems and workflows through APIs, connectors, or middleware components |
Ethical and Privacy | Adhere to data privacy regulations, maintain transparency in AI decision-making, and establish clear guidelines for ethical RAG use |
By carefully addressing these implementation considerations and following best practices, enterprises can harness the full potential of RAG while mitigating risks and ensuring responsible AI deployment.
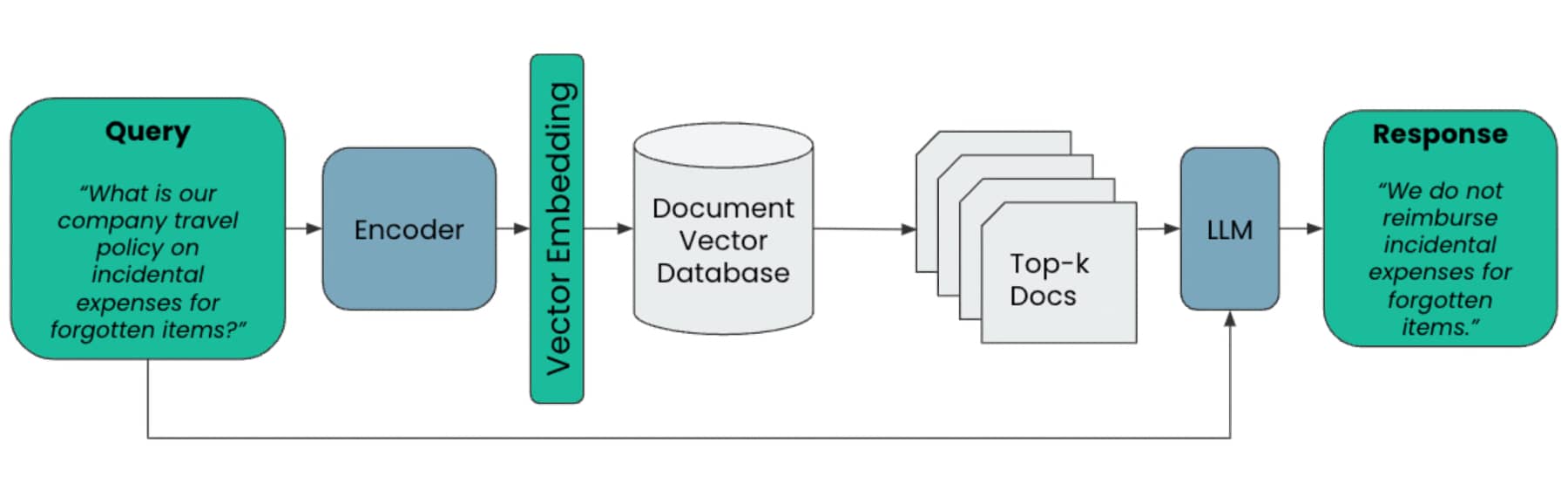
Real-World Examples of RAG in Action
To better understand the transformative potential of Retrieval-Augmented Generation (RAG) in enterprise AI, let's explore some real-world examples of how RAG has been successfully implemented across different industries.
Case Study 1: Enhancing Market Research Efficiency
A leading market research firm sought to streamline its research process and provide more comprehensive and up-to-date insights to its clients. By implementing RAG in their AI-powered research platform, the firm was able to significantly enhance the efficiency and quality of their market research efforts.
The RAG system allowed researchers to quickly retrieve relevant information from a vast repository of market reports, news articles, and social media data. By leveraging semantic search capabilities, the system could identify and surface the most pertinent insights based on specific research queries. This not only saved researchers countless hours of manual data gathering but also ensured that the insights provided to clients were accurate, timely, and actionable.
As a result of implementing RAG, the market research firm was able to deliver higher-quality research outputs in a fraction of the time, leading to increased client satisfaction and a significant competitive advantage in the industry.
Case Study 2: Transforming Customer Support in E-commerce
An e-commerce giant recognized the need to enhance its customer support capabilities to keep up with the growing demand and expectations of its customers. By integrating RAG into their customer support chatbot, the company was able to deliver more personalized and efficient support experiences.
The RAG-powered chatbot could retrieve relevant information from customer profiles, order histories, and product databases in real-time, enabling it to provide accurate and contextually appropriate responses to customer inquiries. This level of personalization not only improved the speed and quality of issue resolution but also fostered stronger customer relationships.
Moreover, the RAG system allowed the chatbot to access up-to-date information about product availability, shipping status, and promotional offers, ensuring that customers received the most current and reliable information. This helped to reduce customer frustration and improve overall satisfaction with the support experience.
The successful implementation of RAG in their customer support chatbot led to significant improvements in key metrics, such as reduced response times, increased first-contact resolution rates, and higher customer satisfaction scores.
Case Study 3: Optimizing Content Creation for Marketing Campaigns
A global marketing agency recognized the potential of RAG to revolutionize their content creation process and deliver more impactful marketing campaigns for their clients. By leveraging RAG in their content creation workflow, the agency was able to generate high-quality and engaging content at scale.
The RAG system could retrieve relevant information from various sources, such as industry reports, competitor analysis, and customer feedback, to inform the content creation process. By analyzing this data, the system could identify trending topics, popular formats, and key messaging points that resonated with target audiences.
Using this insights, the RAG system could generate draft content, such as blog posts, social media updates, and ad copy, that was aligned with the campaign objectives and audience preferences. The content creators could then review, refine, and approve the generated content, ensuring that it met the highest quality standards.
The integration of RAG in their content creation process allowed the marketing agency to produce more targeted and effective content in a shorter timeframe. This not only improved the efficiency of their content production but also led to better campaign results for their clients, including higher engagement rates, increased conversions, and improved brand awareness.
These real-world examples demonstrate the tangible benefits and transformative potential of RAG in various enterprise settings. By leveraging the power of RAG, businesses can unlock new levels of efficiency, personalization, and data-driven insights, ultimately driving better business outcomes and customer experiences.
Industry | Company | RAG Application | Results |
Market Research | Leading Market Research Firm | Enhanced research efficiency and insights quality | Faster delivery of high-quality research outputs, increased client satisfaction |
E-commerce | E-commerce Giant | Personalized and efficient customer support chatbot | Reduced response times, increased first-contact resolution, higher customer satisfaction |
Marketing | Global Marketing Agency | Optimized content creation for marketing campaigns | More targeted and effective content, improved campaign results, higher efficiency |
As more enterprises recognize the value of RAG and successfully implement it in their AI systems, we can expect to see even more innovative applications and success stories emerge across industries.
The Future of RAG in Enterprise AI
As we have seen, Retrieval-Augmented Generation (RAG) is already making significant strides in transforming enterprise AI applications across various industries. However, the potential of RAG extends far beyond its current implementations. Let's explore the future of RAG and how it is poised to shape the landscape of enterprise AI in the coming years.
Advancements in RAG Technology
The field of RAG is constantly evolving, with ongoing research and development efforts aimed at pushing the boundaries of what is possible. As RAG technology continues to advance, we can expect to see significant improvements in several key areas:
Improving Accuracy and Processing Speed
One of the primary focus areas for RAG advancements is enhancing the accuracy and processing speed of retrieval and generation processes. Researchers are exploring new techniques, such as advanced neural architectures, attention mechanisms, and transfer learning, to improve the precision and efficiency of RAG systems. These advancements will enable RAG to handle larger volumes of data, deliver more accurate results, and generate high-quality outputs in real-time.
Handling More Complex Queries and Tasks
Another exciting frontier in RAG development is expanding its capabilities to handle more complex queries and tasks. As RAG systems become more sophisticated, they will be able to understand and respond to increasingly nuanced and multi-faceted queries, providing more comprehensive and contextually relevant answers. Additionally, RAG will be able to take on more complex tasks, such as multi-step problem-solving, reasoning, and creative ideation, opening up new possibilities for enterprise AI applications.
Strategic Importance of RAG in Data-Driven Enterprises
As enterprises become increasingly data-driven, the strategic importance of RAG in leveraging that data for competitive advantage cannot be overstated. RAG will play a pivotal role in enabling organizations to harness the full potential of their data assets and drive innovation across all aspects of their business.
Gaining Competitive Advantage through Efficient Data Utilization
RAG will be a key differentiator for enterprises looking to gain a competitive edge in their respective markets. By enabling efficient and intelligent utilization of data, RAG will help organizations uncover hidden insights, identify emerging trends, and make data-driven decisions faster than ever before. This will allow enterprises to stay ahead of the curve, anticipate customer needs, and seize new opportunities before their competitors.
Driving Innovation and Decision-Making with Actionable Insights
RAG will also be instrumental in driving innovation and informing strategic decision-making within enterprises. By providing real-time access to relevant and actionable insights, RAG will empower business leaders and teams to make informed choices and develop innovative solutions to complex challenges. This will foster a culture of data-driven experimentation and continuous improvement, enabling enterprises to adapt and thrive in the face of rapid change and disruption.
Potential Future Applications and Developments
As RAG technology continues to evolve and mature, we can anticipate the emergence of new and exciting applications across various domains. Some potential future developments and applications of RAG in enterprise AI include:
Intelligent Automation: RAG could power intelligent automation systems that can understand and process unstructured data, enabling the automation of complex, knowledge-intensive tasks and workflows.
Augmented Analytics: RAG could revolutionize the field of data analytics by enabling natural language querying, automated insights generation, and self-service analytics for business users.
Personalized Recommendations: RAG could drive highly personalized and context-aware recommendation engines, transforming the way enterprises engage with customers and deliver tailored experiences.
Creative Ideation: RAG could support creative ideation processes by generating novel ideas, concepts, and solutions based on the synthesis of diverse data sources and domain knowledge.
As these potential applications illustrate, the future of RAG in enterprise AI is incredibly promising. As organizations continue to recognize the value and potential of RAG, we can expect to see a surge in adoption and innovation in this space, leading to new breakthroughs and transformative impacts across industries.
Challenges and Considerations in Implementing RAG
While the benefits and potential of Retrieval-Augmented Generation (RAG) in enterprise AI are undeniable, implementing RAG systems also comes with its own set of challenges and considerations. Organizations must be aware of these challenges and address them proactively to ensure successful RAG adoption and deployment.
Ensuring Data Quality and Relevance
One of the critical challenges in implementing RAG is ensuring the quality and relevance of the data used for retrieval and generation. RAG systems heavily rely on the underlying data to provide accurate and meaningful outputs. If the data is incomplete, inconsistent, or irrelevant, it can lead to suboptimal or even misleading results.
To mitigate this challenge, enterprises must invest in robust data governance practices, including regular data audits, cleansing, and validation processes. They should also establish clear data quality metrics and continuously monitor and improve the quality of their data assets. Additionally, organizations should carefully curate and select data sources that are most relevant to their specific use cases and industries to ensure the relevance of the retrieved information.
Addressing Ethical and Privacy Concerns
Another significant challenge in RAG implementation is addressing the ethical and privacy concerns associated with the use of AI and data. As RAG systems process and generate information based on large volumes of data, including potentially sensitive or personal data, organizations must ensure that they comply with relevant data protection regulations and ethical guidelines.
This involves implementing strong data security measures, such as encryption, access controls, and data anonymization techniques, to protect sensitive information. Organizations should also establish clear data usage policies and obtain necessary consents from individuals whose data is being used in RAG systems.
Moreover, enterprises must be transparent about how RAG systems are being used, what data is being processed, and how decisions are being made based on the generated outputs. This transparency is crucial for building trust with stakeholders and ensuring the responsible and ethical use of RAG technology.
Seamless Integration with Existing Systems and Workflows
Integrating RAG systems with existing enterprise systems and workflows can also present challenges. RAG implementation often requires significant changes to IT infrastructure, data pipelines, and business processes. Organizations must carefully plan and execute the integration process to ensure smooth adoption and minimize disruption to ongoing operations.
This involves conducting a thorough assessment of existing systems, identifying integration points, and developing robust APIs and connectors to enable seamless data flow and interoperability. It also requires close collaboration between IT teams, business units, and end-users to ensure that RAG systems are aligned with specific requirements and can be effectively leveraged within existing workflows.
Change management is another critical aspect of RAG integration. Organizations must provide adequate training and support to employees to help them understand and adopt RAG-powered tools and processes. This includes communicating the benefits of RAG, providing hands-on training, and establishing clear guidelines and best practices for using RAG systems effectively.
Best Practices for Successful RAG Implementation
To overcome the challenges and ensure successful RAG implementation, organizations should follow best practices and adopt a strategic approach. Here are some key best practices for implementing RAG in enterprise AI:
Defining Clear Objectives and Use Cases
Before embarking on RAG implementation, organizations should clearly define their objectives and identify specific use cases where RAG can deliver the most value. This involves conducting a thorough analysis of business needs, pain points, and opportunities, and aligning RAG initiatives with overall enterprise goals.
By having a clear understanding of the desired outcomes and use cases, organizations can prioritize their RAG efforts, allocate resources effectively, and measure the success of their RAG implementations against well-defined metrics.
Investing in Data Management and Infrastructure
Successful RAG implementation relies heavily on the quality and availability of data. Organizations must invest in robust data management practices and infrastructure to ensure that RAG systems have access to accurate, relevant, and up-to-date information.
This includes establishing data governance frameworks, implementing data quality controls, and building scalable data storage and processing infrastructure. Organizations should also invest in data integration technologies to enable seamless data flow between RAG systems and other enterprise applications.
Collaborating with Experienced AI Partners
Implementing RAG in enterprise AI can be a complex and resource-intensive endeavor. To accelerate RAG adoption and ensure successful implementation, organizations should consider collaborating with experienced AI partners who have deep expertise in RAG technologies and enterprise AI solutions.
These partners can provide valuable guidance, best practices, and proven methodologies for implementing RAG systems effectively. They can also offer pre-built RAG solutions, tools, and frameworks that can be customized to meet specific enterprise requirements, reducing development time and effort.
Continuously Monitoring and Refining RAG Models
RAG implementation is not a one-time effort but an ongoing process of continuous improvement. Organizations should establish mechanisms to monitor the performance of RAG models regularly and identify areas for optimization and refinement.
This involves tracking key performance indicators (KPIs) such as accuracy, response time, and user satisfaction, and using this data to fine-tune RAG algorithms and models. It also requires staying up-to-date with the latest advancements in RAG technology and incorporating new techniques and approaches as they emerge.
By continuously monitoring and refining RAG models, organizations can ensure that their RAG systems remain effective, relevant, and aligned with evolving business needs and user expectations.
Conclusion
Retrieval-Augmented Generation (RAG) represents a significant leap forward in the field of enterprise AI, offering immense potential for transforming how organizations leverage their data assets and drive business value. By combining the power of retrieval mechanisms and natural language processing, RAG enables enterprises to build AI systems that are more knowledgeable, contextually aware, and responsive to user needs.
Throughout this article, we have explored the key concepts, benefits, and applications of RAG in enterprise settings. We have seen how RAG can enhance the accuracy and relevance of information, leverage up-to-date external data sources, and improve efficiency in knowledge-intensive tasks. We have also examined real-world examples of RAG in action, demonstrating its transformative impact across industries such as market research, e-commerce, and marketing.
As we look to the future, the potential of RAG in enterprise AI is truly exciting. With ongoing advancements in RAG technology, we can expect to see even more sophisticated and powerful applications emerge, driving innovation, competitive advantage, and data-driven decision-making in organizations.
However, successfully implementing RAG in enterprise AI also requires careful consideration of challenges and best practices. Organizations must address data quality and relevance, ethical and privacy concerns, and seamless integration with existing systems and workflows. By following best practices such as defining clear objectives, investing in data management and infrastructure, collaborating with experienced AI partners, and continuously monitoring and refining RAG models, enterprises can overcome these challenges and realize the full potential of RAG.