The insurance industry is a complex and ever-evolving landscape, constantly facing challenges in risk assessment, underwriting, and customer service. Insurers must navigate through vast amounts of data, historical records, and evolving customer expectations to remain competitive and profitable. However, traditional methods of data analysis and decision-making often fall short in terms of accuracy, efficiency, and adaptability.
Enter Retrieval-Augmented Generation (RAG), a groundbreaking approach that combines the power of information retrieval and generative AI models to revolutionize the insurance industry. RAG empowers insurers to harness the full potential of their data, unlocking new possibilities for risk assessment, underwriting, and customer service.
In this article, we will explore the transformative potential of RAG in the insurance sector. We will delve into the technical aspects of RAG, its applications in various insurance domains, and the benefits it brings to insurers and customers alike. Moreover, we will examine real-world case studies, address challenges and considerations, and provide a glimpse into the future of RAG in insurance.
Understanding Retrieval-Augmented Generation (RAG)
At its core, Retrieval-Augmented Generation (RAG) is a powerful framework that bridges the gap between information retrieval and generative AI models. It leverages the strengths of both approaches to deliver accurate, contextual, and efficient results.
Combining Information Retrieval and Generative AI Models
RAG seamlessly integrates two key components: information retrieval and generative AI models. The information retrieval component focuses on accessing and selecting the most relevant data from internal sources, such as knowledge bases, databases, and documents. This data can include historical records, customer profiles, policy documents, and claim histories.
On the other hand, the generative AI component utilizes advanced language models and machine learning algorithms to analyze the retrieved data and generate meaningful insights, recommendations, and responses. These models are trained on vast amounts of data to understand complex patterns, relationships, and contextual nuances.
Retrieving Relevant Data from Internal Sources
One of the key strengths of RAG lies in its ability to retrieve relevant data from an organization's internal sources. Insurance companies possess a wealth of information scattered across various systems and repositories. RAG enables insurers to tap into this valuable data by employing techniques such as:
Document Encoding: RAG transforms unstructured data, such as policy documents and claim reports, into structured, machine-readable formats. This process involves techniques like tokenization, vectorization, and embedding, which convert text into numerical representations that can be efficiently processed by AI models.
Vector Databases: RAG leverages vector databases, such as ChromaDB or PineCone, to store and retrieve high-dimensional vectors representing insurance data. These databases enable fast and efficient similarity search, allowing insurers to find relevant information based on semantic similarity rather than exact keyword matches.
Knowledge Graphs: RAG incorporates knowledge graphs to capture the relationships and contextual information between entities in the insurance domain. Knowledge graphs provide a structured representation of data, enabling AI models to understand complex relationships and make more informed decisions.
Generating Accurate and Contextual Responses
Once the relevant data is retrieved, RAG employs generative AI models to analyze the information and generate accurate and contextual responses. These models, such as Large Language Models (LLMs), are trained on extensive datasets and can understand the nuances and complexities of insurance-related queries.
RAG enables insurers to generate responses that are tailored to specific customer needs, taking into account their individual circumstances, policy details, and historical data. This level of personalization enhances the customer experience and helps build trust and loyalty.
Advantages of RAG over Traditional AI Models
RAG offers several advantages over traditional AI models in the insurance industry:
Improved Accuracy: By retrieving relevant data from internal sources and leveraging generative AI models, RAG provides more accurate and reliable insights compared to models that rely solely on general knowledge or limited datasets.
Context-Aware Responses: RAG takes into account the specific context of each insurance query, generating responses that are tailored to the individual customer's needs and circumstances. This context-awareness leads to more meaningful and personalized interactions.
Efficient Data Retrieval: With the help of vector databases and knowledge graphs, RAG enables efficient retrieval of relevant information from vast amounts of insurance data. This efficiency saves time and resources, allowing insurers to respond quickly to customer queries and make informed decisions.
Scalability: RAG is designed to handle large-scale insurance datasets and can easily integrate with existing systems and workflows. As the volume of data grows, RAG can scale seamlessly, ensuring consistent performance and reliability.
Advantages of RAG | Traditional AI Models | RAG |
Accuracy | Limited | Improved |
Context-Awareness | Generic | Tailored |
Data Retrieval | Inefficient | Efficient |
Scalability | Restricted | Seamless |
By leveraging the power of RAG, insurance companies can unlock new levels of efficiency, accuracy, and customer satisfaction. In the following sections, we will explore how RAG is revolutionizing various aspects of the insurance industry, from underwriting to customer service and beyond.
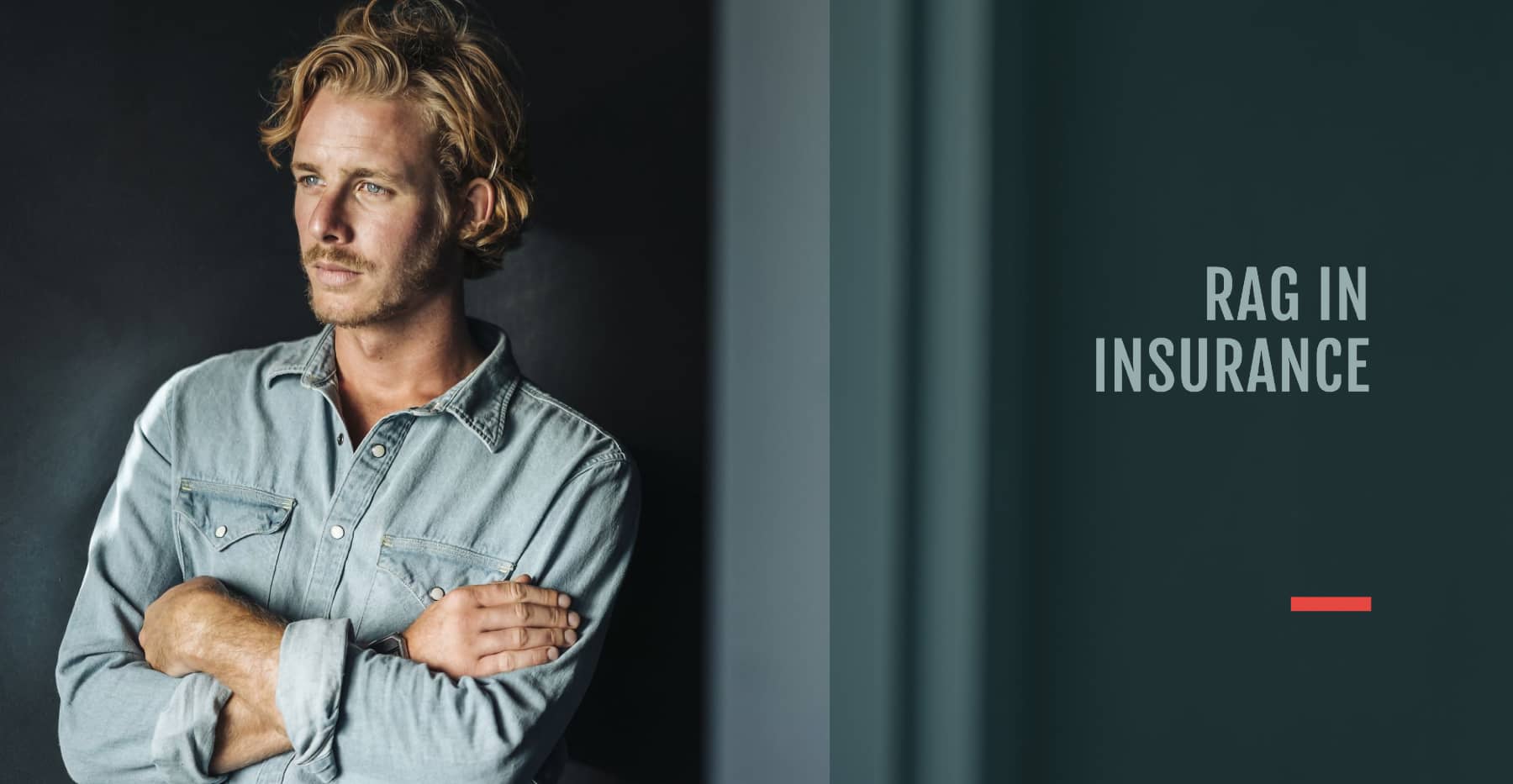
RAG in Insurance
Insurance underwriting is a critical process that involves assessing the risk associated with potential policyholders and determining the appropriate coverage and pricing. Traditionally, underwriting relied heavily on manual efforts, subjective judgment, and limited data analysis. However, RAG is revolutionizing the underwriting process by enabling insurers to make data-driven decisions and improve risk assessment accuracy.
Enhancing Risk Assessment Accuracy
RAG empowers insurers to enhance the accuracy of risk assessment by leveraging historical data and AI-powered insights. By retrieving relevant information from internal sources, such as policy documents, claim histories, and customer profiles, RAG provides underwriters with a comprehensive view of the potential risks associated with each applicant.
Moreover, RAG utilizes AI models to analyze the retrieved data and generate insights that go beyond traditional risk assessment methods. These models can identify patterns, correlations, and risk factors that may not be apparent to human underwriters. By considering a wide range of variables and historical data, RAG enables insurers to make more informed and accurate risk assessments.
Streamlining the Underwriting Process
In addition to improving accuracy, RAG streamlines the underwriting process by automating manual tasks and reducing the time and effort required to assess risk. With RAG, underwriters can quickly identify relevant data points and focus on the most critical aspects of each application.
RAG leverages techniques such as semantic search and vector embeddings to efficiently retrieve the most relevant information from vast amounts of insurance data. This allows underwriters to access the right data at the right time, without having to manually sift through numerous documents and records.
Furthermore, RAG can assist underwriters in making faster and more consistent decisions. By providing data-driven insights and recommendations, RAG helps underwriters prioritize high-risk cases and allocate their time and resources more effectively. This streamlined process not only saves time but also reduces the potential for human error and inconsistencies.
Mitigating Bias in Underwriting Decisions
Bias in underwriting decisions is a significant concern in the insurance industry. Traditional underwriting methods may be influenced by subjective judgments and unconscious biases, leading to unfair or discriminatory practices. RAG helps mitigate bias by relying on data-driven insights and objective criteria.
By leveraging AI models and statistical analysis, RAG ensures that underwriting decisions are based on factual data and unbiased algorithms. These models are trained on diverse datasets and are designed to identify and eliminate potential biases in the decision-making process.
RAG promotes fairness and transparency in underwriting by providing a clear audit trail of the data and insights used to make decisions. This transparency allows insurers to demonstrate their commitment to ethical and unbiased practices, enhancing customer trust and regulatory compliance.
Real-World Applications in Various Insurance Domains
RAG finds applications across various insurance domains, offering benefits specific to each sector:
Property & Casualty (P&C) Insurance: RAG can analyze historical claim data, property information, and risk factors to accurately assess the risk associated with insuring specific properties or individuals. It can identify patterns and correlations that help insurers price policies more accurately and reduce the likelihood of fraudulent claims.
Life & Health Insurance: RAG can assist in underwriting life and health insurance policies by analyzing medical records, lifestyle factors, and mortality data. It can identify potential health risks, predict future claims, and help insurers offer personalized coverage options based on individual risk profiles.
Commercial Insurance: RAG can streamline the underwriting process for commercial insurance by analyzing financial data, industry trends, and historical claim patterns. It can assess the risk associated with insuring specific businesses and help insurers make informed decisions about coverage limits, pricing, and risk mitigation strategies.
Insurance Domain | RAG Applications |
Property & Casualty | Accurate risk assessment, fraud detection |
Life & Health | Personalized coverage, health risk identification |
Commercial | Streamlined underwriting, industry-specific insights |
By leveraging RAG in insurance underwriting, insurers can enhance risk assessment accuracy, streamline processes, mitigate bias, and make data-driven decisions across various insurance domains. This leads to improved underwriting efficiency, reduced losses, and better customer experiences.
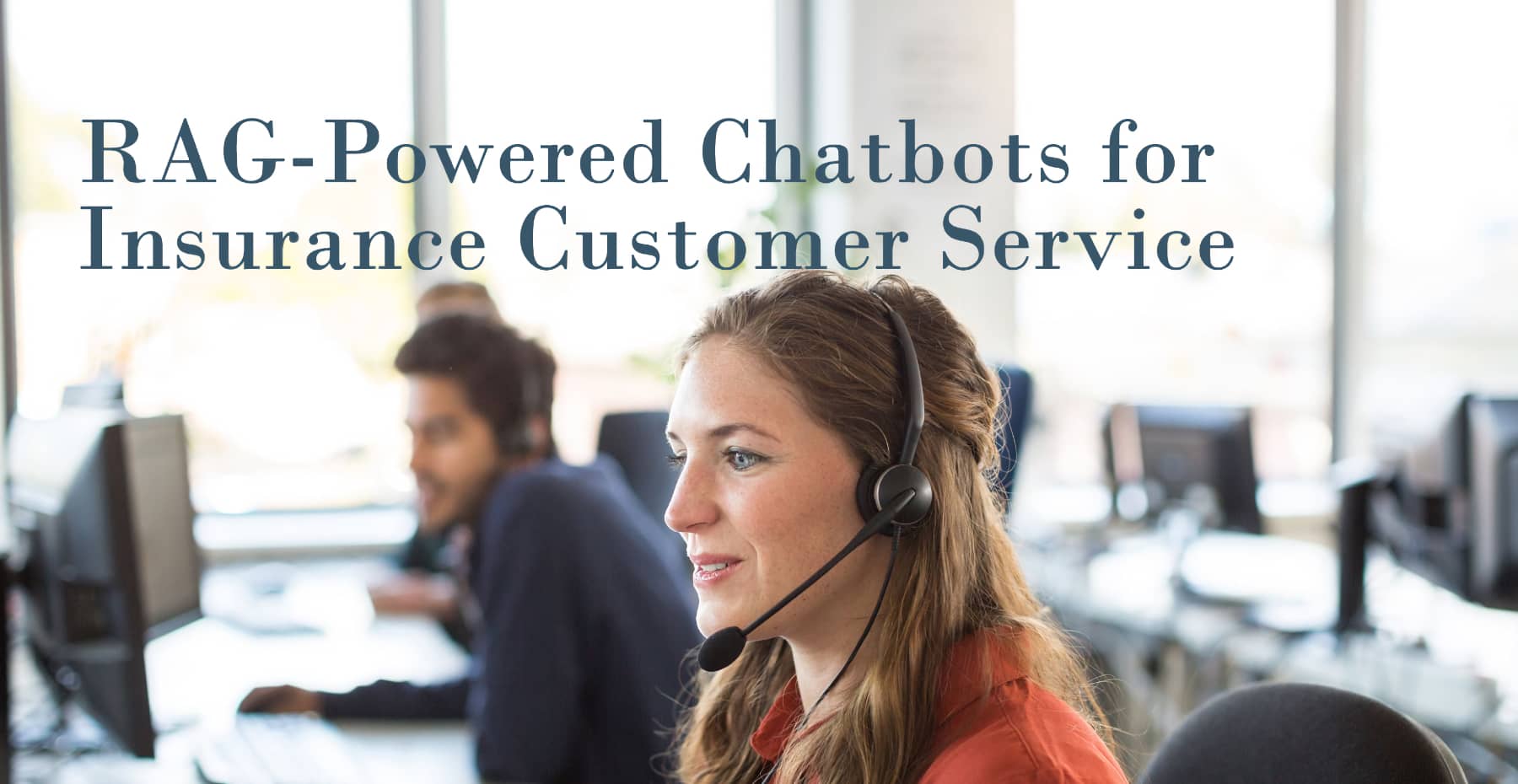
RAG-Powered Chatbots for Insurance Customer Service
Customer service is a critical aspect of the insurance industry, and RAG is transforming the way insurers interact with their customers. By integrating RAG with chatbots and virtual assistants, insurance companies can provide personalized, accurate, and efficient support to policyholders.
Handling Complex Insurance Queries
Insurance policies and procedures can be complex and difficult for customers to understand. RAG-powered chatbots are designed to handle the intricacies of insurance queries by leveraging advanced natural language processing (NLP) and machine learning techniques.
These chatbots can understand the context and nuances of customer inquiries, even when they are expressed in natural language. By retrieving relevant information from policy documents, FAQs, and knowledge bases, RAG enables chatbots to provide accurate and contextually relevant responses to customer questions.
RAG-powered chatbots can handle a wide range of insurance-related queries, such as:
Policy coverage and exclusions
Claims processes and status updates
Premium calculations and payments
Insurance terminology and definitions
By providing instant and accurate answers, RAG-powered chatbots reduce the need for customers to navigate through complex documents or wait for human assistance.
Improving Customer Experience
RAG-powered chatbots significantly enhance the customer experience in the insurance industry. With 24/7 availability, customers can access support and information at their convenience, without being limited by business hours or call center capacity.
Moreover, RAG enables chatbots to provide personalized interactions tailored to each customer's specific needs and circumstances. By retrieving customer data, policy details, and interaction history, chatbots can offer customized recommendations, suggest relevant products or services, and proactively address potential concerns.
RAG-powered chatbots can also handle multi-turn conversations, allowing customers to have more natural and engaging interactions. Chatbots can ask clarifying questions, provide step-by-step guidance, and offer personalized insights based on the customer's responses.
By delivering efficient, personalized, and accessible support, RAG-powered chatbots improve customer satisfaction, build trust, and foster long-term relationships between insurers and policyholders.
Integrating with Existing Systems and Workflows
RAG-powered chatbots can be seamlessly integrated into an insurer's existing systems and workflows, enhancing the capabilities of human agents and streamlining customer service processes.
Chatbots can handle routine inquiries and provide instant support, freeing up human agents to focus on more complex and high-value tasks. When necessary, chatbots can escalate conversations to human agents, providing them with relevant context and customer information for a smooth handover.
RAG enables chatbots to access and retrieve data from various internal systems, such as policy management platforms, claims databases, and customer relationship management (CRM) systems. This integration ensures that chatbots have access to the most up-to-date and accurate information, enabling them to provide comprehensive and reliable support.
Furthermore, RAG-powered chatbots can be integrated with other communication channels, such as websites, mobile apps, and social media platforms. This omnichannel approach allows customers to interact with insurers through their preferred channels, providing a consistent and seamless experience across different touchpoints.
By integrating RAG-powered chatbots into existing systems and workflows, insurers can enhance operational efficiency, reduce response times, and improve overall customer service quality.
Benefits of RAG-Powered Chatbots | Description |
24/7 Availability | Provide support and information around the clock |
Personalized Interactions | Tailor responses based on customer data and history |
Multi-turn Conversations | Handle natural and engaging dialogues |
Integration with Existing Systems | Access data from various internal systems |
Omnichannel Support | Interact with customers across different channels |
RAG-powered chatbots are revolutionizing the way insurers deliver customer service, offering a more efficient, personalized, and customer-centric approach. By leveraging the power of RAG, insurers can enhance customer satisfaction, build stronger relationships, and gain a competitive edge in the market.
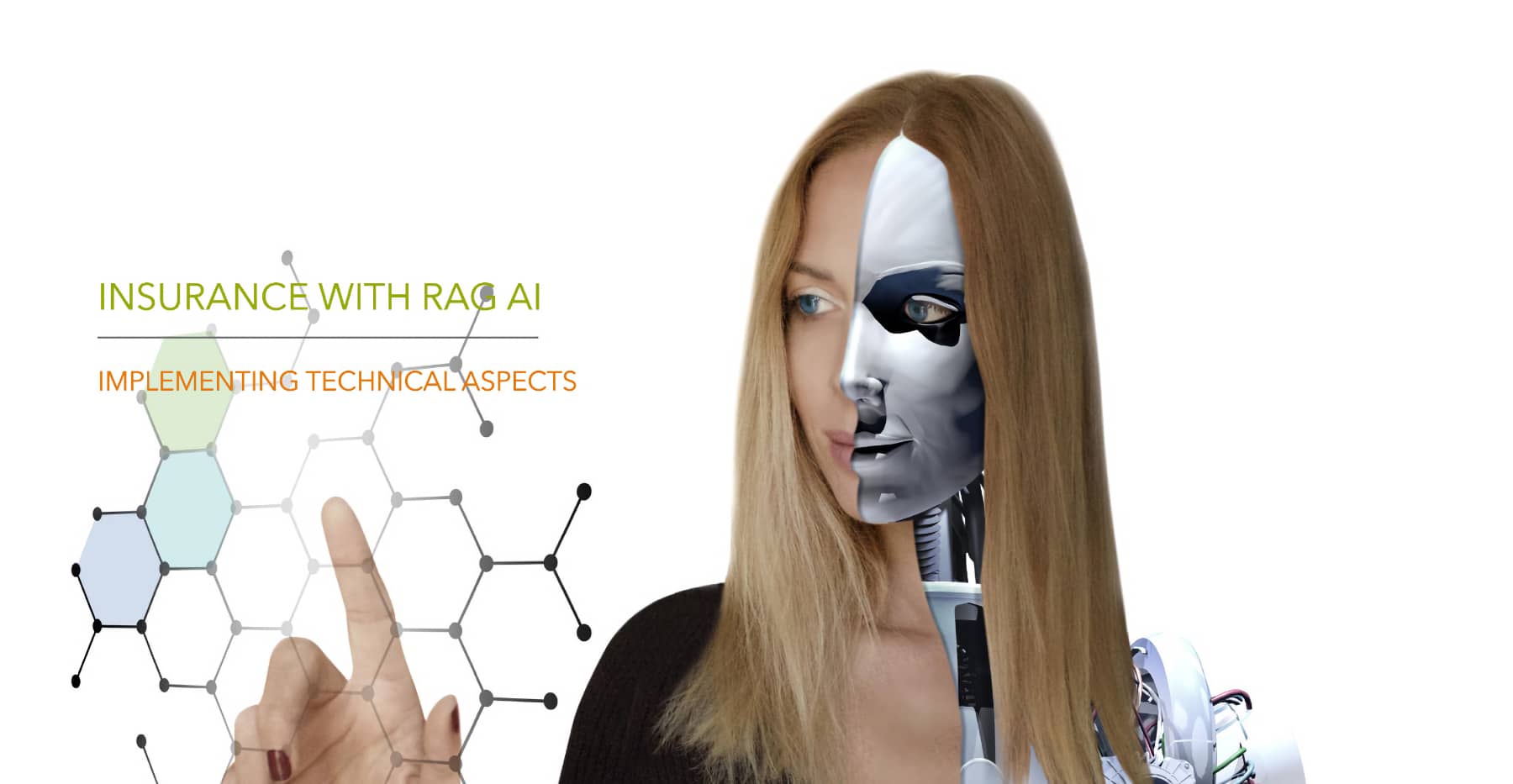
Technical Aspects of RAG Implementation in Insurance
Implementing RAG in the insurance industry involves several technical considerations and processes. Let's explore the key aspects of RAG implementation and how they enable insurers to leverage the full potential of this technology.
Encoding and Vectorizing Insurance Documents
One of the fundamental steps in implementing RAG is transforming unstructured insurance data, such as policy documents, claim reports, and customer communications, into a format that can be efficiently processed by AI models. This process involves encoding and vectorizing the textual data.
Encoding refers to the process of converting text into numerical representations that capture the semantic meaning and relationships between words and phrases. Techniques like tokenization and embedding are used to break down the text into smaller units and assign them unique numerical values.
Vectorization takes the encoded text and represents it as high-dimensional vectors in a mathematical space. Each vector captures the semantic information and contextual relationships of the text, allowing for efficient similarity comparisons and retrieval.
By encoding and vectorizing insurance documents, RAG enables insurers to leverage the vast amount of unstructured data available within their organizations. This process unlocks the potential for AI models to understand and analyze complex insurance-related information, leading to more accurate insights and decision-making.
Leveraging Vector Databases and Knowledge Graphs
RAG relies on advanced data storage and retrieval techniques to efficiently access and utilize the encoded insurance data. Two key components in this regard are vector databases and knowledge graphs.
Vector databases, such as ChromaDB and PineCone, are specialized databases designed to store and retrieve high-dimensional vectors. These databases enable fast and efficient similarity search, allowing RAG models to quickly find relevant information based on the semantic similarity between query vectors and stored vectors.
Knowledge graphs, on the other hand, provide a structured representation of insurance-related entities, their attributes, and the relationships between them. Knowledge graphs capture the domain-specific knowledge and ontologies relevant to the insurance industry, such as policy types, coverage terms, and claim processes.
By combining vector databases and knowledge graphs, RAG can perform complex queries and retrieve the most relevant information from vast amounts of insurance data. This enables insurers to provide accurate and contextually relevant responses to customer inquiries, support underwriting decisions, and generate personalized recommendations.
Cloud vs. On-Premises Deployment Considerations
When implementing RAG in the insurance industry, organizations must consider the deployment options available: cloud-based or on-premises. Each approach has its own advantages and considerations.
Cloud-based deployment offers several benefits, including:
Cost-efficiency: Cloud providers offer scalable and flexible pricing models, allowing insurers to pay for the resources they consume without significant upfront investments.
Scalability: Cloud platforms can easily scale up or down based on the demand, ensuring that RAG models can handle varying workloads and growing data volumes.
Ease of integration: Cloud-based RAG solutions often provide APIs and connectors for seamless integration with existing insurance systems and workflows.
However, cloud-based deployment also raises concerns about data privacy and security. Insurers must ensure that sensitive customer data is protected and complies with regulatory requirements when stored and processed in the cloud.
On-premises deployment, on the other hand, gives insurers full control over their data and infrastructure. It allows for greater customization and integration with existing on-premises systems. On-premises deployment is suitable for organizations with strict data privacy and security requirements or those operating in regulated environments.
The choice between cloud-based and on-premises deployment depends on various factors, including an insurer's technology landscape, regulatory compliance needs, budget considerations, and scalability requirements. Many insurers opt for a hybrid approach, combining the benefits of both deployment models to strike a balance between flexibility and control.
Deployment Model | Advantages | Considerations |
Cloud-based | Cost-efficiency, scalability, ease of integration | Data privacy and security concerns |
On-premises | Full control, customization, integration with existing systems | Higher upfront costs, limited scalability |
Regardless of the deployment model chosen, implementing RAG in the insurance industry requires careful planning, data governance, and collaboration between business and IT teams. Insurers must ensure that the RAG implementation aligns with their business objectives, regulatory requirements, and customer expectations.
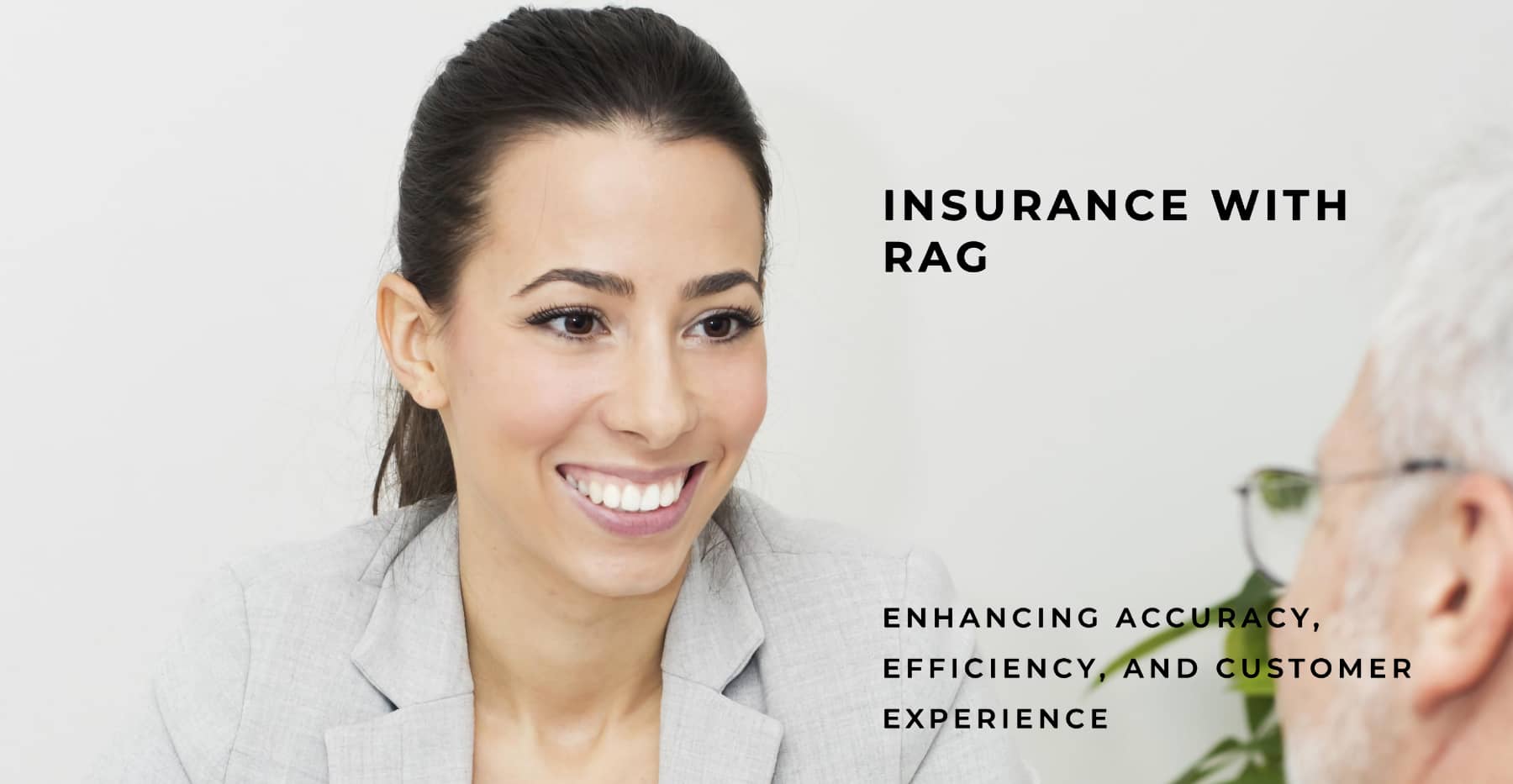
Challenges and Considerations
While RAG offers tremendous potential for transforming the insurance industry, it also presents certain challenges and considerations that insurers must address to ensure successful implementation and adoption.
Ensuring Data Quality and Completeness
One of the primary challenges in implementing RAG is ensuring the quality and completeness of the data used to train and operate the models. Insurance data often comes from various sources and may be inconsistent, incomplete, or outdated. Insurers must invest in data cleansing, standardization, and integration processes to ensure that the data fed into RAG models is accurate, reliable, and up to date.
Moreover, insurers must establish robust data governance frameworks to maintain the integrity and security of sensitive customer information. This includes implementing strict access controls, data encryption, and compliance with privacy regulations such as GDPR or HIPAA.
Addressing Explainability and Transparency
As RAG models become more complex and sophisticated, ensuring explainability and transparency becomes crucial. Insurers must be able to understand and interpret the reasoning behind the decisions and recommendations made by RAG models. This is particularly important in the insurance industry, where decisions can have significant financial and legal implications.
Insurers should prioritize the development of explainable AI techniques that provide clear insights into the factors influencing RAG model outputs. This transparency helps build trust with customers, regulators, and other stakeholders, demonstrating that the models are fair, unbiased, and aligned with ethical principles.
Navigating Regulatory Compliance
The insurance industry is heavily regulated, with strict requirements around data privacy, fairness, and consumer protection. Implementing RAG in insurance must be done in compliance with these regulations to avoid legal and reputational risks.
Insurers must work closely with legal and compliance teams to ensure that RAG models adhere to relevant laws and guidelines. This may involve conducting regular audits, implementing governance frameworks, and maintaining detailed documentation of the data and algorithms used in RAG models.
Balancing AI-Driven Insights with Human Judgment
While RAG offers powerful capabilities for automating tasks and generating insights, it is essential to strike the right balance between AI-driven decisions and human judgment. Insurance professionals, such as underwriters and claims adjusters, bring valuable domain expertise and intuition that cannot be fully replicated by machines.
Insurers should view RAG as a tool to augment and support human decision-making rather than replace it entirely. By combining the strengths of AI and human expertise, insurers can achieve optimal results and ensure that decisions are made with the necessary context and nuance.
Real-World Case Studies
To illustrate the tangible benefits of RAG in the insurance industry, let's explore a few real-world case studies where insurers have successfully implemented this technology.
Case Study 1: Improving Claims Processing Efficiency
A leading property and casualty insurer implemented RAG to streamline its claims processing workflow. By leveraging RAG-powered chatbots and intelligent document processing, the insurer was able to automatically extract relevant information from claim forms, policy documents, and supporting evidence.
The RAG system analyzed the extracted data, identified patterns, and provided claims adjusters with prioritized tasks and recommendations. This automation reduced manual effort, accelerated the claims process, and improved the accuracy of settlements. As a result, the insurer achieved a 30% reduction in claims processing time and a 25% increase in customer satisfaction.
Case Study 2: Enhancing Risk Assessment in Life Insurance
A life insurance company deployed RAG to enhance its risk assessment capabilities. By integrating RAG with its underwriting systems, the insurer was able to retrieve and analyze vast amounts of structured and unstructured data, including medical records, lifestyle factors, and mortality tables.
The RAG models identified complex patterns and correlations, enabling underwriters to make more accurate risk assessments and pricing decisions. The system also provided personalized recommendations for additional medical tests or lifestyle modifications to mitigate potential risks.
Through the implementation of RAG, the life insurer achieved a 15% improvement in risk assessment accuracy, leading to better-informed underwriting decisions and more competitive pricing for customers.
Future Outlook
As RAG continues to evolve and mature, the insurance industry can expect to see even more transformative applications and benefits. Some of the exciting prospects on the horizon include:
Personalized Insurance Products: RAG can enable insurers to offer highly personalized insurance products tailored to individual customer needs and risk profiles. By leveraging real-time data and advanced analytics, insurers can dynamically adjust coverage options, pricing, and rewards based on customer behavior and preferences.
Proactive Risk Mitigation: RAG can help insurers proactively identify and mitigate risks by analyzing vast amounts of data from various sources, such as IoT devices, social media, and public records. By detecting early warning signs and providing timely recommendations, insurers can prevent losses and improve risk management strategies.
Fraud Detection and Prevention: RAG can bolster an insurer's ability to detect and prevent fraudulent activities. By analyzing patterns and anomalies in claims data, customer behavior, and network relationships, RAG models can identify potential fraud cases with high accuracy, saving insurers significant losses and protecting honest policyholders.
Seamless Customer Experience: RAG can enable insurers to deliver a seamless and personalized customer experience across all touchpoints. By integrating RAG-powered chatbots, virtual assistants, and recommendation engines, insurers can provide instant support, relevant information, and tailored offerings, enhancing customer satisfaction and loyalty.
As the insurance industry continues to embrace RAG and other AI technologies, the future looks promising. Insurers that successfully harness the power of RAG will be well-positioned to drive innovation, improve operational efficiency, and deliver superior customer value in an increasingly competitive market.
Conclusion
Retrieval-Augmented Generation (RAG) is revolutionizing the insurance industry by enabling insurers to unlock the full potential of their data and deliver more accurate, efficient, and customer-centric services. From enhancing risk assessment in underwriting to streamlining claims processing and enabling personalized customer experiences, RAG offers a wide range of benefits across various insurance domains.
However, implementing RAG in insurance also comes with challenges and considerations. Insurers must prioritize data quality, explainability, regulatory compliance, and the balance between AI-driven insights and human judgment. By addressing these challenges head-on and adopting best practices, insurers can successfully harness the transformative power of RAG.
Real-world case studies demonstrate the tangible impact of RAG in improving operational efficiency, risk assessment accuracy, and customer satisfaction. As the technology continues to advance, the future of RAG in insurance looks bright, with exciting prospects for personalized products, proactive risk mitigation, fraud detection, and seamless customer experiences.
Insurers that embrace RAG and invest in its responsible implementation will be well-positioned to thrive in the rapidly evolving insurance landscape. By leveraging the power of AI and data-driven insights, insurers can drive innovation, gain a competitive edge, and deliver unparalleled value to their customers.
So, if you're an insurer looking to stay ahead of the curve, now is the time to explore the transformative potential of Retrieval-Augmented Generation. Embrace the future of insurance and unlock the full power of your data with RAG.